Attention-Sharing Correlation Learning For Cross-Media Retrieval
IMAGE AND GRAPHICS (ICIG 2017), PT I(2017)
摘要
Cross-media retrieval is a challenging research topic with wide prospect of application, aiming to retrieve among different media types by using a single-media query. The main challenge of cross-media retrieval is to learn the correlation between different media types for addressing the issue of "media gap". The close semantic correlation usually lies in specific parts of cross-media data such as image and text, which plays the key role for precious correlation mining. However, existing works usually focus on correlation learning in the level of whole media instance, or adopt patch segmentation but treat the patches indiscriminately. They ignore the fine-grained discrimination learning, which limits the retrieval accuracy. Inspired by attention mechanism, this paper proposes the attention-sharing correlation learning network, which is an end-to-end network to generate cross-media common representation for retrieval. By sharing the common attention weights, the attention of different media types can be learned coordinately. It can not only emphasize the single-media discriminative parts, but also enhance the cross-media fine-grained consistent pattern, and so learn more precious cross-media correlation to improve retrieval accuracy. Experimental results on 2 widely-used datasets with state-of-the-art methods verify the effectiveness of the proposed approach.
更多查看译文
AI 理解论文
溯源树
样例
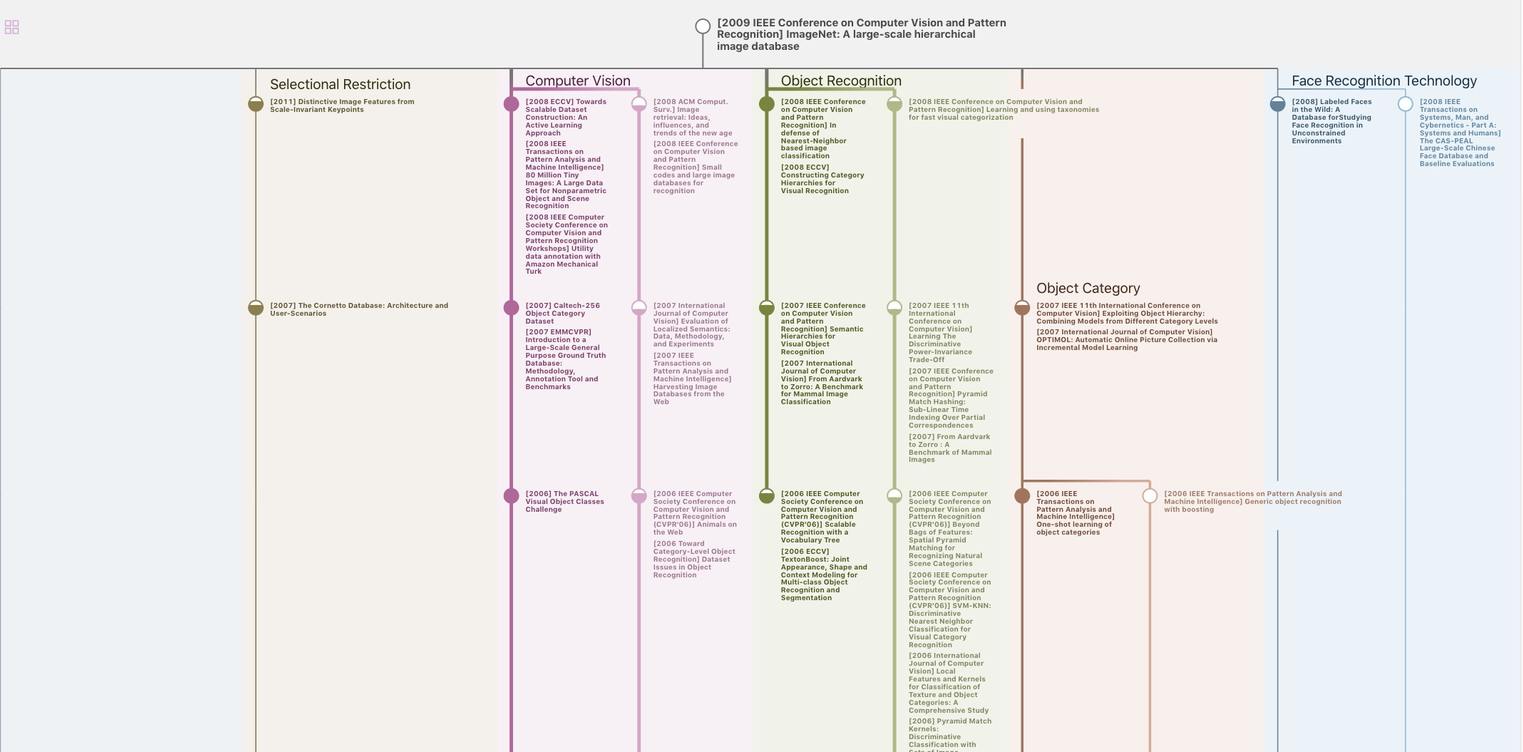
生成溯源树,研究论文发展脉络
Chat Paper
正在生成论文摘要