On Lower Bounds for Statistical Learning Theory.
ENTROPY(2017)
摘要
In recent years, tools from information theory have played an increasingly prevalent role in statistical machine learning. In addition to developing efficient, computationally feasible algorithms for analyzing complex datasets, it is of theoretical importance to determine whether such algorithms are "optimal" in the sense that no other algorithm can lead to smaller statistical error. This paper provides a survey of various techniques used to derive information-theoretic lower bounds for estimation and learning. We focus on the settings of parameter and function estimation, community recovery, and online learning for multi-armed bandits. A common theme is that lower bounds are established by relating the statistical learning problem to a channel decoding problem, for which lower bounds may be derived involving information-theoretic quantities such as the mutual information, total variation distance, and Kullback-Leibler divergence. We close by discussing the use of information-theoretic quantities to measure independence in machine learning applications ranging from causality to medical imaging, and mention techniques for estimating these quantities efficiently in a data-driven manner.
更多查看译文
关键词
machine learning,minimax estimation,community recovery,online learning,multi-armed bandits,channel decoding,threshold phenomena
AI 理解论文
溯源树
样例
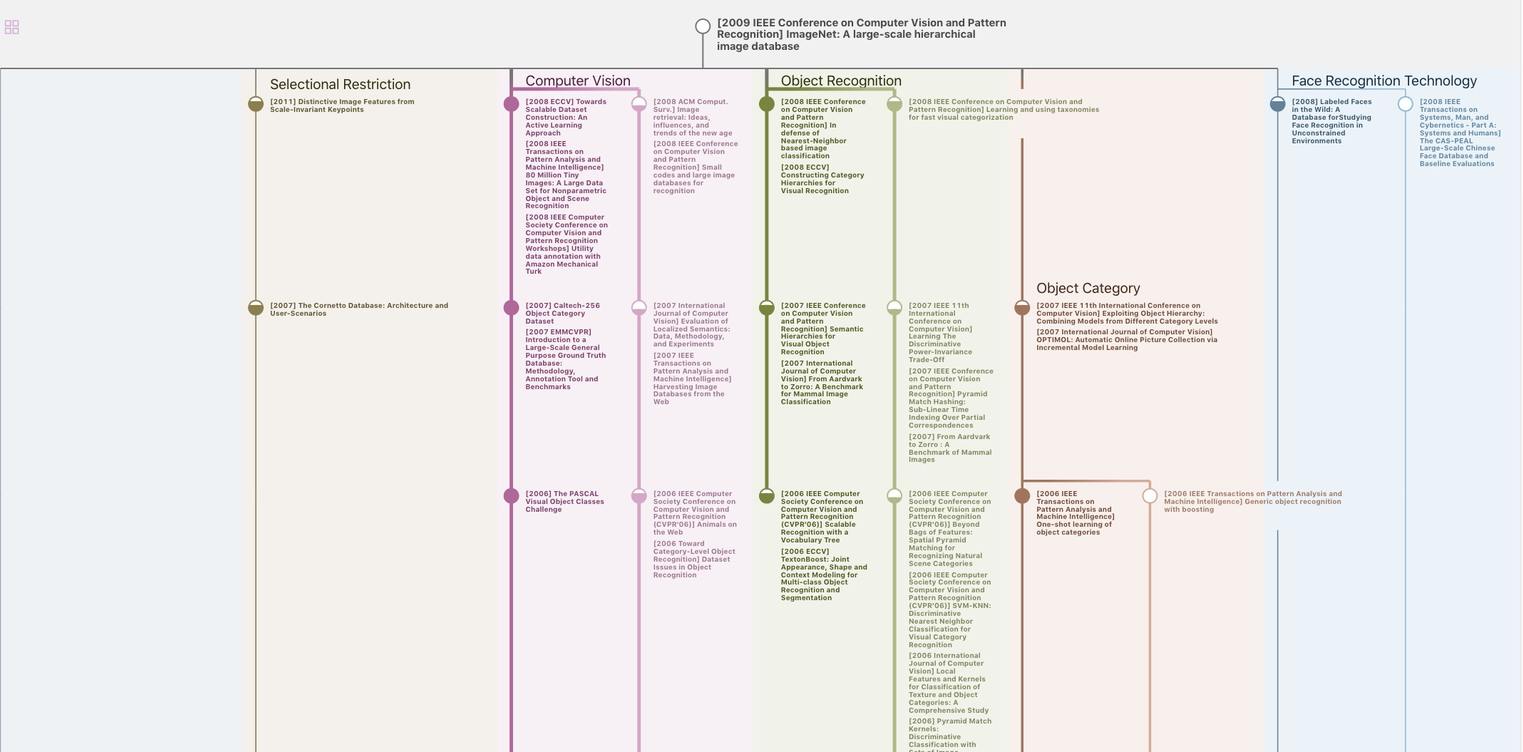
生成溯源树,研究论文发展脉络
Chat Paper
正在生成论文摘要