Augmenting Practical Cross-Layer Mac Schedulers Via Offline Reinforcement Learning
2017 IEEE 28TH ANNUAL INTERNATIONAL SYMPOSIUM ON PERSONAL, INDOOR, AND MOBILE RADIO COMMUNICATIONS (PIMRC)(2017)
摘要
An automated offline design process for optimized cross-layer schedulers can produce augmented scheduling algorithms tailored to a target deployment scenario. We discuss the application of ODDS, a reinforcement learning technique we introduced, for augmenting LTE MAC scheduler algorithms of practical significance. ODDS observes the correlation between the value of a utility function and the cross-layer state parameters seen by an instrumented baseline scheduler, determining the best actions via an offline Monte Carlo exploration of the problem space. The result of the ODDS process is a compact definition of a scheduling policy that has been optimized for a target scenario and utility function. In this paper we instrument a production scheduler definition to evaluate the potential of augmented schedulers in practical use, and experiment with awareness to application traffic properties by using a multi-class utility function, yielding scheduling policies that behave differently depending on the features of individual traffic classes.
更多查看译文
关键词
offline reinforcement learning,multiclass utility function,augmented schedulers,production scheduler definition,scheduling policy,ODDS process,offline Monte Carlo exploration,instrumented baseline scheduler,cross-layer state parameters,LTE MAC scheduler algorithms,reinforcement learning technique,target deployment scenario,augmented scheduling algorithms,optimized cross-layer schedulers,automated offline design process,practical cross-layer MAC schedulers
AI 理解论文
溯源树
样例
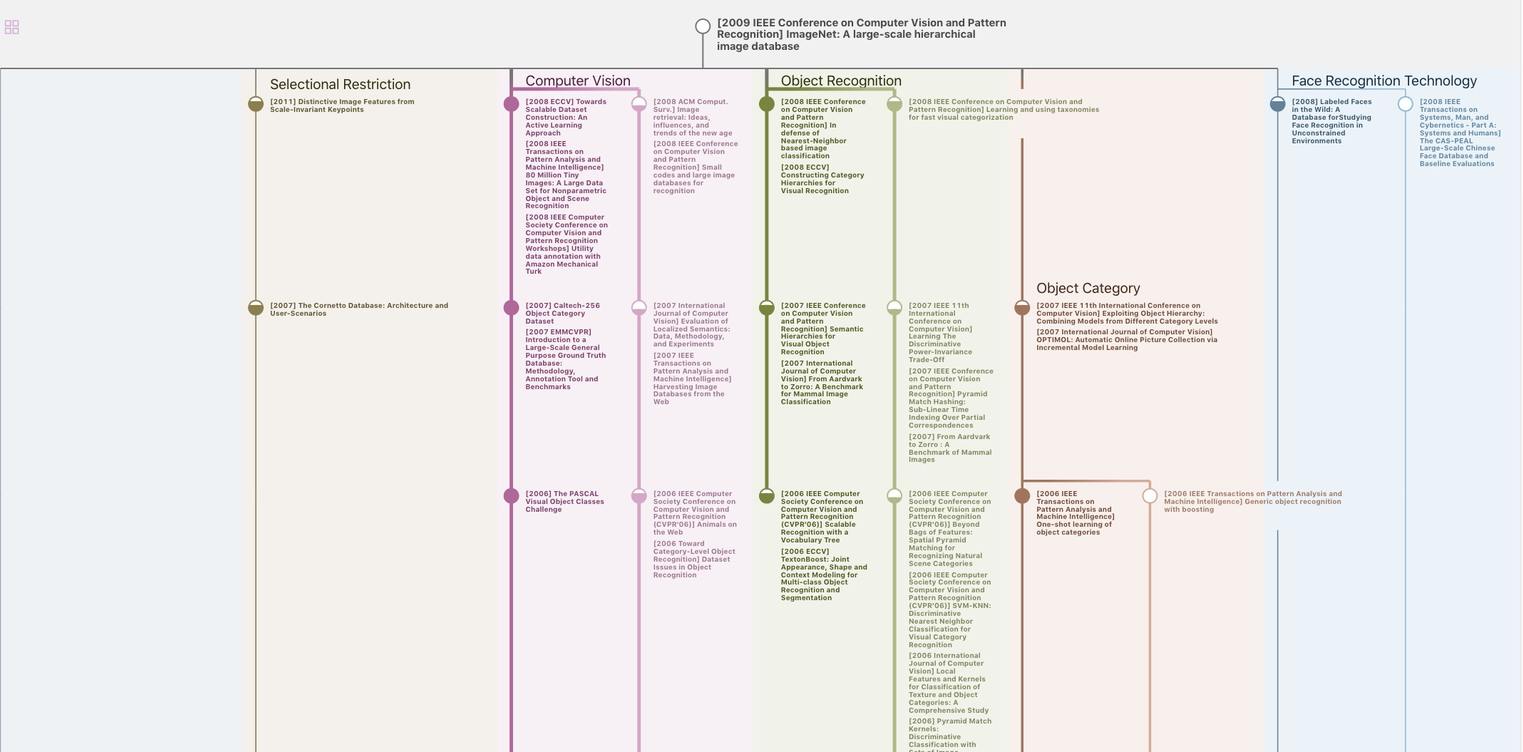
生成溯源树,研究论文发展脉络
Chat Paper
正在生成论文摘要