Approach To Detect Non-Adversarial Overlapping Collusion In Crowdsourcing
2017 IEEE 36TH INTERNATIONAL PERFORMANCE COMPUTING AND COMMUNICATIONS CONFERENCE (IPCCC)(2017)
摘要
Crowdsourcing services have become one of the most common ways organizations can gather ideas for new products and services from large crowds of consumers by offering monetary rewards depending on the tasks. However, this monetary reward has begun to attract malicious crowds of users who wish to complete the task with minimal effort through collaboration. For instance, a task based on reviews of a product can be degraded when malicious users copy each other with minimal edits of the review, giving a misrepresentation of the true quality of the product. More specifically, we investigate the case where different malicious crowd sizes cooperate on different tasks, known as overlapping groups. Such sophisticated and hard to detect malicious crowds provide unfair evaluations and misleading results to the crowdsourcers. To overcome this type of attack, we propose two methods to point out such groups with high accuracy. The first method detects similar reviews by including a new proposed similarity between review texts and show the results outperform the vectorial similarity measures used in prior works. The second method is based on community detection on networks and exploits the semantic similarity of the reviews. The experiments were conducted on reviews from Ott dataset on Amazon Mechanical Turk.
更多查看译文
关键词
Crowdsourcing, security, and colluding attackers
AI 理解论文
溯源树
样例
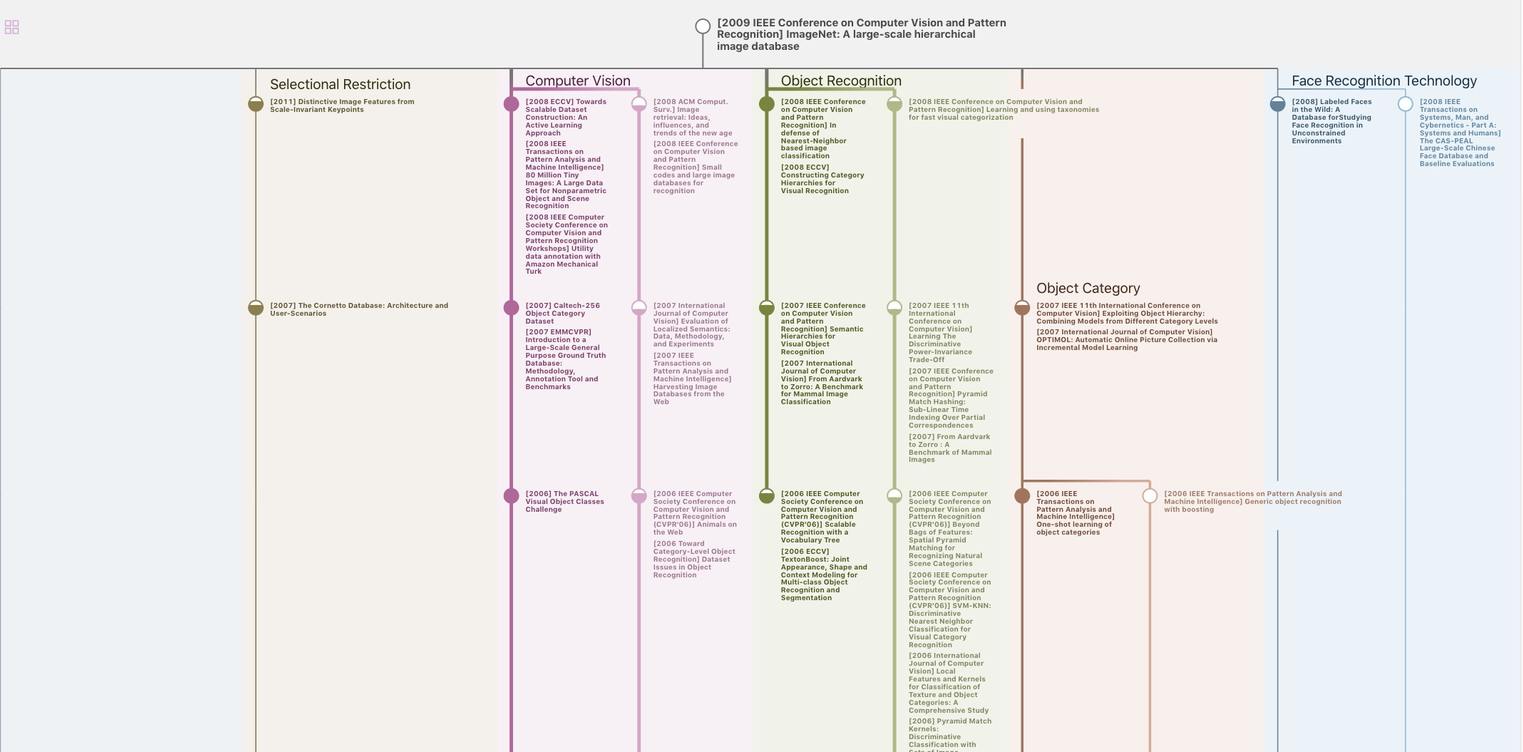
生成溯源树,研究论文发展脉络
Chat Paper
正在生成论文摘要