Low-Rank Methods in Event Detection.
arXiv: Data Structures and Algorithms(2018)
摘要
We present low-rank methods for event detection. We assume that normal observation come from a low-rank subspace, prior to being corrupted by a uniformly distributed noise. Correspondingly, we aim at recovering a representation of the subspace, and perform event detection by running point-to-subspace distance query $ell^infty$, for each incoming observation. In particular, we use a variant of matrix completion under interval uncertainty on a suitable flattening $M in R^{m times n}$ of the input data to obtain a low-rank model $M approx L times R$, $L in R^{m times r}$, $R in R^{r times n}$, $r ll m$. On-line, we compute the distance of each incoming $x in R^n$ to the space spanned by $R$. For the distance computation, we present a constant-time algorithm with a one-sided error bounded by a function of the number of coordinates employed.
更多查看译文
关键词
Sensors, Monitoring, Event detection, Internet of Things, Noise measurement, Time series analysis, Sparse matrices, Multidimensional signal processing, monitoring, matrix completion, point-to-subspace proximity, probably approximately correct learning
AI 理解论文
溯源树
样例
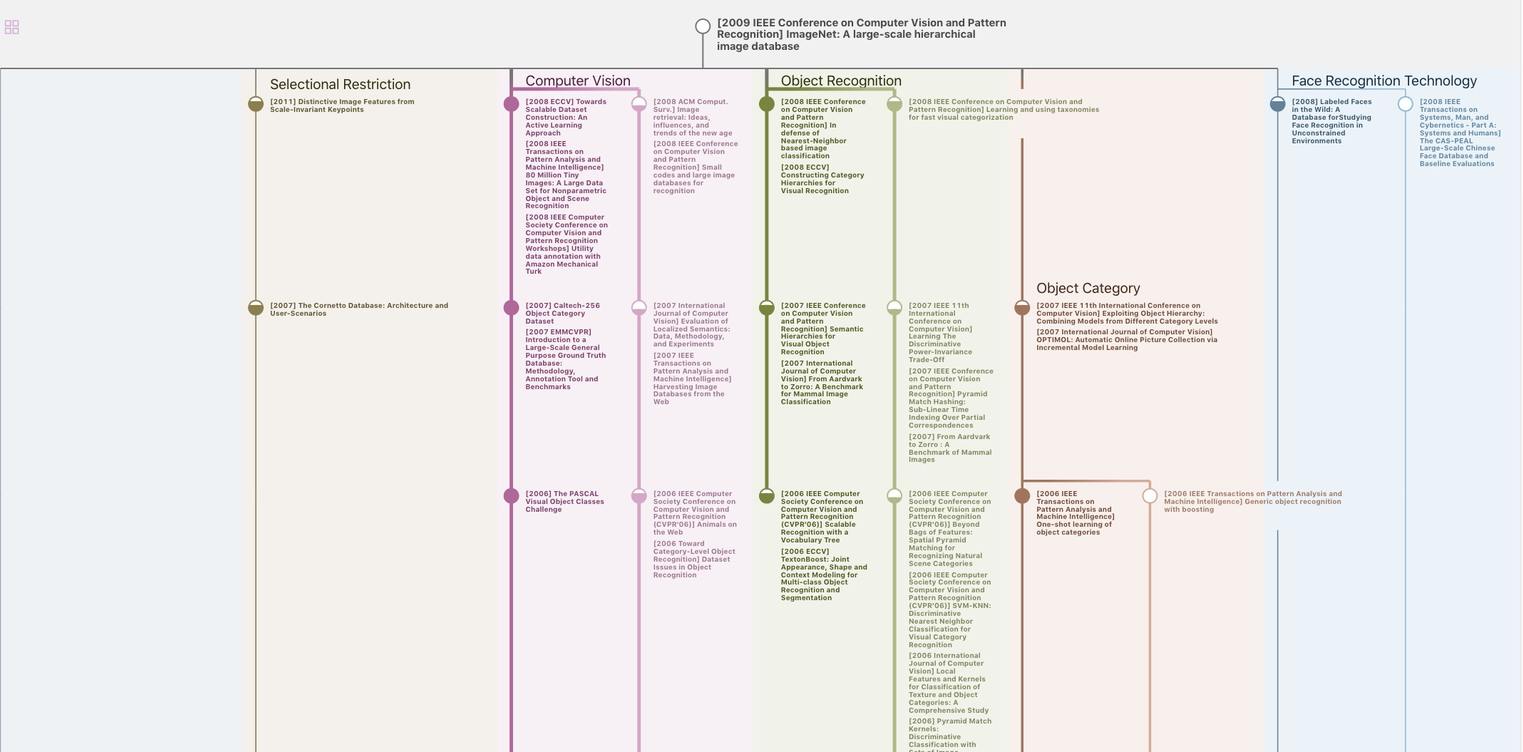
生成溯源树,研究论文发展脉络
Chat Paper
正在生成论文摘要