Characterizing Mention Mismatching Problems For Improving Recognition Results
19TH INTERNATIONAL CONFERENCE ON INFORMATION INTEGRATION AND WEB-BASED APPLICATIONS & SERVICES (IIWAS2017)(2017)
摘要
Mentions to real world things which are recognized by software tools in text often mismatch the ground truth. This paper proposes a formal classification of mention mismatching problems, including partial matching. Then, it depicts evidence that some longer mentions are associated with higher precision and more specific things than shorter mentions that overlap them. Based on this, some algorithms are proposed to automatically improve mentions by increasing their sizes whenever and as much as possible. Experimental results applying a variety of state-of-the-art annotation tools against several datasets made from real world texts show that over-segmentation (returned mention contained in the corresponding one of the ground truth) is the most prevalent partial matching problem among those of the proposed classification. In addition, some of the proposed algorithms for mention enhancing were able to correct most over-segmented mentions returned by tools used in the experiments with prominent benchmarks, leading to gains in precision and recall.
更多查看译文
关键词
Text annotation, semantic annotation, NER, mention mismatch, segmented mentions
AI 理解论文
溯源树
样例
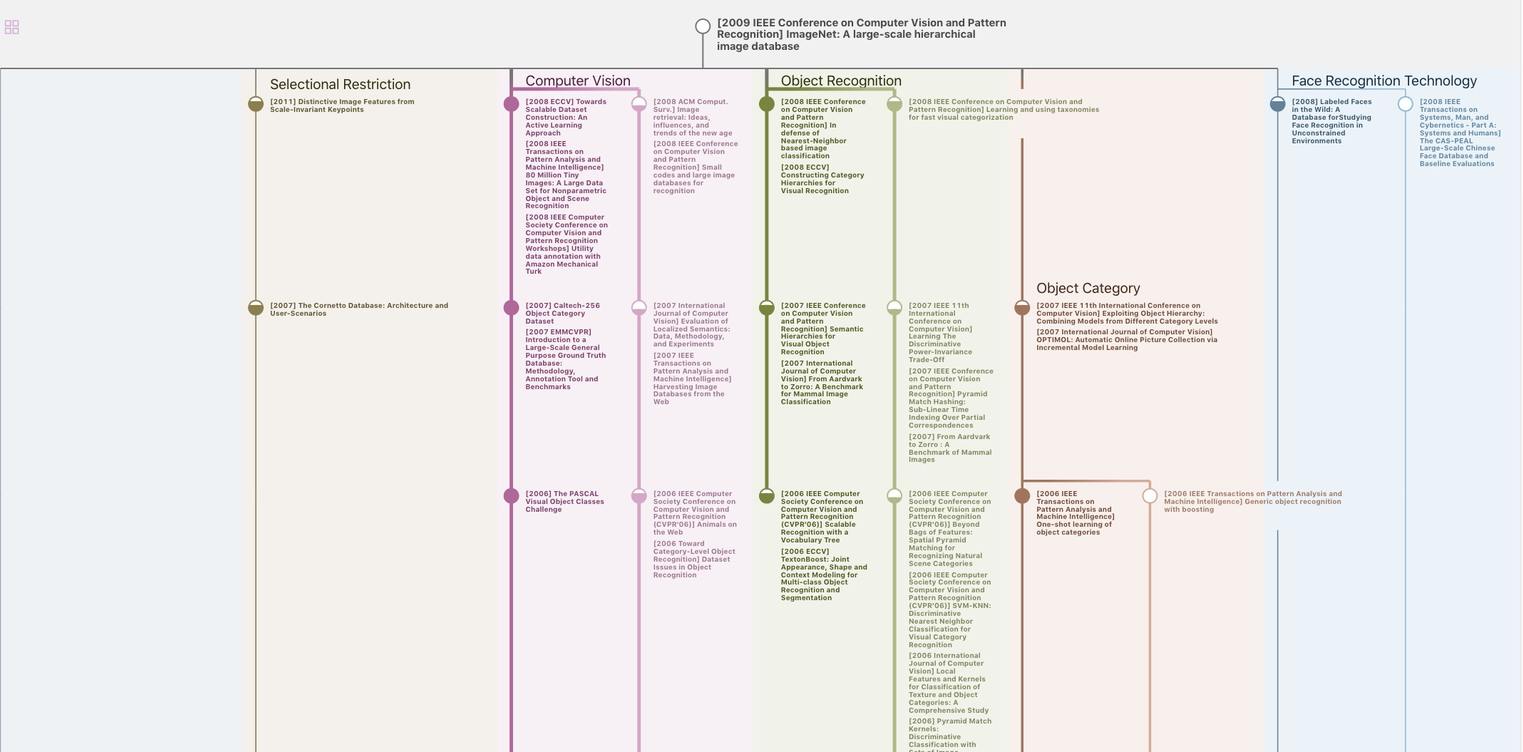
生成溯源树,研究论文发展脉络
Chat Paper
正在生成论文摘要