Black-Box Reductions for Parameter-free Online Learning in Banach Spaces
arXiv (Cornell University)(2018)
摘要
We introduce several new black-box reductions that significantly improve the design of adaptive and parameter-free online learning algorithms by simplifying analysis, improving regret guarantees, and sometimes even improving runtime. We reduce parameter-free online learning to online exp-concave optimization, we reduce optimization in a Banach space to one-dimensional optimization, and we reduce optimization over a constrained domain to unconstrained optimization. All of our reductions run as fast as online gradient descent. We use our new techniques to improve upon the previously best regret bounds for parameter-free learning, and do so for arbitrary norms.
更多查看译文
关键词
learning,banach spaces,reductions,black-box,parameter-free
AI 理解论文
溯源树
样例
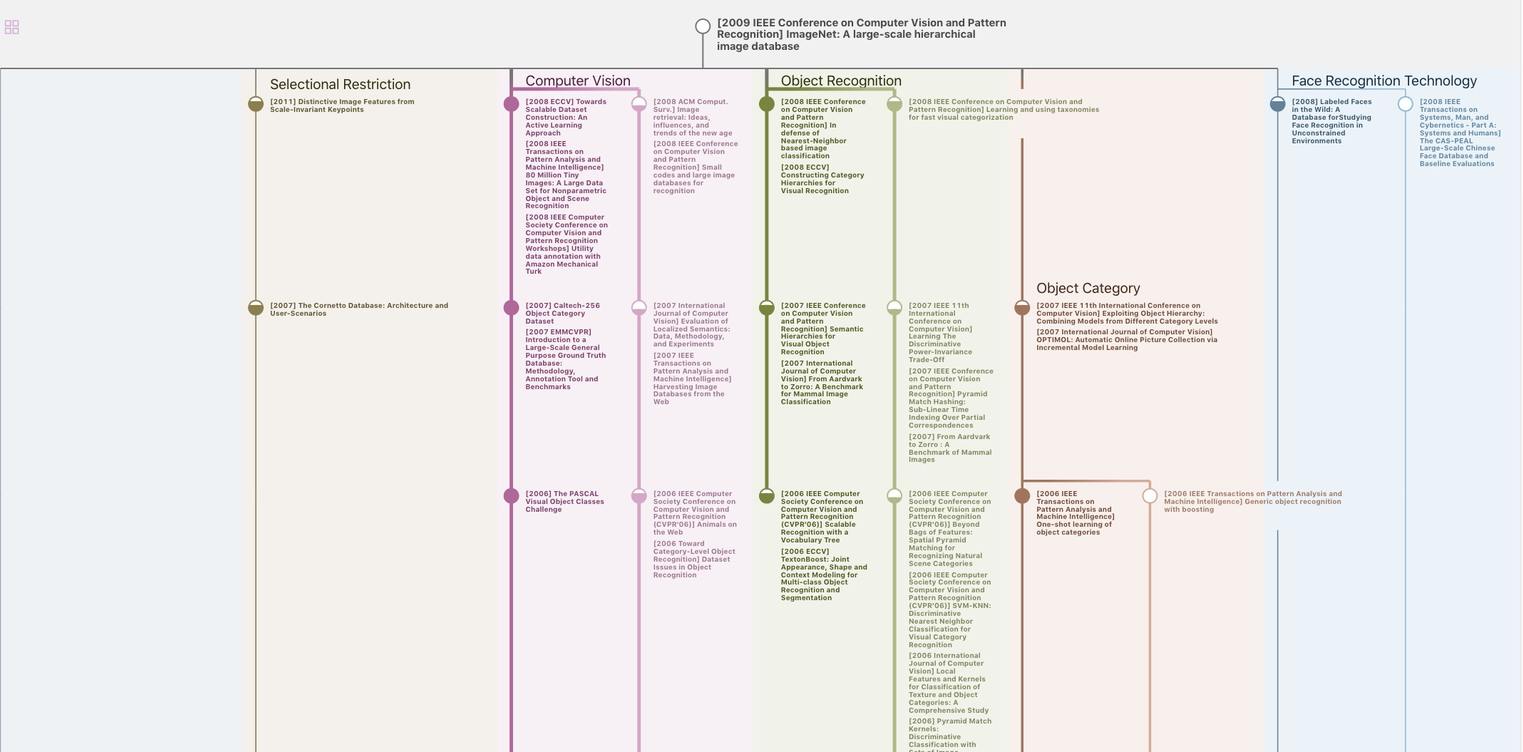
生成溯源树,研究论文发展脉络
Chat Paper
正在生成论文摘要