A Flexible Framework for the Evaluation of Unsupervised Image Annotation.
CIARP(2017)
摘要
Automatic Image Annotation (AIA) consists in assigning keywords to images describing their visual content. A prevalent way to address the AIA task is based on supervised learning. However, the unsupervised approach is a new alternative that makes a lot of sense when there are not manually labeled images to train supervised techniques. AIA methods are typically evaluated using supervised learning performance measures, however applying these kind of measures to unsupervised methods is difficult and unfair. The main restriction has to do with the fact that unsupervised methods use an unrestricted annotation vocabulary while supervised methods use a restricted one. With the aim to alleviate the unfair evaluation, in this paper we propose a flexible evaluation framework that allows us to compare coverage and relevance of the assigned words by unsupervised automatic image annotation (UAIA) methods. We show the robustness of our framework through a set of experiments where we evaluated the output of both, unsupervised and supervised methods.
更多查看译文
关键词
evaluation,image,flexible framework
AI 理解论文
溯源树
样例
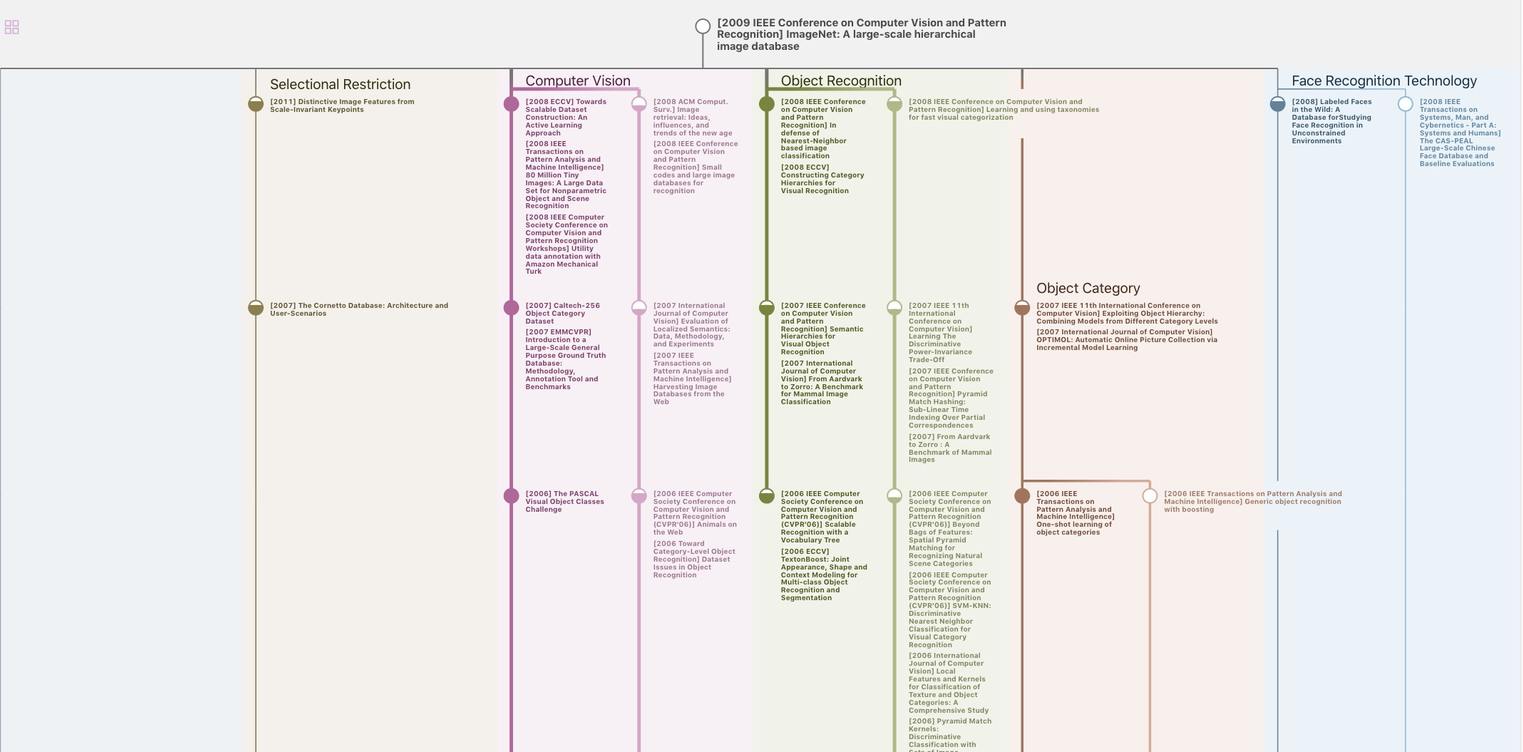
生成溯源树,研究论文发展脉络
Chat Paper
正在生成论文摘要