Deepcd: Learning Deep Complementary Descriptors For Patch Representations
2017 IEEE INTERNATIONAL CONFERENCE ON COMPUTER VISION (ICCV)(2017)
摘要
This paper presents the DeepCD framework which learns a pair of complementary descriptors jointly for image patch representation by employing deep learning techniques. It can be achieved by taking any descriptor learning architecture for learning a leading descriptor and augmenting the architecture with an additional network stream for learning a complementary descriptor. To enforce the complementary property, a new network layer, called data-dependent modulation (DDM) layer, is introduced for adaptively learning the augmented network stream with the emphasis on the training data that are not well handled by the leading stream. By optimizing the proposed joint loss function with late fusion, the obtained descriptors are complementary to each other and their fusion improves performance. Experiments on several problems and datasets show that the proposed method(1) is simple yet effective, outperforming state-of-the-art methods.
更多查看译文
关键词
patch representations,DeepCD framework,image patch representation,deep learning techniques,descriptor learning architecture,leading descriptor,network layer,called data-dependent modulation layer,augmented network stream,leading stream,deep complementary descriptors
AI 理解论文
溯源树
样例
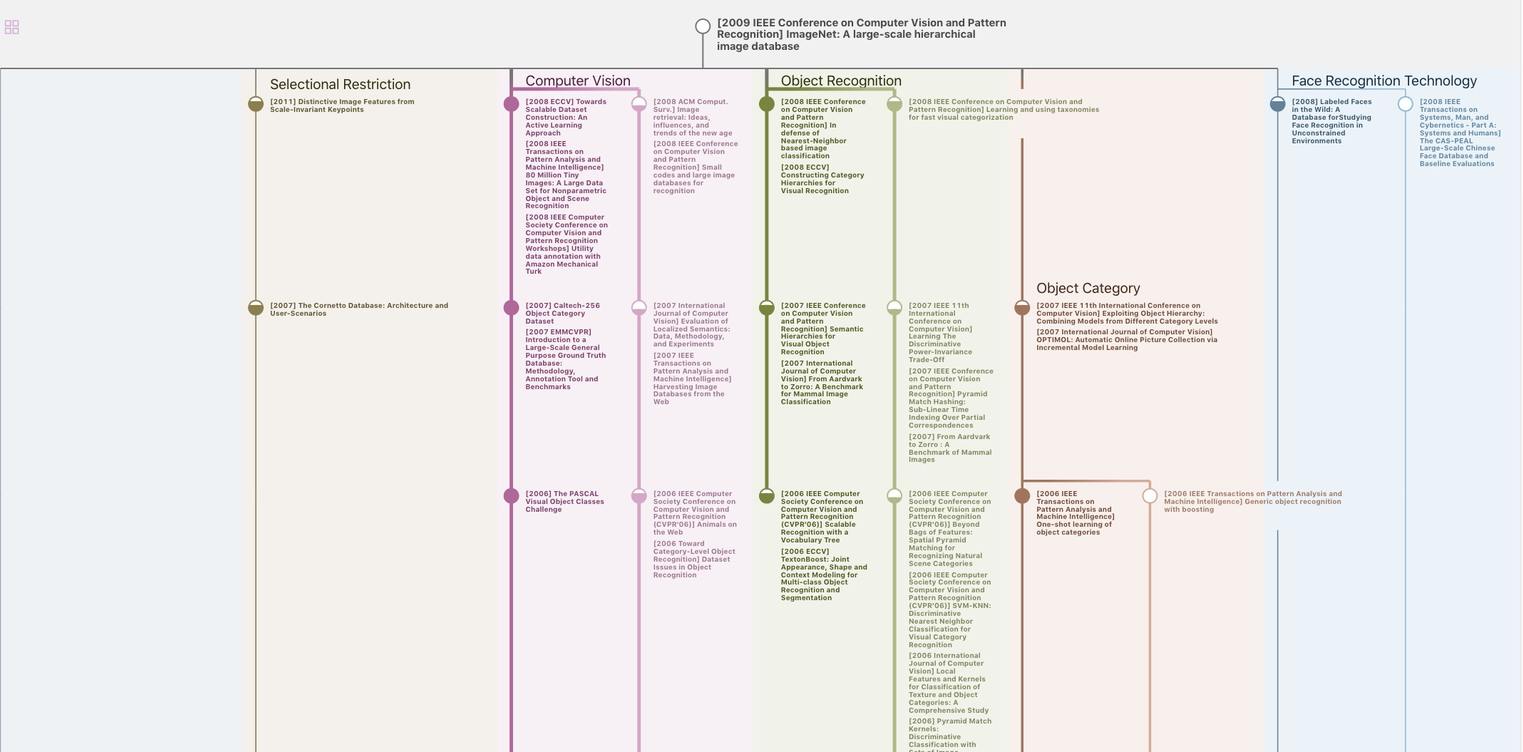
生成溯源树,研究论文发展脉络
Chat Paper
正在生成论文摘要