Online Learning of Optimally Diverse Rankings.
POMACS(2018)
摘要
Search engines answer users' queries by listing relevant items (e.g. documents, songs, products, web pages, ...). These engines rely on algorithms that learn to rank items so as to present an ordered list maximizing the probability that it contains relevant item. The main challenge in the design of learning-to-rank algorithms stems from the fact that queries often have different meanings for different users. In absence of any contextual information about the query, one often has to adhere to the diversity principle, i.e., to return a list covering the various possible topics or meanings of the query. To formalize this learning-to-rank problem, we propose a natural model where (i) items are categorized into topics, (ii) users find items relevant only if they match the topic of their query, and (iii) the engine is not aware of the topic of an arriving query, nor of the frequency at which queries related to various topics arrive, nor of the topic-dependent click-through-rates of the items. For this problem, we devise LDR (Learning Diverse Rankings), an algorithm that efficiently learns the optimal list based on users' feedback only. We show that after T queries, the regret of LDR scales as O((N-L)log(T)) where N is the number of all items. This scaling cannot be improved, i.e., LDR is order optimal.
更多查看译文
关键词
diversity, regret, search engines
AI 理解论文
溯源树
样例
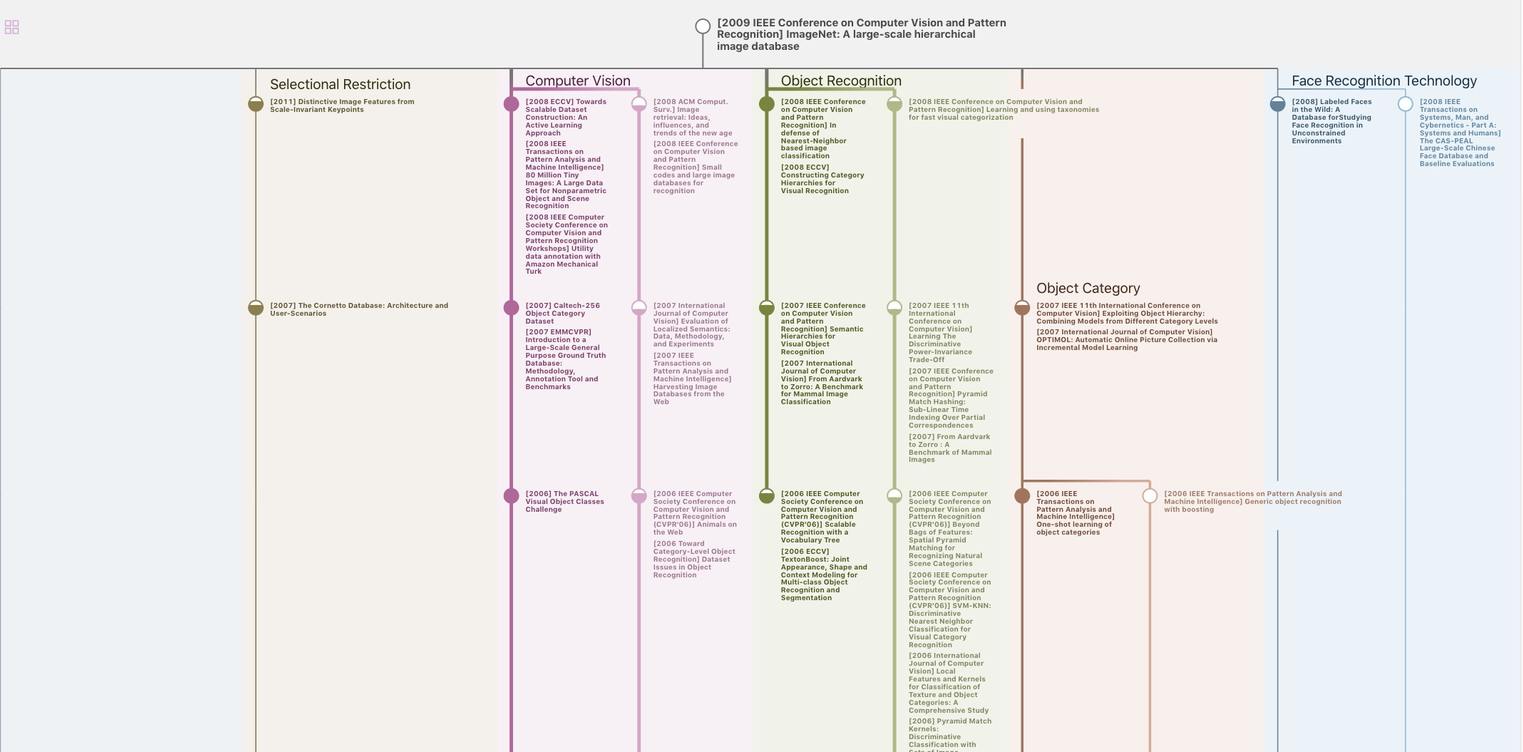
生成溯源树,研究论文发展脉络
Chat Paper
正在生成论文摘要