A Modified Differential Evolution With Distance-based Selection for Continuous Optimization in Presence of Noise.
IEEE ACCESS(2017)
摘要
The performance of evolutionary algorithms (EAs), suitable for optimization on static functional landscapes, usually degrade in presence of noises with different statistical features. In this paper, we present a simple variant of the differential evolution (DE) algorithm, one of the most competitive EAs of recent interest, to tackle complex optimization problems in the presence of additive noise. The proposed DE variant is equipped with three new algorithmic components. A new population central tendency-based mutation scheme is proposed and it is switched in a probabilistic manner with the difference mean-based perturbation strategy in the mutation step. Instead of the regular binomial or exponential crossover of DE, we adopt a blending crossover during the recombination stage. Finally, a novel distance-based selection mechanism is incorporated to enable the occasional inclusion of a few inferior solutions to future generations, thus making the usual DE selection less greedy. Five different additive noise models namely Gaussian, Poisson, Rayleigh, Exponential, and Random are considered with a variety of noise amplitudes to simulate the noisy behavior of the objective functions. In total, 79 benchmark functions from traditional, as well as modern (IEEE CEC 2013 and 2017) test-suites, are used to extensively compare and contrast the proposed method with the other state-of-art evolutionary optimization algorithms, tailor-made for noisy function optimization. Experimental results, supported with the non-parametric statistical tests, indicate that our proposed method is very competitive against the noise-resilient variants of classical as well as very recent evolutionary optimizers, including the winners of the recent IEEE CEC competitions of real parameter optimization on the complex fitness landscapes.
更多查看译文
关键词
Differential evolution,noisy optimization,selection,gaussian noise,poisson noise
AI 理解论文
溯源树
样例
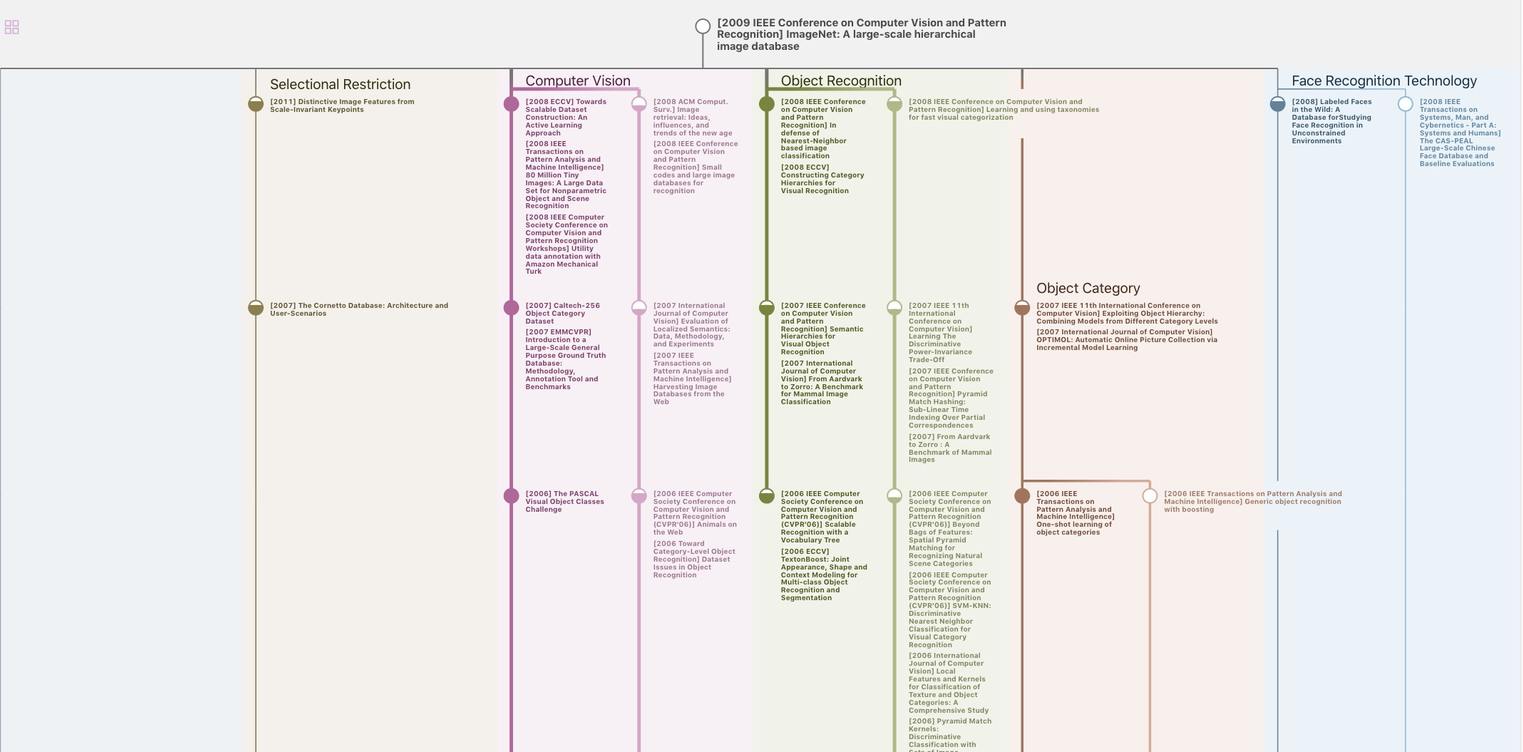
生成溯源树,研究论文发展脉络
Chat Paper
正在生成论文摘要