Quantile regression for additive coefficient models in high dimensions
Journal of Multivariate Analysis(2018)
摘要
In this paper, we consider quantile regression in additive coefficient models (ACM) with high dimensionality under a sparsity assumption and approximate the additive coefficient functions by B-spline expansion. First, we consider the oracle estimator for quantile ACM when the number of additive coefficient functions is diverging. Then we adopt the SCAD penalty and investigate the non-convex penalized estimator for model estimation and variable selection. Under some regularity conditions, we prove that the oracle estimator is a local solution of the SCAD penalized quantile regression problem. Simulation studies and an application to a genome-wide association study show that the proposed method yields good numerical results.
更多查看译文
关键词
62G05,62G20
AI 理解论文
溯源树
样例
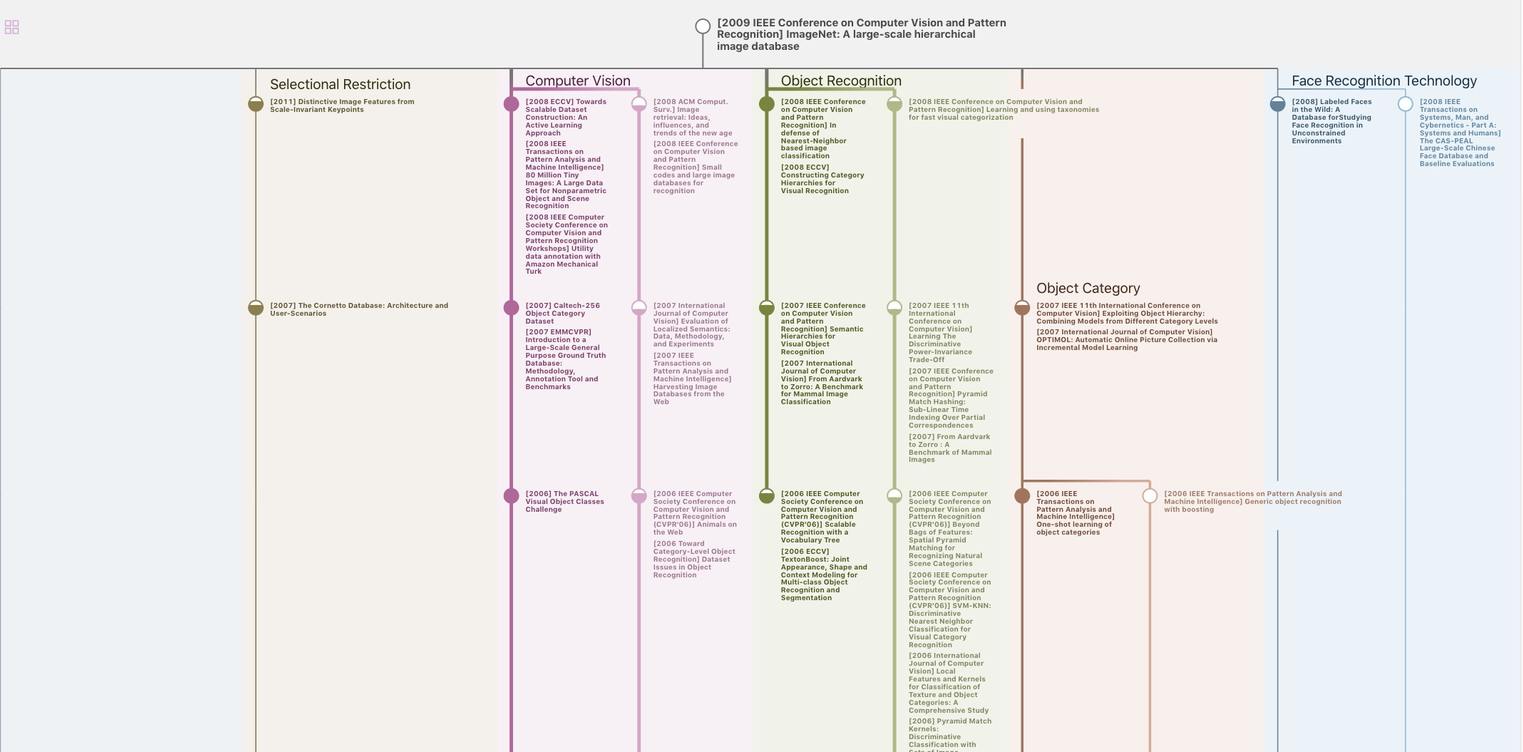
生成溯源树,研究论文发展脉络
Chat Paper
正在生成论文摘要