Ballpark Crowdsourcing: The Wisdom of Rough Group Comparisons.
WSDM 2018: The Eleventh ACM International Conference on Web Search and Data Mining Marina Del Rey CA USA February, 2018(2018)
摘要
Crowdsourcing has become a popular method for collecting labeled training data. However, in many practical scenarios traditional labeling can be difficult for crowdworkers(for example, if the data is high-dimensional or unintuitive, or the labels are continuous).
In this work, we develop a novel model for crowdsourcing that can complement standard practices by exploiting people»s intuitions about groups and relations between them. We employ a recent machine learning setting, called Ballpark Learning, that can estimate individual labels given only coarse, aggregated signal over groups of data points. To address the important case of continuous labels, we extend the Ballpark setting(which focused on classification) to regression problems. We formulate the problem as a convex optimization problem and propose fast, simple methods with an innate robustness to outliers.
We evaluate our methods on real-world datasets, demonstrating how useful constraints about groups can be harnessed from a crowd of non-experts. Our methods can rival supervised models trained on many true labels, and can obtain considerably better results from the crowd than a standard label-collection process(for a lower price). By collecting rough guesses on groups of instances and using machine learning to infer the individual labels, our lightweight framework is able to address core crowdsourcing challenges and train machine learning models in a cost-effective way.
更多查看译文
AI 理解论文
溯源树
样例
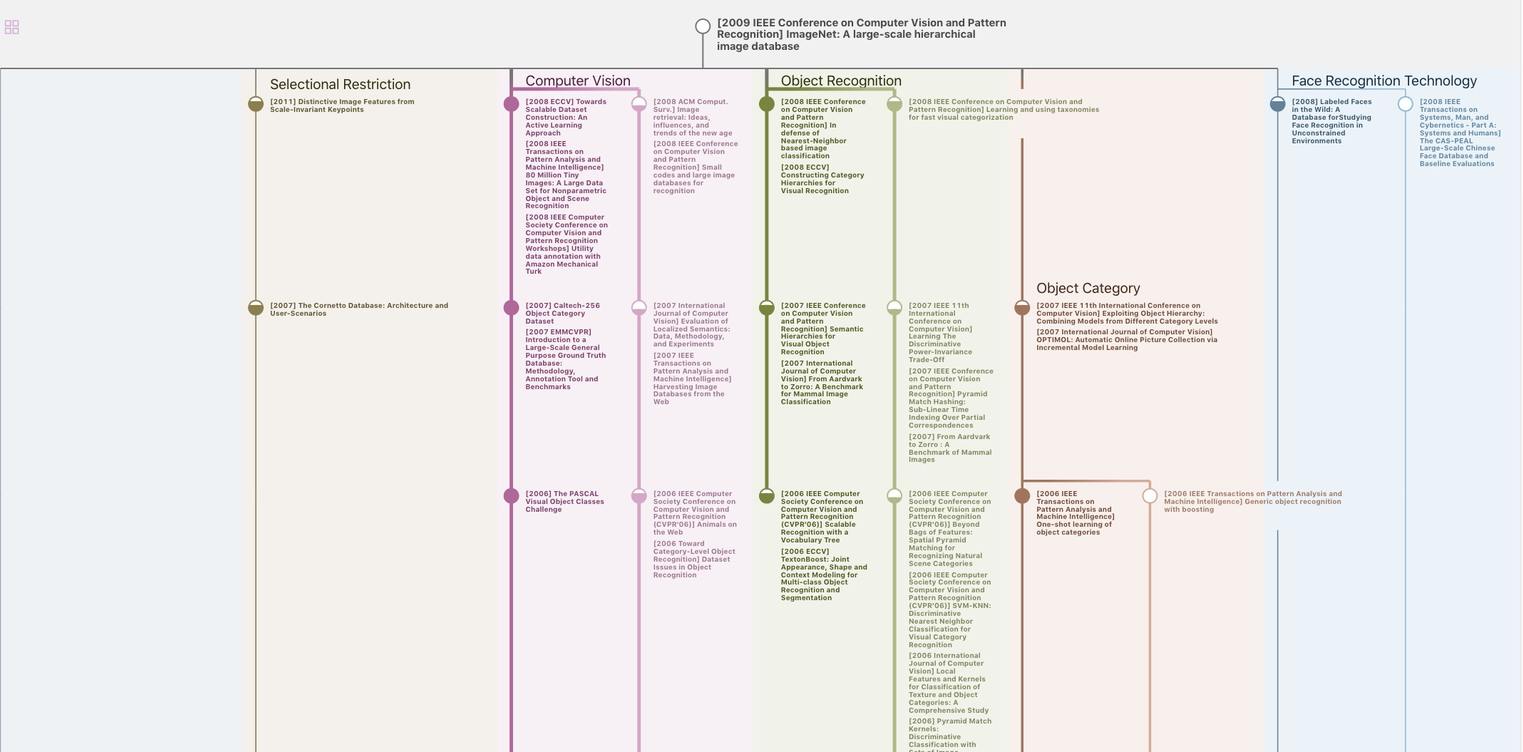
生成溯源树,研究论文发展脉络
Chat Paper
正在生成论文摘要