AFT*: Integrating Active Learning and Transfer Learning to Reduce Annotation Efforts.
arXiv: Learning(2018)
摘要
The splendid success of convolutional neural networks (CNNs) in computer vision is largely attributed to the availability of large annotated datasets, such as ImageNet and Places. However, in biomedical imaging, it is very challenging to create such large annotated datasets, as annotating biomedical images is not only tedious, laborious, and time consuming, but also demanding of costly, specialty-oriented skills, which are not easily accessible. To dramatically reduce annotation cost, this paper presents a novel method to naturally integrate active learning and transfer learning (fine-tuning) into a single framework, called AFT*, which starts directly with a pre-trained CNN to seek worthy samples for annotation and gradually enhance the (fine-tuned) CNN via continuous fine-tuning. We have evaluated our method in three distinct biomedical imaging applications, demonstrating that it can cut the annotation cost by at least half, in comparison with the state-of-the-art method. This performance is attributed to the several advantages derived from the advanced active, continuous learning capability of our method. Although AFT* was initially conceived in the context of computer-aided diagnosis in biomedical imaging, it is generic and applicable to many tasks in computer vision and image analysis; we illustrate the key ideas behind AFT* with the Places database for scene interpretation in natural images.
更多查看译文
AI 理解论文
溯源树
样例
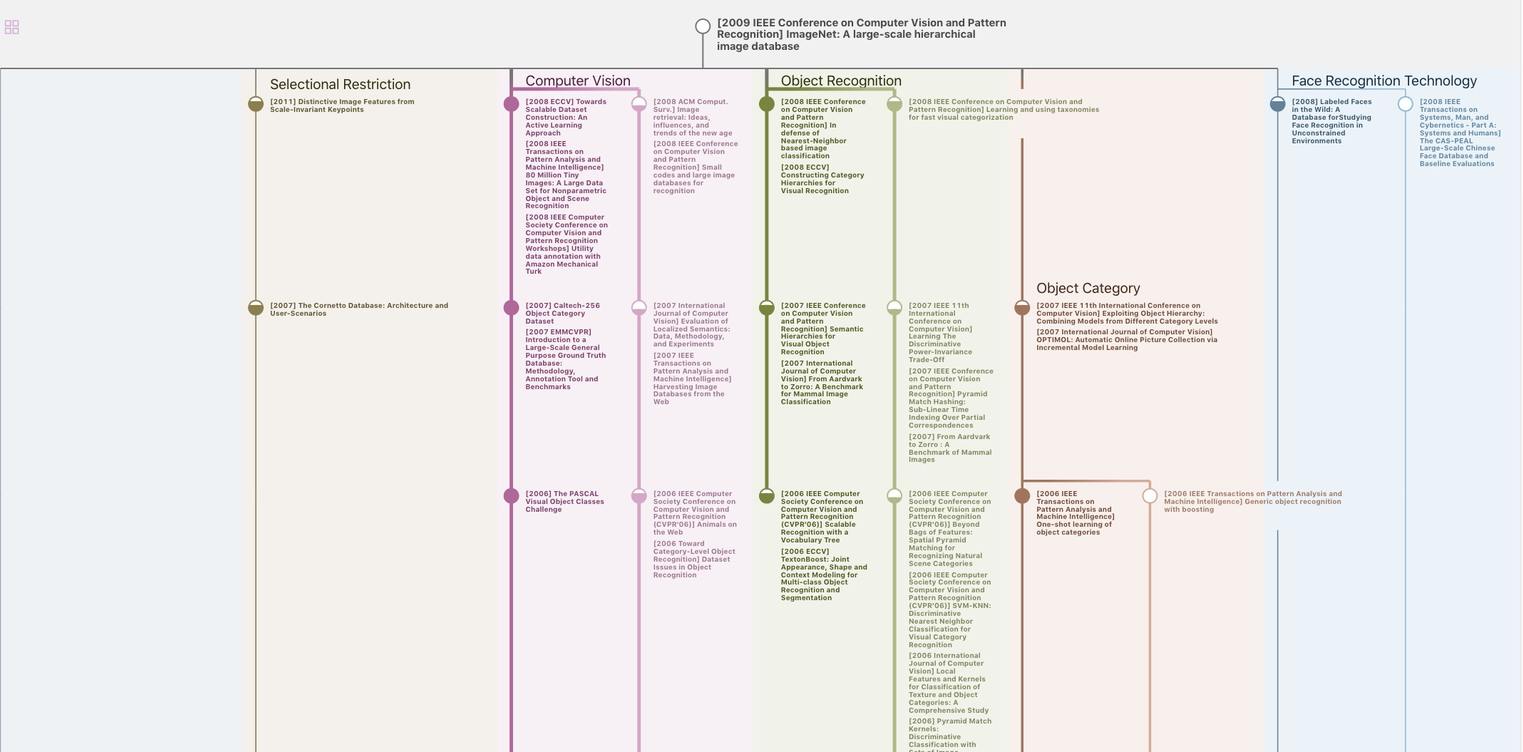
生成溯源树,研究论文发展脉络
Chat Paper
正在生成论文摘要