Point-pattern matching based on point pair local topology and probabilistic relaxation labeling
The Visual Computer(2016)
摘要
This paper presents a robust point-pattern matching (PPM) algorithm, in which the invariant feature and probabilistic relaxation labeling are combined to improve the assignment accuracy and efficiency. A local feature descriptor, namely, point pair local topology (PPLT), is proposed first. The feature descriptor is defined by histogram which is constructed using the weighting of distance measures and angle measures based on local point pair. We use the matching scores of point pair local topology descriptor’s statistic test to define new compatibility coefficients. Then, the robust support functions are constructed based on the obtained compatibility coefficients. Finally, according to the relaxed iterations of matching probability matrix and the mapping constraints required by the bijective correspondence, the correct matching results are obtained. A number of comparison and evaluation experiments on both synthetic point sets and real-world data demonstrate that the proposed algorithm performs better in the presence of outliers and positional jitter. In addition, it achieves the superior performance under similarity and even nonrigid transformation among point sets in the meantime compared with state-of-the-art approaches.
更多查看译文
关键词
Point-pattern matching (PPM),Point pair local topology (PPLT),Probabilistic relaxation labeling,Compatibility coefficients
AI 理解论文
溯源树
样例
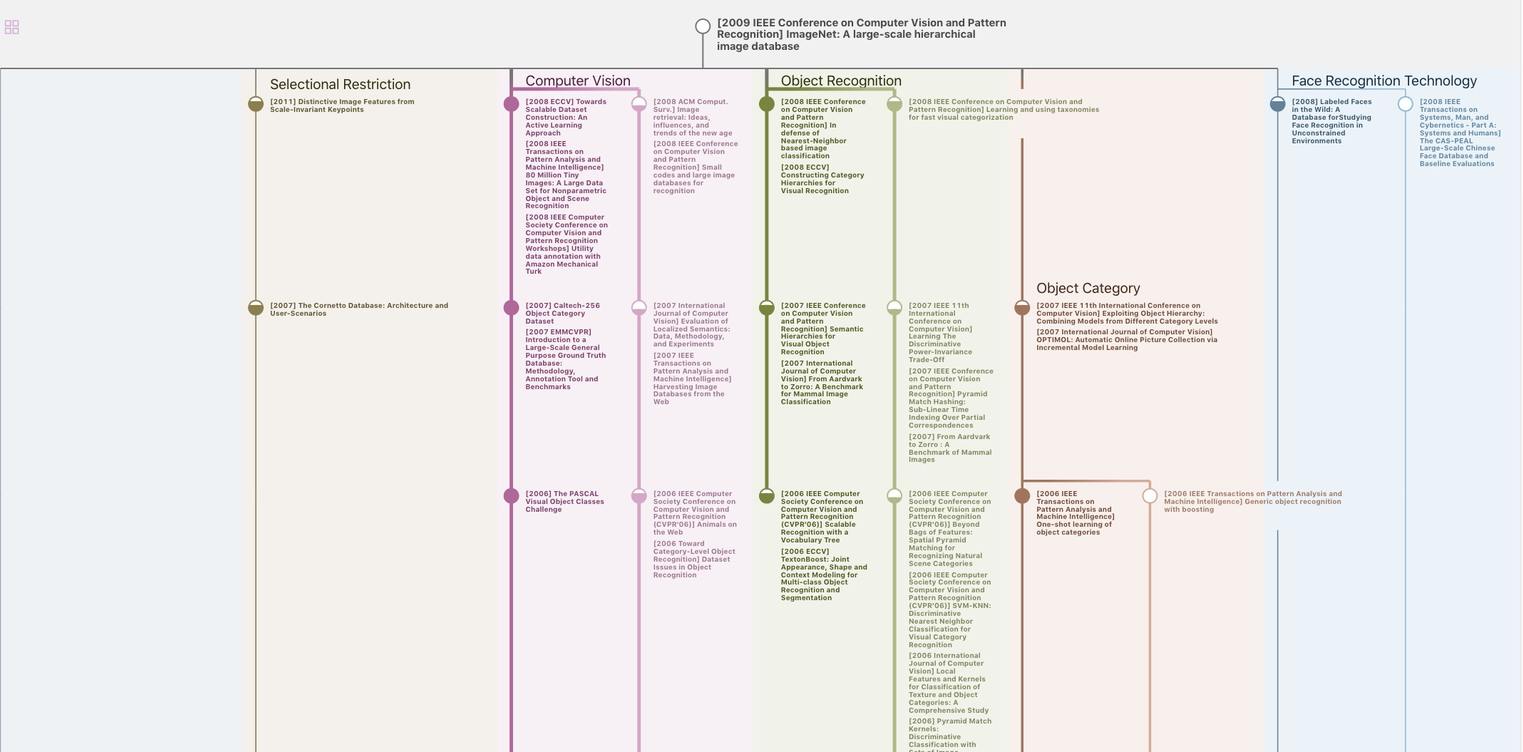
生成溯源树,研究论文发展脉络
Chat Paper
正在生成论文摘要