Learning To Push The Limits Of Efficient Fft-Based Image Deconvolution
2017 IEEE INTERNATIONAL CONFERENCE ON COMPUTER VISION (ICCV)(2017)
摘要
This work addresses the task of non-blind image deconvolution. Motivated to keep up with the constant increase in image size, with megapixel images becoming the norm, we aim at pushing the limits of efficient FFT-based techniques. Based on an analysis of traditional and more recent learning-based methods, we generalize existing discriminative approaches by using more powerful regularization, based on convolutional neural networks. Additionally, we propose a simple, yet effective, boundary adjustment method that alleviates the problematic circular convolution assumption, which is necessary for FFT-based deconvolution. We evaluate our approach on two common non-blind deconvolution benchmarks and achieve state-of-the-art results even when including methods which are computationally considerably more expensive.
更多查看译文
关键词
nonblind image deconvolution,image size,megapixel images,discriminative approaches,convolutional neural networks,learning-based methods,FFT,boundary adjustment method
AI 理解论文
溯源树
样例
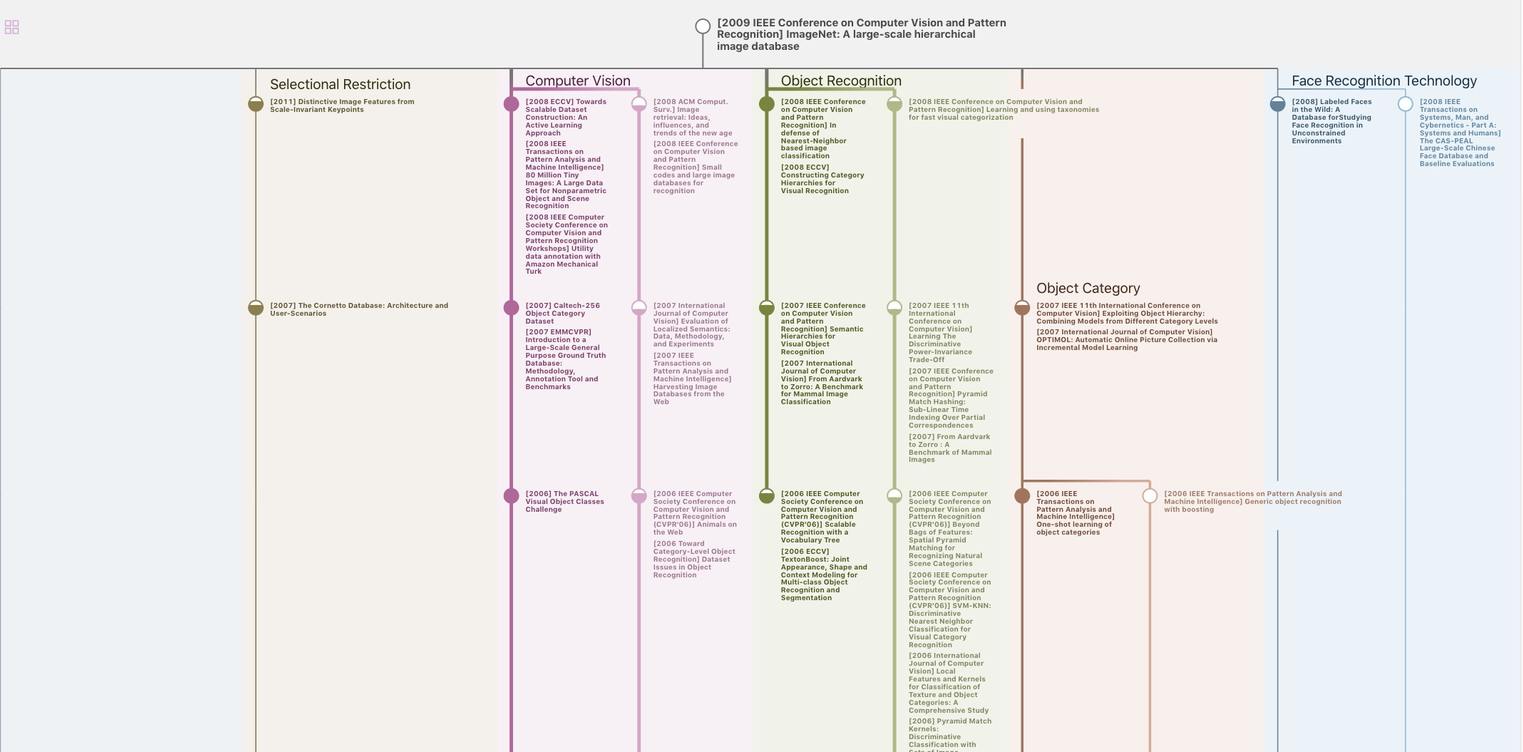
生成溯源树,研究论文发展脉络
Chat Paper
正在生成论文摘要