Approximate Computing Techniques for Iterative Graph Algorithms
2017 IEEE 24th International Conference on High Performance Computing (HiPC)(2017)
摘要
Approximate computing enables processing of large-scale graphs by trading off quality for performance. Approximate computing techniques have become critical not only due to the emergence of parallel architectures but also due to the availability of large scale datasets enabling data-driven discovery. Using two prototypical graph algorithms, PageRank and community detection, we present several approximate computing heuristics to scale the performance with minimal loss of accuracy. We present several heuristics including loop perforation, data caching, incomplete graph coloring and synchronization, and evaluate their efficiency. We demonstrate performance improvements of up to 83% for PageRank and up to 450x for community detection, with low impact on accuracy for both the algorithms. We expect the proposed approximate techniques will enable scalable graph analytics on data of importance to several applications in science and their subsequent adoption to scale similar graph algorithms.
更多查看译文
关键词
Approximate Computing,Graph Algorithms,PageRank,Community Detection,Parallel Algorithms
AI 理解论文
溯源树
样例
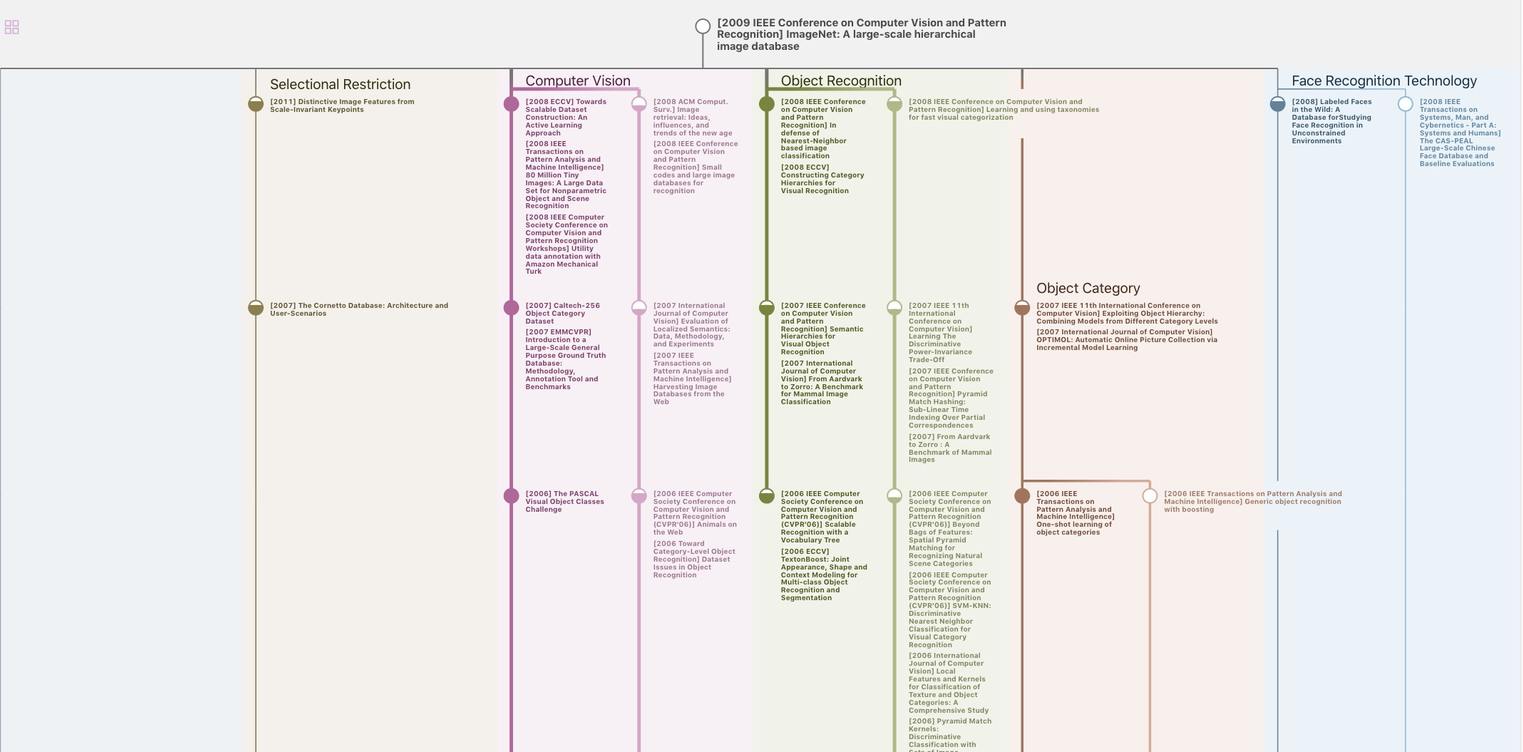
生成溯源树,研究论文发展脉络
Chat Paper
正在生成论文摘要