Evolving cauchy possibilistic clustering and its application to large-scale cyberattack monitoring
2017 IEEE SYMPOSIUM SERIES ON COMPUTATIONAL INTELLIGENCE (SSCI)(2017)
摘要
This paper gives the idea of large-scale monitoring for cyberattacks using evolving Cauchy possibilistic clustering (eCauchy). The idea of density based clustering is appealing when the data samples are highly noisy and when also the outliers appears frequently. The basic measure of density in recursive form can be modified in a way to be applied on classification problems such as large-scale monitoring for cyberattacks. The algorithm is in on-line form to deal with the data streams and is therefore appropriate for dealing with big-data problems. The development of density as a measure of similarity follows from Cauchy density and is similar to the typicality defined in the possibilistic clustering approach. The described eCauchy clustering deals with just few tuning parameters, such as maximal density. The algorithm evolves the structure during operation by adding and removing the clusters. This is appropriate for data granulation which is of great importance in the case of the clusters which are of different sizes and shapes. In the proposed large-scale monitoring system, darknet sensor packets within a certain period are transformed into 17 traffic features and they are categorized by eCauchy in an on-line fashion. To evaluate the proposed darknet monitoring system, a large set of TCP and UDP packets collected from January 2nd 2016 to March 1st 2016 (60 days) with the NICT /16 darknet sensor are used for evaluation. Our experimental results demonstrate that the proposed monitoring system can detect DDoS backscatter with more than 98% accuracy for TCP packets and non-DDoS backscatter with 72.8 % accuracy for UDP packets. The proposed system can learn and predict quite fast, 12.6 sec. for TCP and 312.6 sec. for UDP.
更多查看译文
关键词
evolving cauchy possibilistic clustering,large-scale cyberattack monitoring,density based clustering,data samples,recursive form,classification problems,on-line form,time 312.6 s,time 12.6 s,time 60.0 d,DDoS backscatter detection,TCP packets,eCauchy clustering,large-scale monitoring,UDP packets,darknet monitoring system,darknet sensor packets,large-scale monitoring system,data granulation,maximal density,possibilistic clustering approach,Cauchy density,big-data problems,data streams
AI 理解论文
溯源树
样例
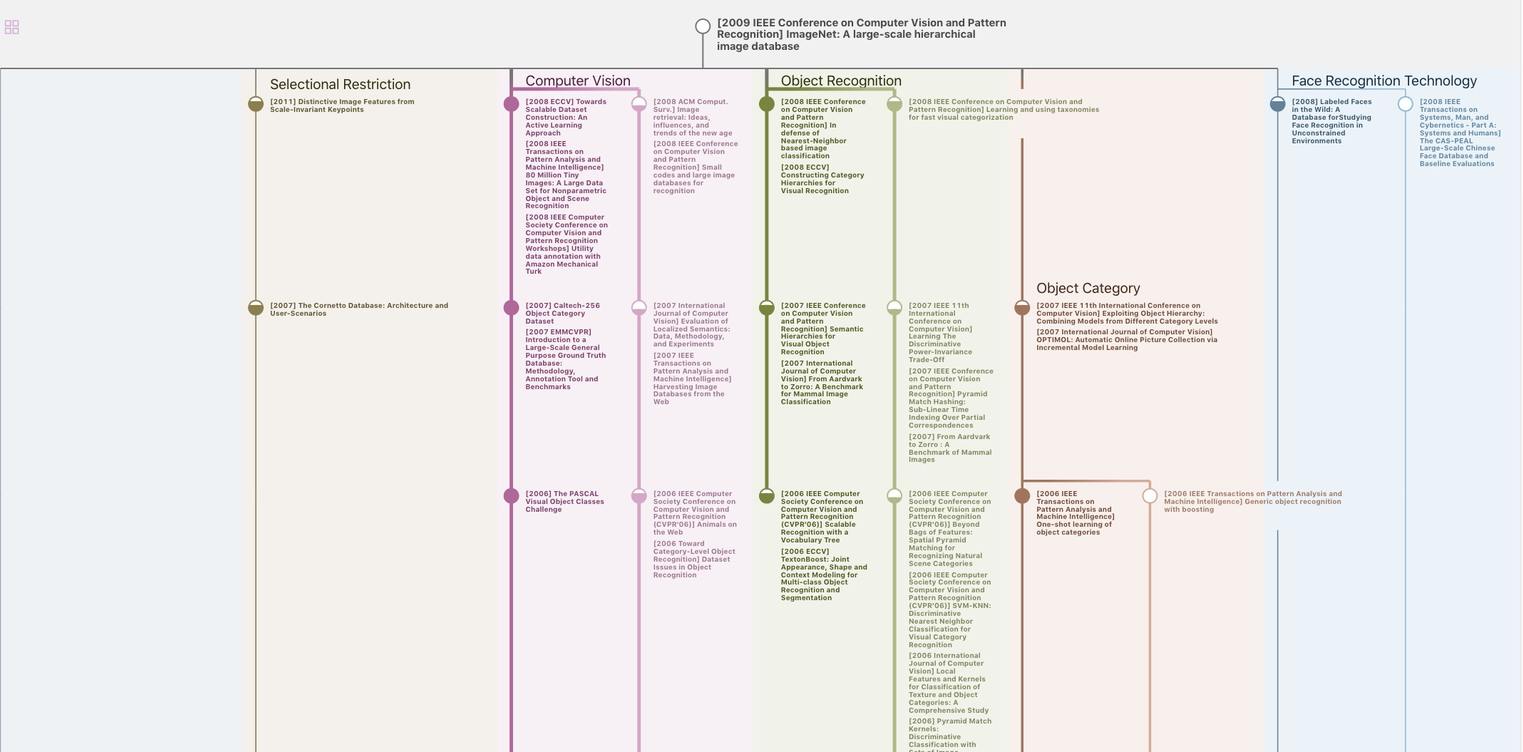
生成溯源树,研究论文发展脉络
Chat Paper
正在生成论文摘要