Optimal-Coherent And Adaptive Software Defined Inference Of Network Traffics (Occasion)
2017 27TH INTERNATIONAL TELECOMMUNICATION NETWORKS AND APPLICATIONS CONFERENCE (ITNAC)(2017)
摘要
In this paper, the OCcASION framework for Traffic Matrix (TM) estimation in Software Defined Networks (SDN) is proposed where, first in the learning phase, the Optimal Observation Matrix (OOM) of SNMP link-loads is estimated. Then in the measurement and inference phase, the OOM is used to coherently find the minimum-norm estimate of the unknown TM. This framework is applied under both non-adaptive and adaptive scenarios. The performance of OCcASION framework is evaluated using synthetic and practical traffic traces with real network topologies. It is shown that, comparing with regular minimum-norm estimation, this framework can significantly improve the accuracy of the TM estimation; for example, on Geant network the estimation error is approximately reduced by 83%.
更多查看译文
关键词
-coherent software defined inference,adaptive software defined inference,network traffics,OCcASION framework,learning phase,OOM,SNMP link-loads,inference phase,unknown TM,adaptive scenarios,synthetic traffic traces,network topologies,minimum-norm estimation,TM estimation,optimal observation matrix,software defined networks,traffic Matrix estimation
AI 理解论文
溯源树
样例
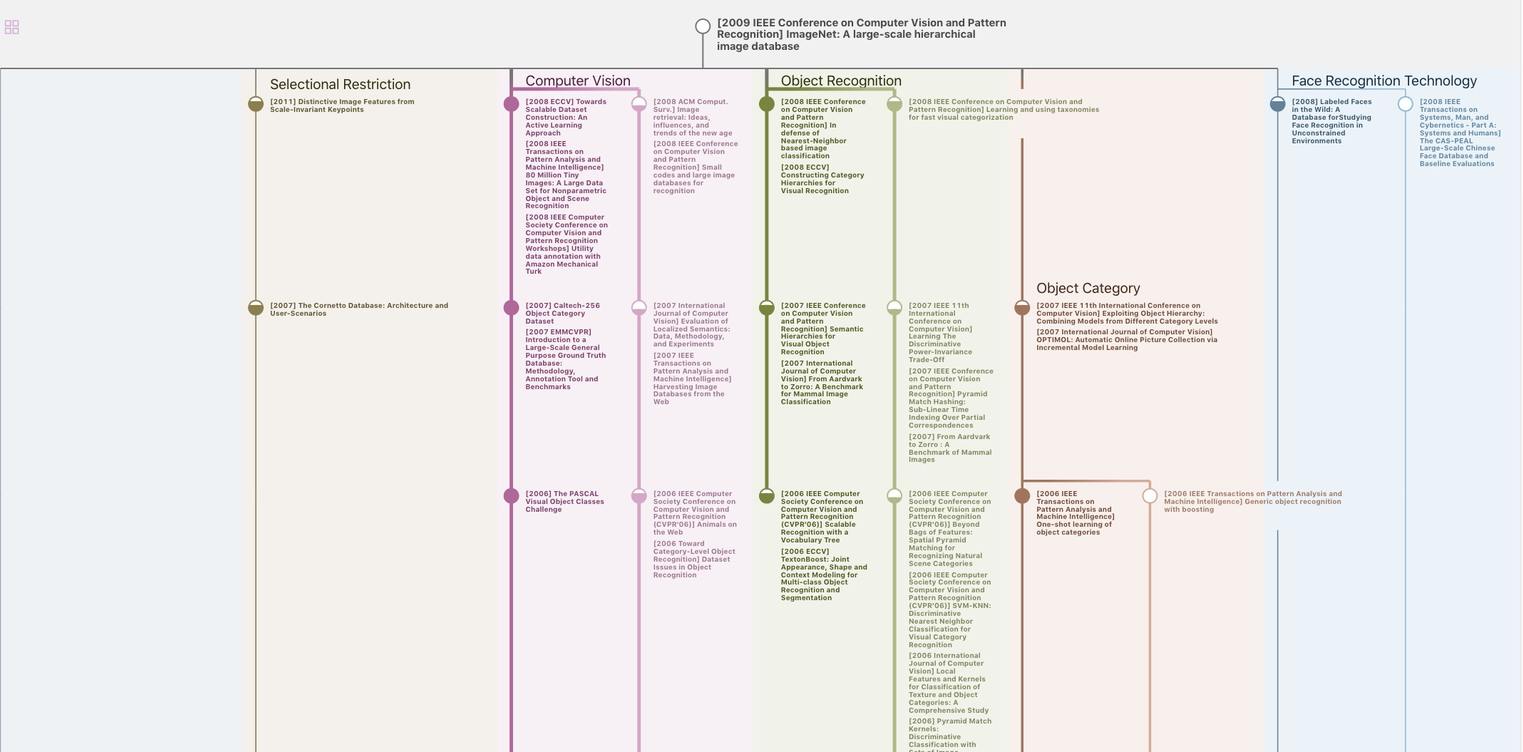
生成溯源树,研究论文发展脉络
Chat Paper
正在生成论文摘要