Deep Tracking With Objectness
2017 24TH IEEE INTERNATIONAL CONFERENCE ON IMAGE PROCESSING (ICIP)(2017)
摘要
Visual tracking is a fundamental problem in computer vision. However, due to the (sometimes) ambiguous target information given at the first frame, it has also been criticized as less well-posed compared with other tasks with clearly-defined targets, such as object detection and semantic segmentation. In this paper, we try to evaluate the importance of object category in visual tracking by tracking objects with known object types. The proposed algorithm, termed Deep-Track with Objectness (DTO), naturally combines the state-of-the-art deep-learning-based detectors and trackers, which essentially share a large part of the network. In DTO, a deep tracker, which is scale-fixed and sensitive to small translations tracks the object in a relative short lifespan. A deep detector, which is scale-changeable and robust to pose or illumination changes guides the deep tracker in a longer lifespan. As the deep tracker and detector share the main part of their networks, no much extra computation is imposed while the performance gain is significant. We test the proposed algorithm on two well-accepted benchmarks and on both of them, the proposed method increases the tracking accuracies remarkably compared with state-of-the-art visual trackers.
更多查看译文
关键词
visual tracking, deep learning, object detection
AI 理解论文
溯源树
样例
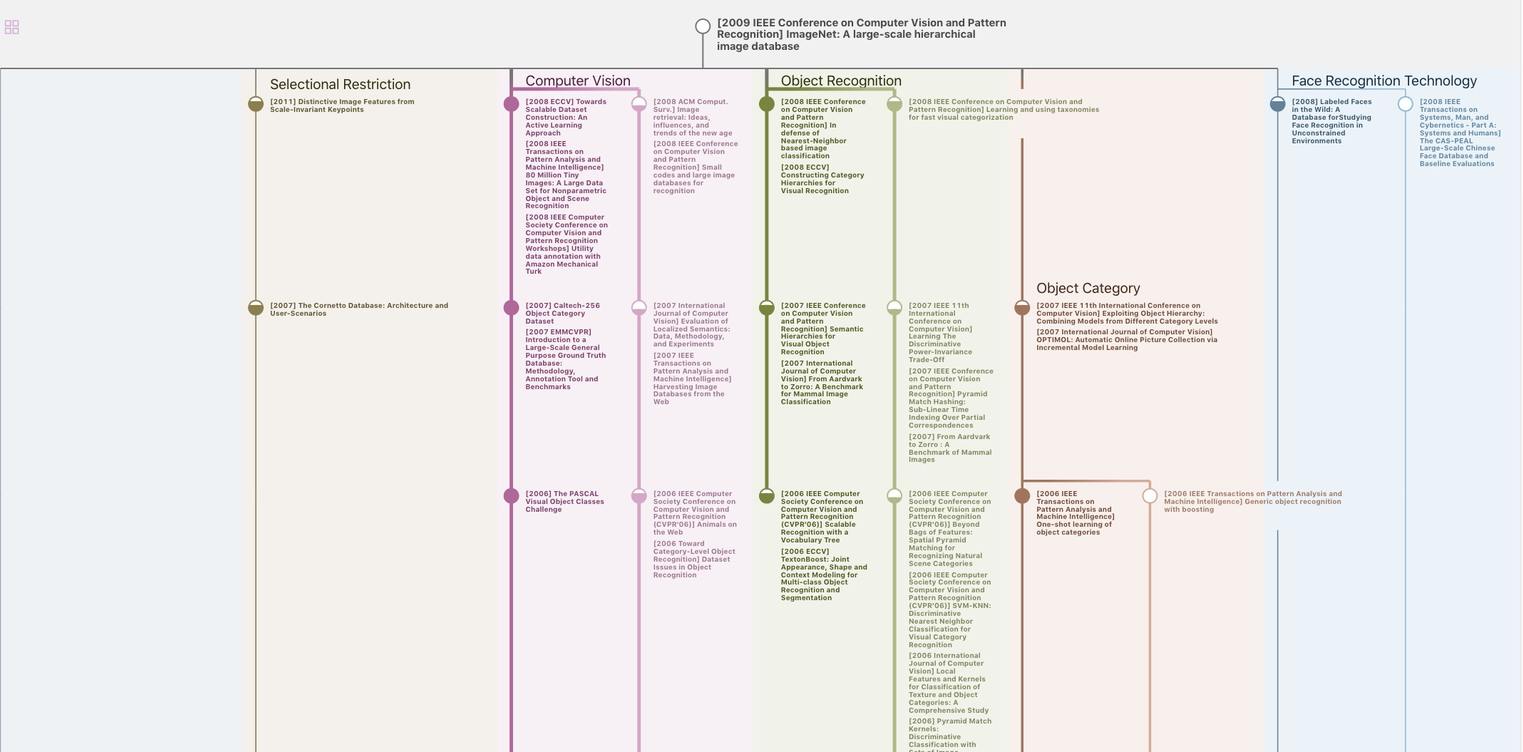
生成溯源树,研究论文发展脉络
Chat Paper
正在生成论文摘要