Synergy Between Face Alignment And Tracking Via Discriminative Global Consensus Optimization
2017 IEEE INTERNATIONAL CONFERENCE ON COMPUTER VISION (ICCV)(2017)
摘要
An open question in facial landmark localization in video is whether one should perform tracking or tracking-by-detection (i.e. face alignment). Tracking produces fittings of high accuracy but is prone to drifting. Tracking-by-detection is drift-free but results in low accuracy fittings.To provide a solution to this problem, we describe the very first, to the best of our knowledge, synergistic approach between detection (face alignment) and tracking which completely eliminates drifting from face tracking, and does not merely perform tracking-by-detection. Our first main contribution is to show that one can achieve this synergy between detection and tracking using a principled optimization framework based on the theory of Global Variable Consensus Optimization using ADMM; Our second contribution is to show how the proposed analytic framework can be integrated within state-of-the-art discriminative methods for face alignment and tracking based on cascaded regression and deeply learned features. Overall, we call our method Discriminative Global Consensus Model (DGCM). Our third contribution is to show that DGCM achieves large performance improvement over the currently best performing face tracking methods on the most challenging category of the 300-VW dataset.
更多查看译文
关键词
face alignment,tracking-by-detection,drift-free but results,face tracking,discriminative global consensus model,discriminative global consensus optimization,global variable consensus optimization,VW
AI 理解论文
溯源树
样例
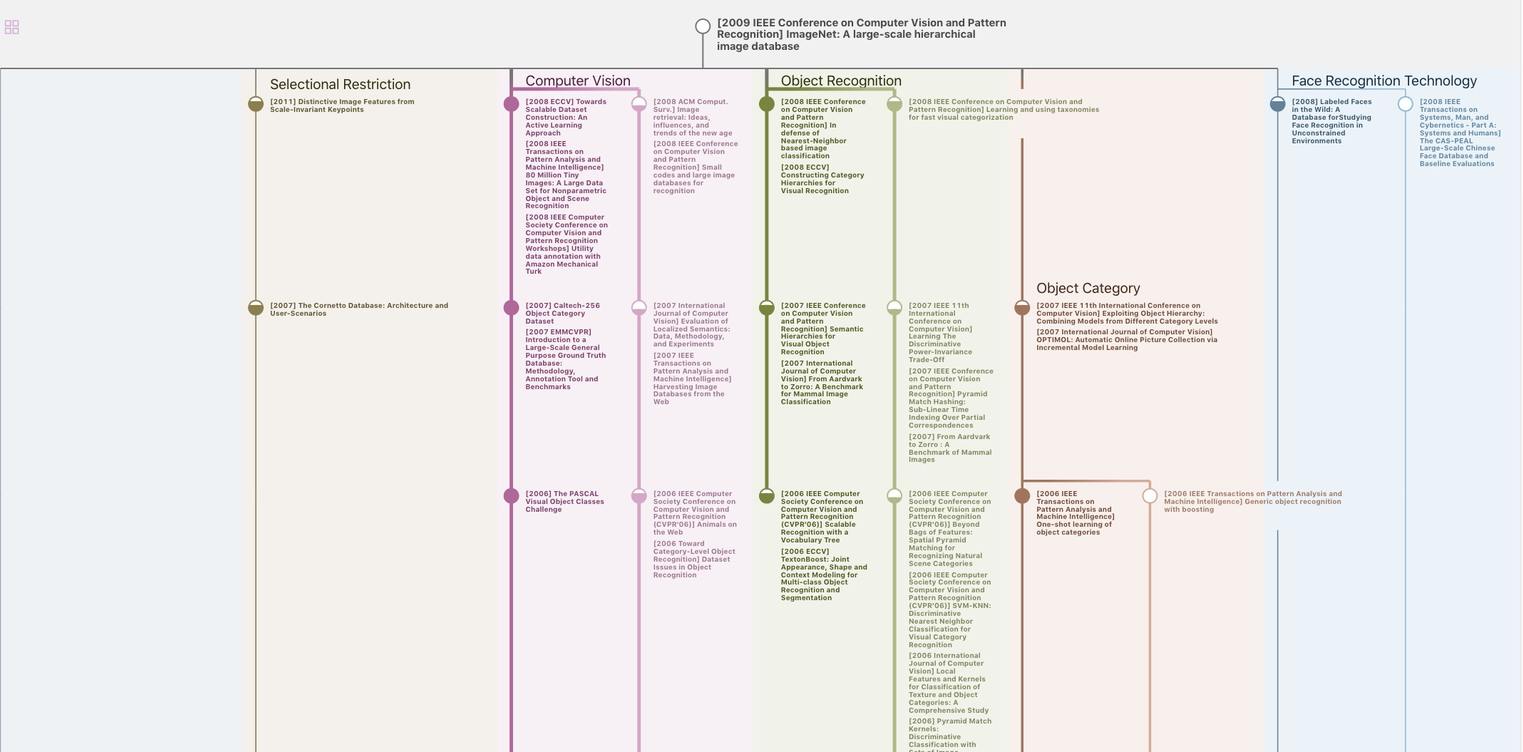
生成溯源树,研究论文发展脉络
Chat Paper
正在生成论文摘要