Computed Tomography Super-Resolution Using Convolutional Neural Networks
2017 24TH IEEE INTERNATIONAL CONFERENCE ON IMAGE PROCESSING (ICIP)(2017)
摘要
The practical application of Computed Tomography (CT) faces the dilemma between higher image resolution and less X-ray exposure for patients, motivating the research on CT super-resolution (SR). In this paper, we apply state-of-the-art SR techniques to reconstruct CT images using two proposed advanced CT SR models based on Convolutional Neural Networks (CNNs) and residual learning: a single-slice CT SR network (S-CTSRN), and a multi-slice CT SR network (M-CTSRN). S-CTSRN improves the high-frequency feature extraction by incorporating the residual learning strategy, while M-CTSRN further utilizes the coherence between neighboring CT slices for better SR reconstruction. We evaluate both models on a large-scale CT dataset(1), and obtain competitive results both quantitatively and qualitatively.
更多查看译文
关键词
Super-resolution (SR), Medical Image Analysis, Computed Tomography (CT), Convolutional Neural Network (CNN), Residual Learning
AI 理解论文
溯源树
样例
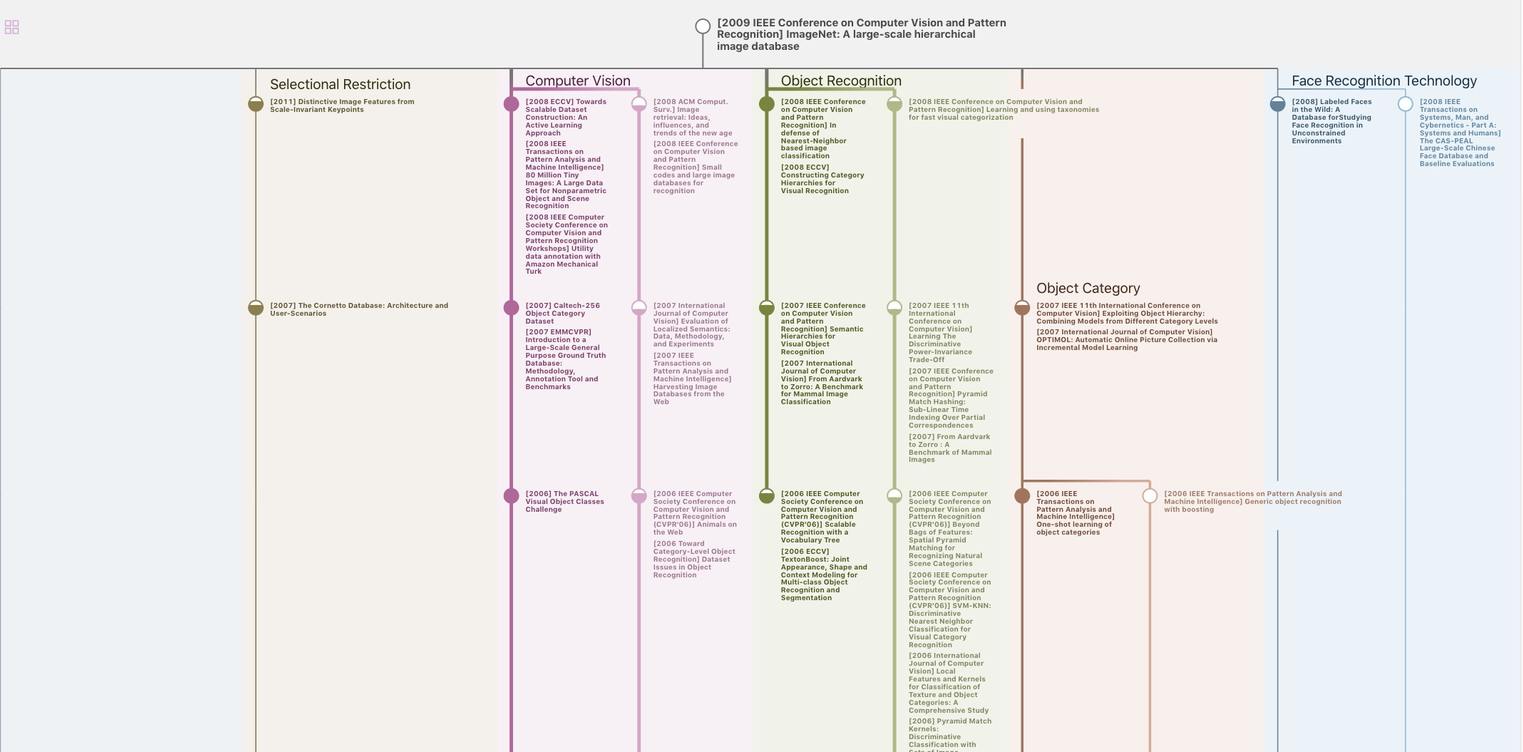
生成溯源树,研究论文发展脉络
Chat Paper
正在生成论文摘要