Convergence Analysis of Belief Propagation on Gaussian Graphical Models.
arXiv: Information Theory(2018)
摘要
Gaussian belief propagation (GBP) is a recursive computation method that is widely used in inference for computing marginal distributions efficiently. Depending on how the factorization of the underlying joint Gaussian distribution is performed, GBP may exhibit different convergence properties as different factorizations may lead to fundamentally different recursive update structures. In this paper, we study the convergence of GBP derived from the factorization based on the distributed linear Gaussian model. The motivation is twofold. From the factorization viewpoint, i.e., by specifically employing a factorization based on the linear Gaussian model, in some cases, we are able to bypass difficulties that exist in other convergence analysis methods that use a different (Gaussian Markov random field) factorization. From the distributed inference viewpoint, the linear Gaussian model readily conforms to the physical network topology arising in large-scale networks, and, is practically useful. For the distributed linear Gaussian model, under mild assumptions, we show analytically three main results: the GBP message inverse variance converges exponentially fast to a unique positive limit for arbitrary nonnegative initialization; we provide a necessary and sufficient convergence condition for the belief mean to converge to the optimal value; and, when the underlying factor graph is given by the union of a forest and a single loop, we show that GBP always converges.
更多查看译文
AI 理解论文
溯源树
样例
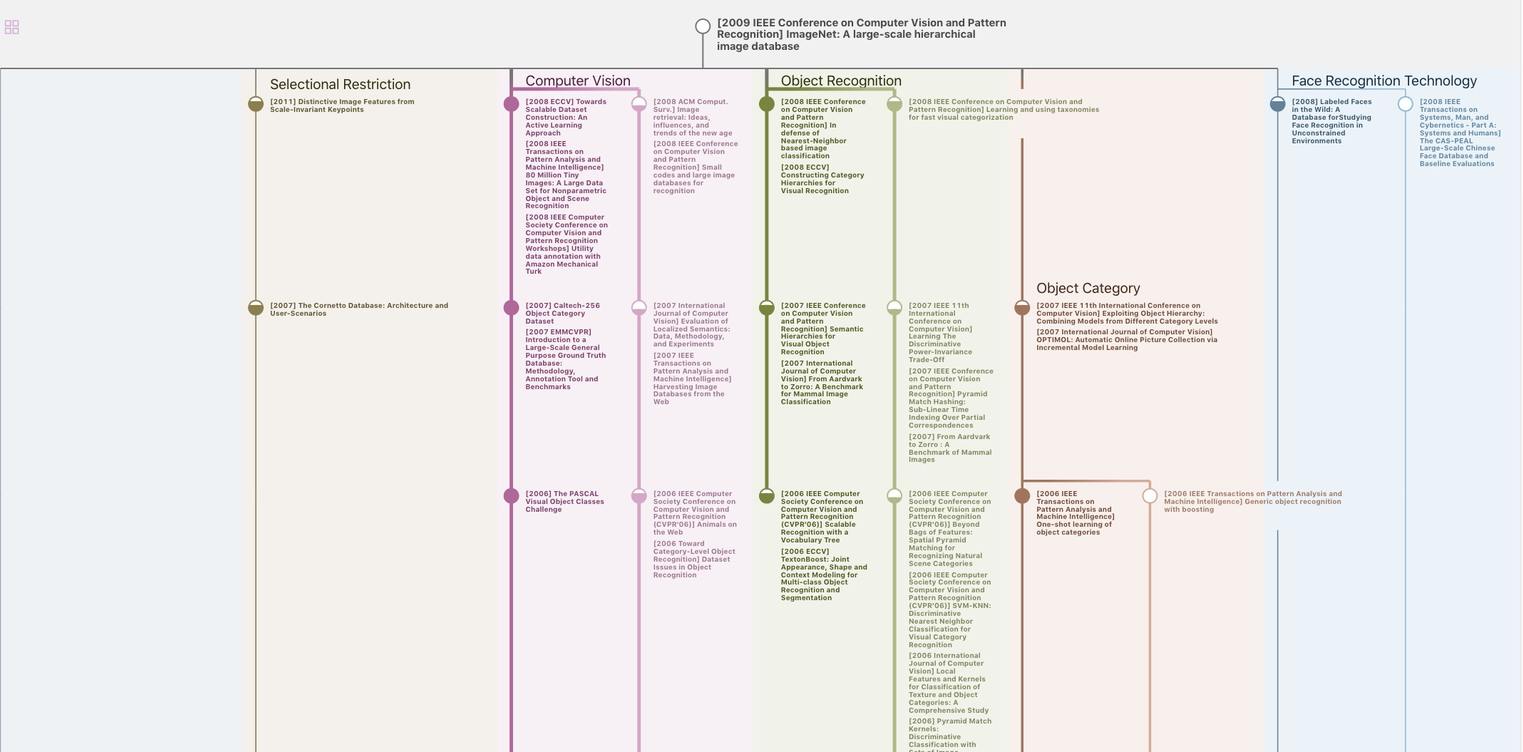
生成溯源树,研究论文发展脉络
Chat Paper
正在生成论文摘要