Network Intrusion Detection through Discriminative Feature Selection by Using Sparse Logistic Regression.
FUTURE INTERNET(2017)
摘要
Intrusion detection system (IDS) is a well-known and effective component of network security that provides transactions upon the network systems with security and safety. Most of earlier research has addressed difficulties such as overfitting, feature redundancy, high-dimensional features and a limited number of training samples but feature selection. We approach the problem of feature selection via sparse logistic regression (SPLR). In this paper, we propose a discriminative feature selection and intrusion classification based on SPLR for IDS. The SPLR is a recently developed technique for data analysis and processing via sparse regularized optimization that selects a small subset from the original feature variables to model the data for the purpose of classification. A linear SPLR model aims to select the discriminative features from the repository of datasets and learns the coefficients of the linear classifier. Compared with the feature selection approaches, like filter (ranking) and wrapper methods that separate the feature selection and classification problems, SPLR can combine feature selection and classification into a unified framework. The experiments in this correspondence demonstrate that the proposed method has better performance than most of the well-known techniques used for intrusion detection.
更多查看译文
关键词
sparse logistic regression (SPLR),intrusion detection system (IDS),computer network security,data mining (DM),machine learning (ML)
AI 理解论文
溯源树
样例
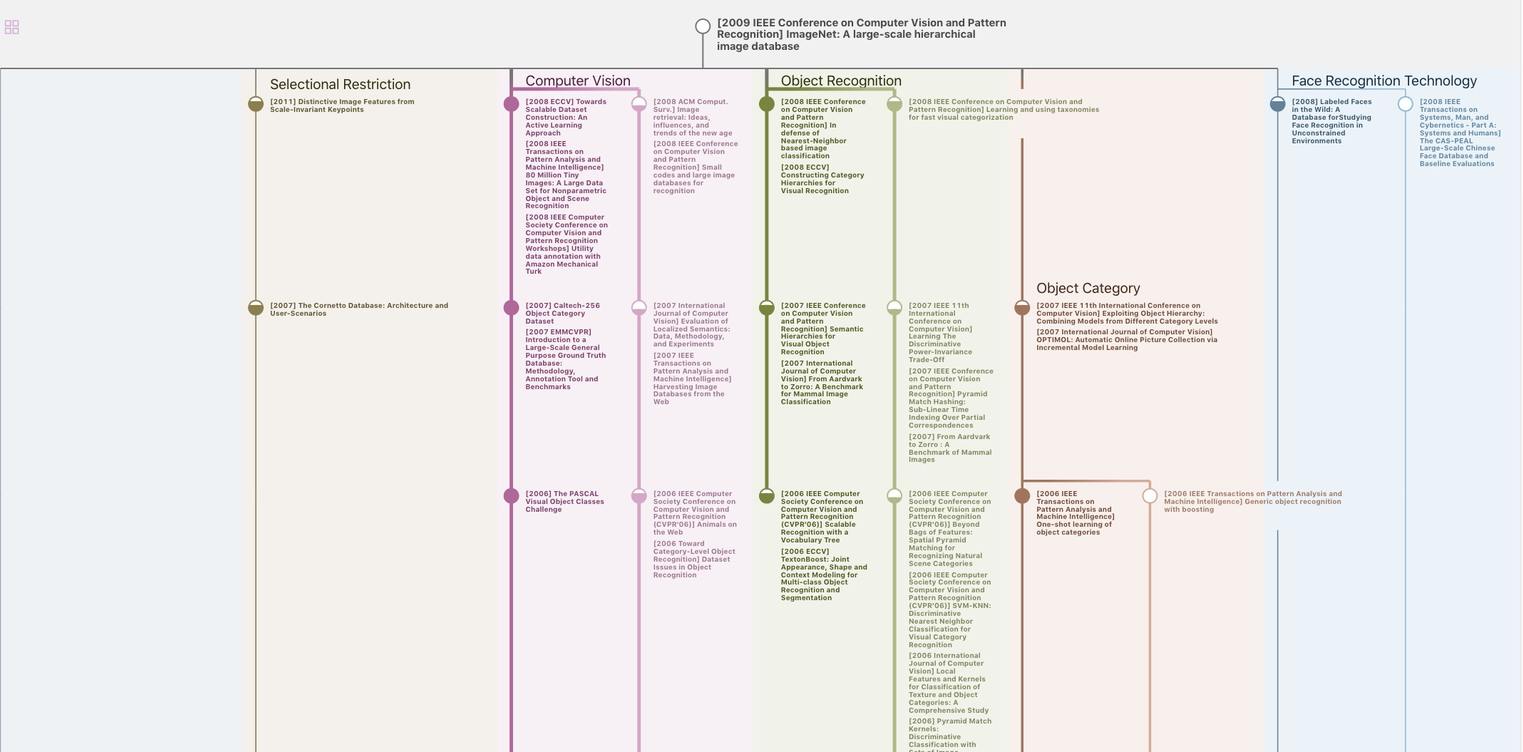
生成溯源树,研究论文发展脉络
Chat Paper
正在生成论文摘要