An automated framework for QSAR model building.
J. Cheminformatics(2018)
摘要
We developed an extendable and highly customizable fully automated QSAR modeling framework. This designed workflow does not require any advanced parameterization nor depends on users decisions or expertise in machine learning/programming. With just a given target or problem, the workflow follows an unbiased standard protocol to develop reliable QSAR models by directly accessing online manually curated databases or by using private data sets. The other distinctive features of the workflow include prior estimation of data modelability to avoid time-consuming modeling trials for non modelable data sets, an efficient variable selection procedure and the facility of output availability at each modeling task for the diverse application and reproduction of historical predictions. The results reached on a selection of thirty QSAR problems suggest that the approach is capable of building reliable models even for challenging problems.
更多查看译文
关键词
Data set modelability,Feature selection,KNIME,Machine learning,Quantitative structure–activity relationship (QSAR),Random forests,Support vector machines,Variable importance
AI 理解论文
溯源树
样例
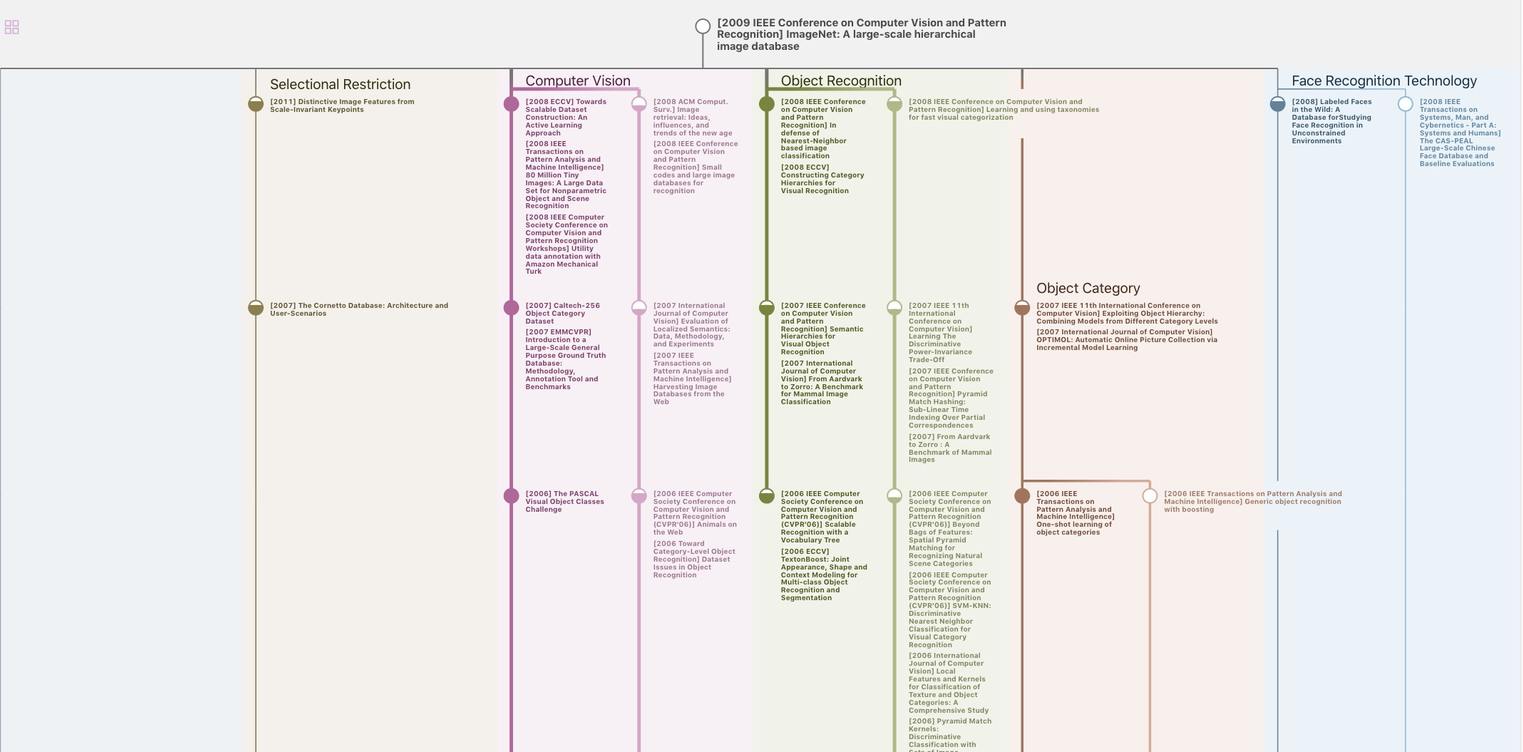
生成溯源树,研究论文发展脉络
Chat Paper
正在生成论文摘要