Correspondence-free pose estimation for 3D objects from noisy depth data
The Visual Computer(2016)
摘要
Estimating the pose of objects from depth data is a problem of considerable practical importance for many vision applications. This paper presents an approach for accurate and efficient 3D pose estimation from noisy 2.5D depth images obtained from a consumer depth sensor. Initialized with a coarsely accurate pose, the proposed approach applies a hypothesize-and-test scheme that combines stochastic optimization and graphics-based rendering to refine the supplied initial pose, so that it accurately accounts for a sensed depth image. Pose refinement employs particle swarm optimization to minimize an objective function that quantifies the misalignment between the acquired depth image and a rendered one that is synthesized from a hypothesized pose with the aid of an object mesh model. No explicit correspondences between the depth data and the model need to be established, whereas pose hypothesis rendering and objective function evaluation are efficiently performed on the GPU. Extensive experimental results demonstrate the superior performance of the proposed approach compared to the ICP algorithm, which is typically used for pose refinement in depth images. Furthermore, the experiments indicate the graceful degradation of its performance to limited computational resources and its robustness to noisy and reduced polygon count models, attesting its suitability for use with automatically scanned object models and common graphics hardware.
更多查看译文
关键词
3D pose estimation,Object localization,Depth images,RGB-D sensors,Particle swarm optimization,GPU computing
AI 理解论文
溯源树
样例
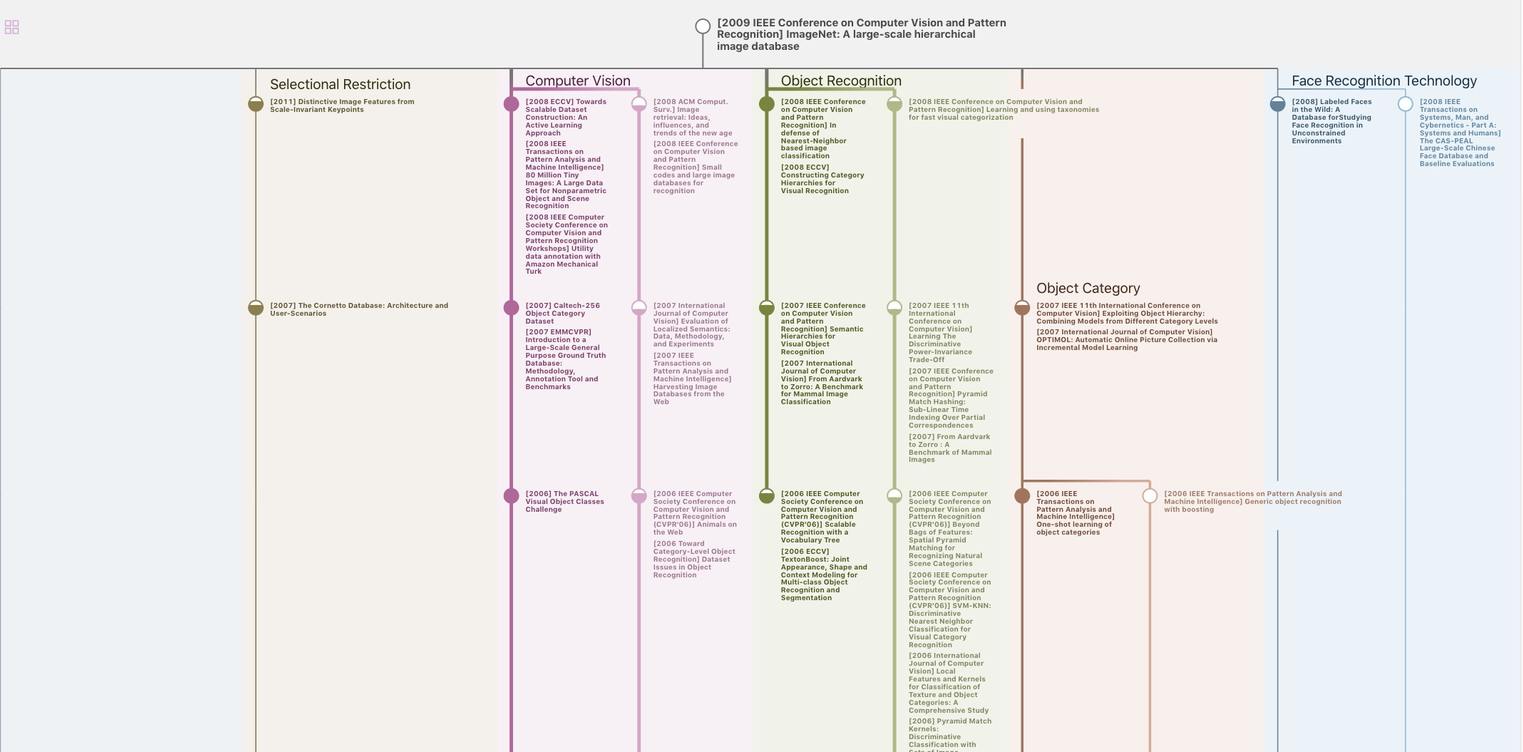
生成溯源树,研究论文发展脉络
Chat Paper
正在生成论文摘要