Analog Network-Coded Modulation With Maximum Euclidean Distance: Mapping Criterion and Constellation Design.
IEEE ACCESS(2017)
摘要
Physical-layer network coding holds the great potential of improving the power efficiency and the spectral efficiency for the two-stage transmission scheme. The first stage is the multiple access stage, where two source nodes (SN1 and SN2) simultaneously transmit to the relay node (RN). The second stage is the Broadcast stage, where the RN broadcasts to the two destination nodes (DN1 and DN2), after a denoising-and-mapping operation. In this paper, we investigate the joint network-coded modulation design of the two stages. A universal modulation framework is built, referred to as analog network-coded modulation strategy, which is more general than the former modulation design mechanism. More explicitly, we propose a joint design criterion to guarantee the forwarding reliability at the RN. The criterion ensures that the neighboring constellation points superposed at the RN are mapped to an identical constellation point for broadcasting if their Euclidean distance (ED) is less than a given threshold. This yields a non-convex polynomial optimization problem by minimizing the average transmission power and constraining the ED among the constellation points. By solving the problem, we propose two joint modulation design algorithms, termed as the Enhanced Semidefinite Relaxation Algorithm and the Fast-Relaxation Algorithm, respectively. The two algorithms can achieve the tradeoff between the communication performance and the computation resources. As for the Fast-Relaxation Algorithm, the theoretical performance boundary is derived in detail. Simulation results demonstrate the effectiveness of both the proposed algorithms by comparing symbol error rate performance with the existing modulation design methods.
更多查看译文
关键词
Two-way relaying,network coding,modulation coding,physical layer,constellation design,optimization methods,relaxation methods,semidefinite programming,Gaussian randomization
AI 理解论文
溯源树
样例
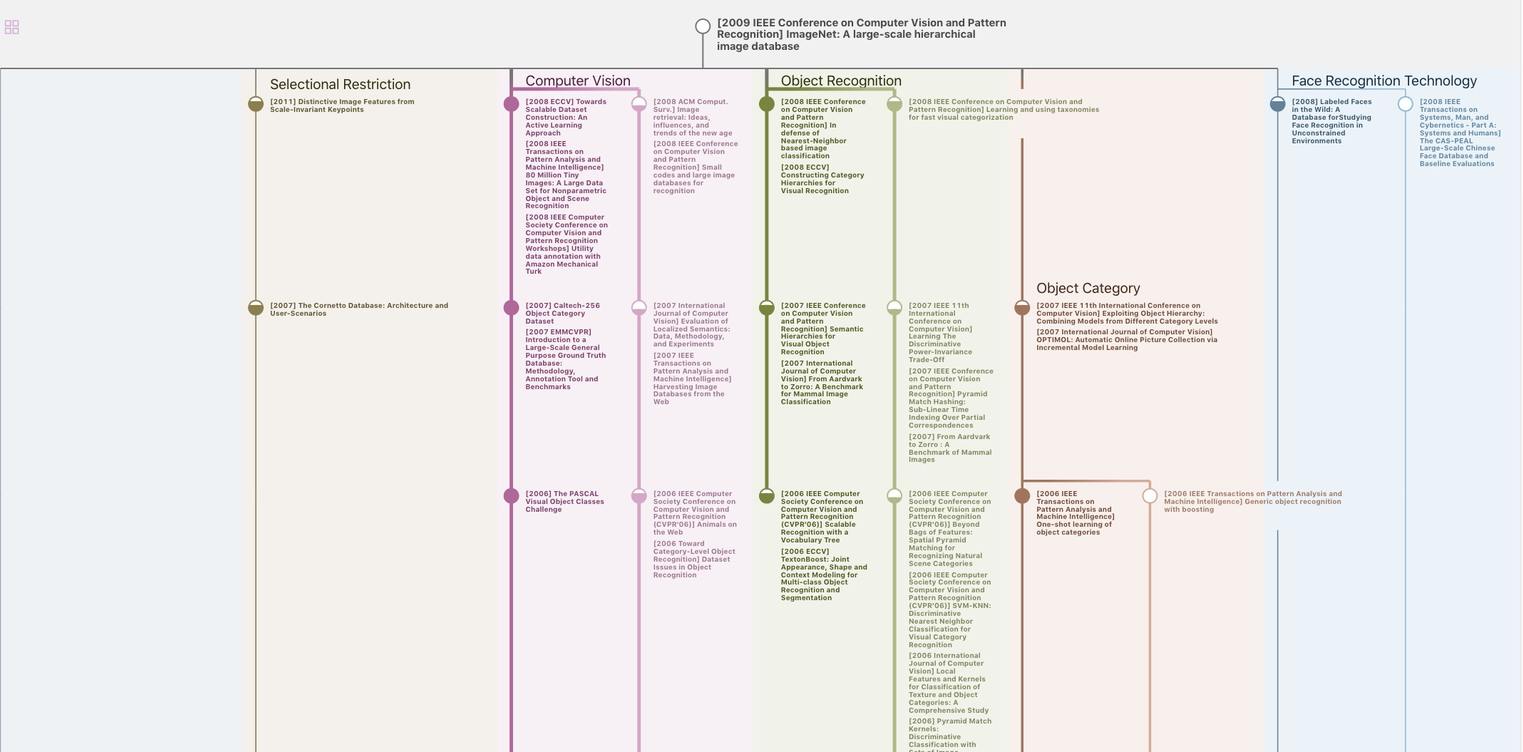
生成溯源树,研究论文发展脉络
Chat Paper
正在生成论文摘要