Supervised Temporal Link Prediction Using Time Series Of Similarity Measures
2017 NINTH INTERNATIONAL CONFERENCE ON UBIQUITOUS AND FUTURE NETWORKS (ICUFN 2017)(2017)
摘要
Online social networks have become more popular in recent years. Many of these networks have very dynamic structures which means that nodes and relations (links) may appear or disappear over time. The dynamicity of the networks provides vital information about the tendency of links to be formed, and, for that reason, should be studied in the link prediction task for better performance. Most of the previous link prediction studies ignore the evolution of the network over time and mainly perform link prediction by statically analyzing the network without considering the temporal behavior of links through different time periods. In this article, we propose a link prediction method based on a supervised learning task for evolving networks which considers the dynamic topology of social networks. Our method first computes different similarity scores at different time periods then builds time series of node similarity scores for each pair of nodes. Then, ARIMA forecasting model is used on these created time series to predict the future similarity scores of the node pairs. These predicted similarity scores are selected as features which are employed by the supervised classifiers to apply the link prediction task. The proposed method is evaluated on different social networks. It is shown that combining time information with supervised classifiers improves the link prediction performance to a large extent.
更多查看译文
关键词
supervised temporal link prediction,time series,similarity measures,online social networks,supervised learning,dynamic topology,ARIMA forecasting model,supervised classifiers
AI 理解论文
溯源树
样例
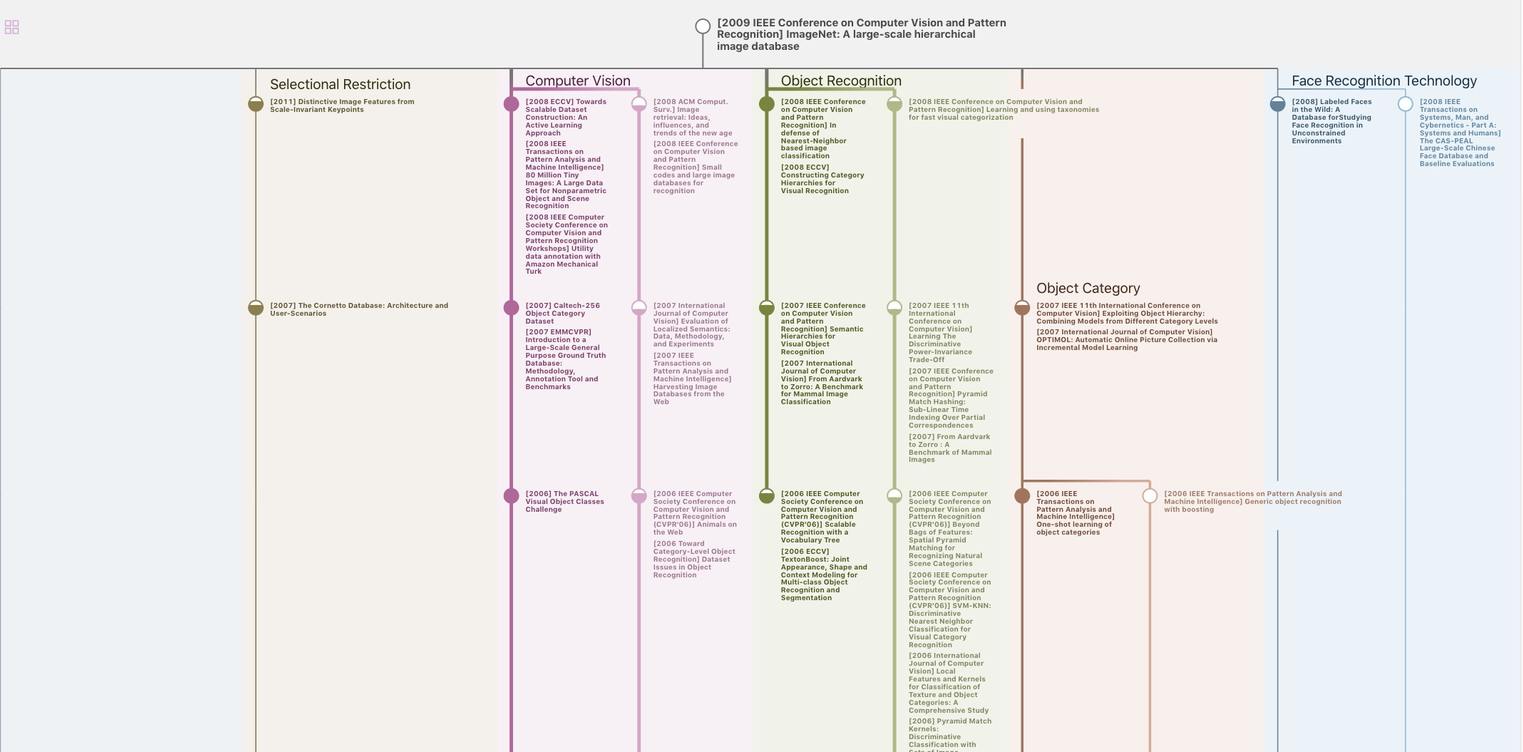
生成溯源树,研究论文发展脉络
Chat Paper
正在生成论文摘要