Mixed Sparsity Regularized Multi-View Unsupervised Feature Selection
2017 24TH IEEE INTERNATIONAL CONFERENCE ON IMAGE PROCESSING (ICIP)(2017)
摘要
The traditional learning machines suffer from the curse of dimensionality because of the data explosion in the areas of multi-media, social network, etc. Feature selection is an effective technique to reduce storage burden and time complexity, and improve generalization ability of the learned models. In real-world applications, the data can be collected from different modalities, or described from multi-views as well. Compared with supervised cases, it is more challenging to reduce the feature dimensionality of multi-view data in unsupervised circumstances. The key difficulty with multi view unsupervised feature selection is how to characterize the multi-view relationships. In this paper, we propose a novel method for multi-view unsupervised feature selection by imposing sparsity on both individual features and views. To exploit the complementary information, we also take the view importance into consideration without introducing explicit view weights. Experiments on benchmark datasets show on the proposed algorithm outperforms other unsupervised feature selection methods
更多查看译文
关键词
multi-view learning, unsupervised feature selection, group sparsity, parameter-free learning
AI 理解论文
溯源树
样例
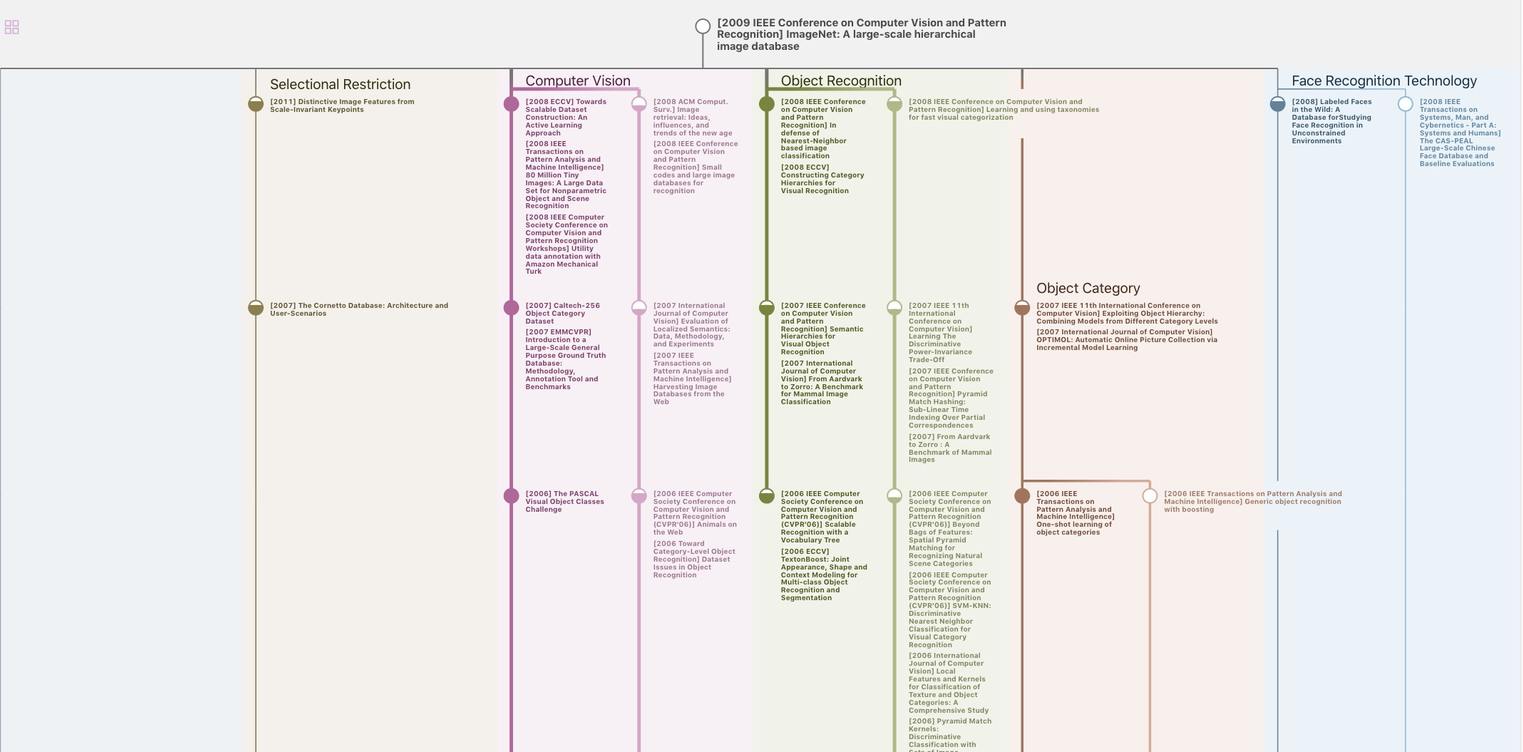
生成溯源树,研究论文发展脉络
Chat Paper
正在生成论文摘要