Transferring Cnns To Multi-Instance Multi-Label Classification On Small Datasets
2017 24TH IEEE INTERNATIONAL CONFERENCE ON IMAGE PROCESSING (ICIP)(2017)
摘要
Image tagging is a well known challenge in image processing. It is typically addressed through multi-instance multi-label (MIME) classification methodologies. Convolutional Neural Networks (CNNs) possess great potential to perform well on MIML tasks, since multi-level convolution and max pooling coincide with the multi-instance setting and the sharing of hidden representation may benefit multi-label modeling. However, CNNs usually require a large amount of carefully labeled data for training, which is hard to obtain in many real applications. In this paper, we propose a new approach for transferring pre-trained deep networks such as VGG16 on lmagenet to small MIML tasks. We extract features from each group of the network layers and apply multiple binary classifiers to them for multi-label prediction. Moreover, we adopt an L-1-norm regularized Logistic Regression (L1LR) to find the most effective features for learning the multi-label classifiers. The experiment results on two most-widely used and relatively small benchmark MIML image datasets demonstrate that the proposed approach can substantially outperform the state-of-the-art algorithms, in terms of all popular performance metrics.
更多查看译文
关键词
CNN, Multi-instance, Multi-label, Small dataset, Transfer Learning
AI 理解论文
溯源树
样例
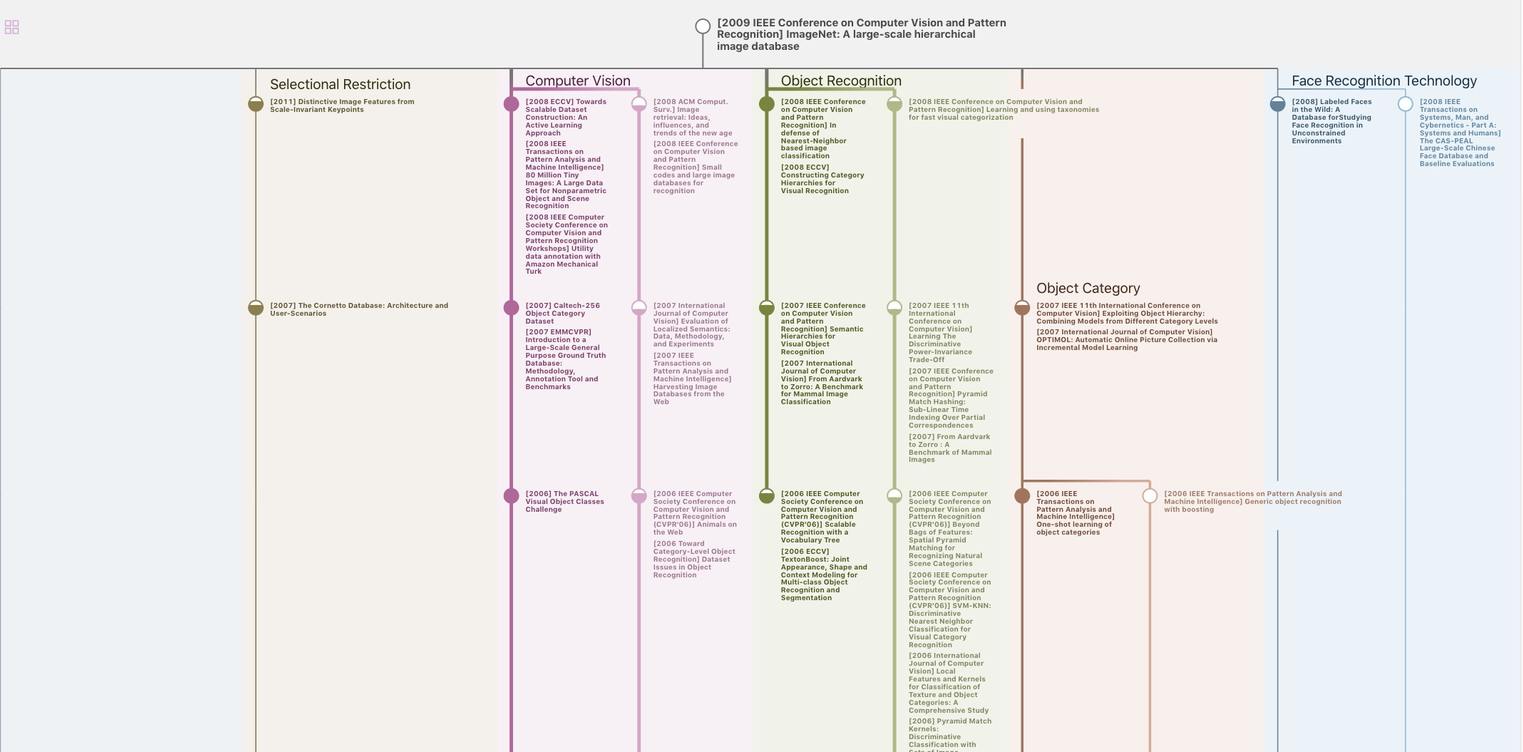
生成溯源树,研究论文发展脉络
Chat Paper
正在生成论文摘要