Predicting Changes in Pediatric Medical Complexity using Large Longitudinal Health Records.
AMIA(2018)
摘要
Medically complex patients consume a disproportionate amount of care resources in hospitals but still often end up with sub-optimal clinical outcomes. Predicting dynamics of complexity in such patients can potentially help improve the quality of care and reduce utilization of hospital resources. In this work, we model the change prediction of medical complexity using a large dataset of 226K pediatric patients over 5 years from Children's Healthcare of Atlanta (CHOA). We compare different classification methods including logistic regression, random forest, gradient boosting trees, and multilayer perceptron in predicting whether patients will change their complexity status in the last year based on the data from previous years. We achieved an area under the ROC curve (AUC) of 88% for predicting noncomplex patients becoming complex and 74% for predicting complex patients staying complex. We also identify the factors associated with the change in complexity of patients.
更多查看译文
AI 理解论文
溯源树
样例
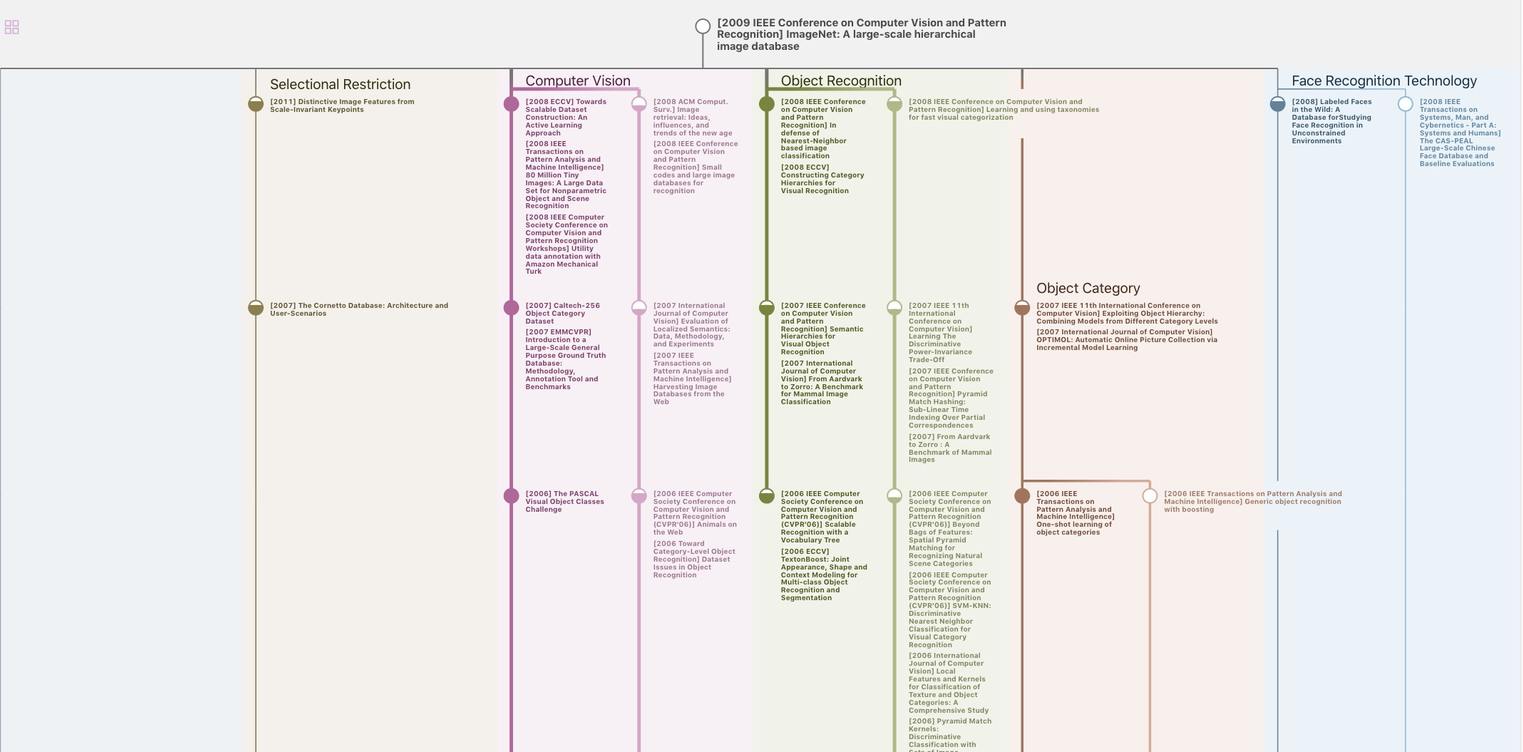
生成溯源树,研究论文发展脉络
Chat Paper
正在生成论文摘要