The k-Nearest Representatives Classifier: A Distance-Based Classifier with Strong Generalization Bounds
2017 IEEE International Conference on Data Science and Advanced Analytics (DSAA)(2017)
摘要
We define the k-Nearest Representatives (k-NR) classifier, a distance-based classifier similar to the k-nearest neighbors classifier with comparable accuracy in practice, and stronger generalization bounds. Uniform convergence is shown through Rademacher complexity, and generalizability is controlled through regularization. Finite-sample risk bound are also given. Compared to the k-NN, the k-NR requires less memory to store and classification queries may be made more efficiently. Training is also efficient, being polynomial in all parameters, and is accomplished via a simple empirical risk minimization process.
更多查看译文
关键词
Classification,Statistical Learning Theory,Rademacher Complexity,VC Dimension,Nearest Neighbor,Quantization,Regularization,Empirical Risk Minimization
AI 理解论文
溯源树
样例
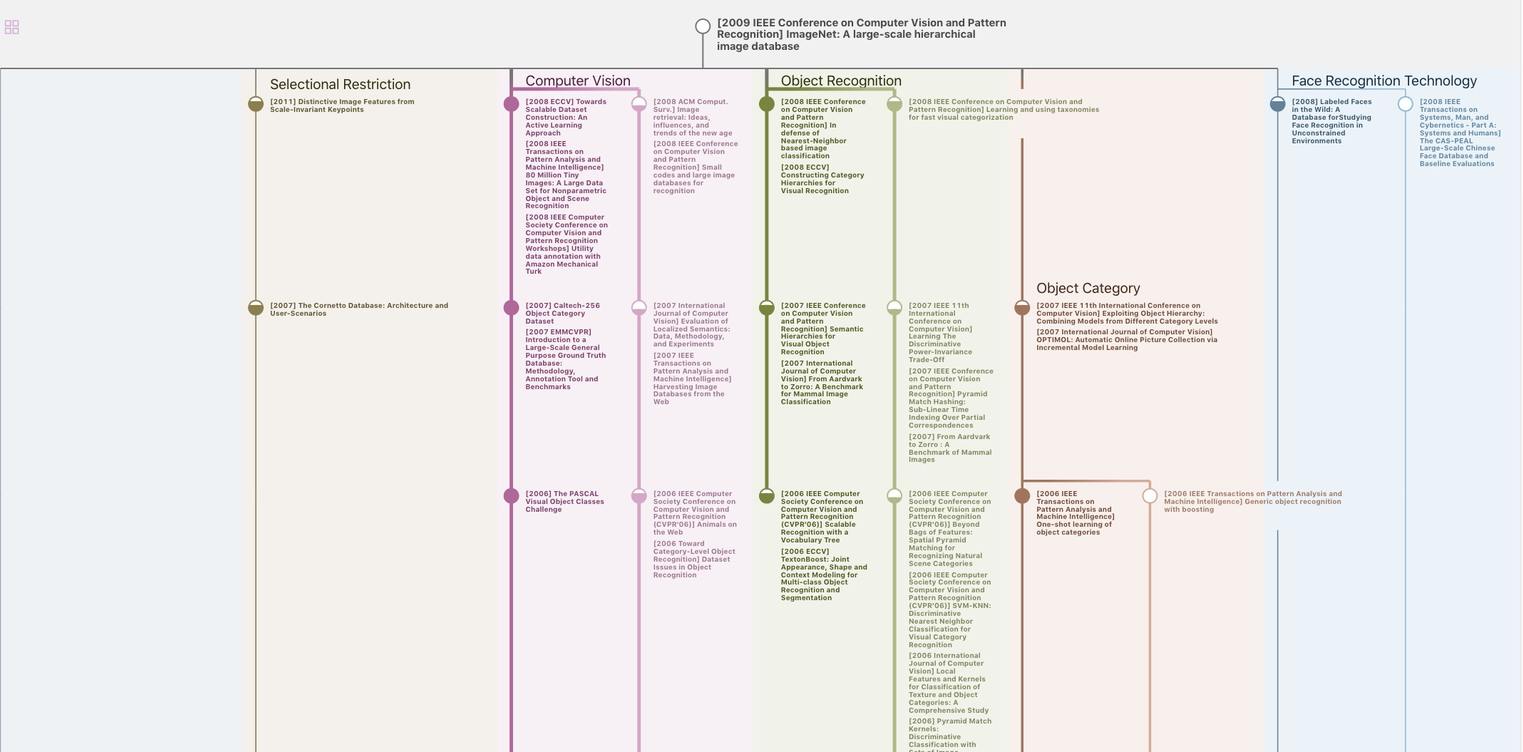
生成溯源树,研究论文发展脉络
Chat Paper
正在生成论文摘要