PSVM: a preference-enhanced SVM model using preference data for classification
SCIENCE CHINA Information Sciences(2017)
摘要
Classification is an essential task in data mining, machine learning and pattern recognition areas. Conventional classification models focus on distinctive samples from different categories. There are fine-grained differences between data instances within a particular category. These differences form the preference information that is essential for human learning, and, in our view, could also be helpful for classification models. In this paper, we propose a preference-enhanced support vector machine (PSVM), that incorporates preference-pair data as a specific type of supplementary information into SVM. Additionally, we propose a two-layer heuristic sampling method to obtain effective preference-pairs, and an extended sequential minimal optimization (SMO) algorithm to fit PSVM. To evaluate our model, we use the task of knowledge base acceleration-cumulative citation recommendation (KBA-CCR) on the TREC-KBA-2012 dataset and seven other datasets from UCI, StatLib and mldata.org. The experimental results show that our proposed PSVM exhibits high performance with official evaluation metrics.
更多查看译文
关键词
preference,SVM,classification,sampling,sequential minimal optimization (SMO)
AI 理解论文
溯源树
样例
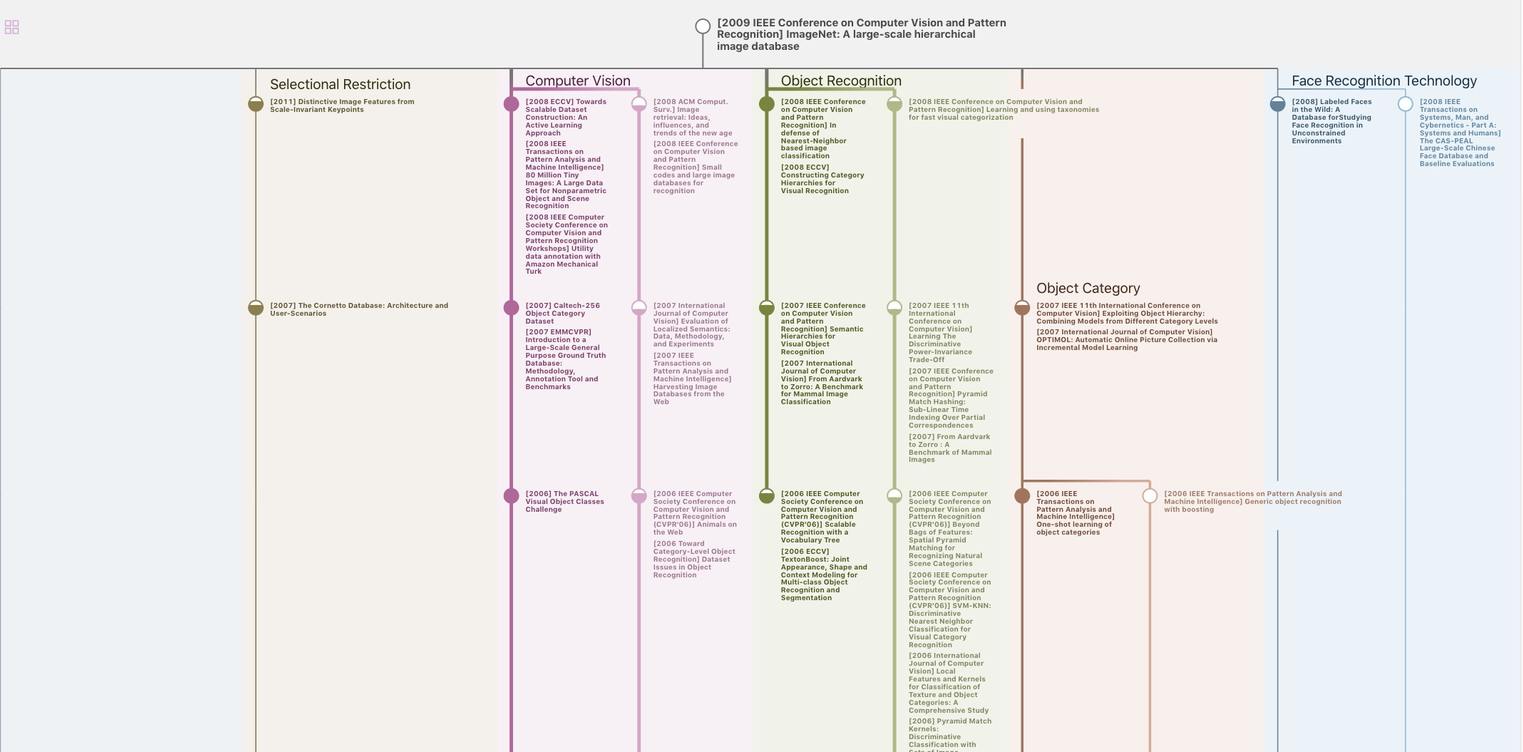
生成溯源树,研究论文发展脉络
Chat Paper
正在生成论文摘要