Learning Background-Aware Correlation Filters for Visual Tracking
2017 IEEE INTERNATIONAL CONFERENCE ON COMPUTER VISION (ICCV)(2017)
摘要
Correlation Filters (CFs) have recently demonstrated excellent performance in terms of rapidly tracking objects under challenging photometric and geometric variations. The strength of the approach comes from its ability to efficiently learn - "on the fly" - how the object is changing over time. A fundamental drawback to CFs, however, is that the background of the object is not be modelled over time which can result in suboptimal results. In this paper we propose a Background-Aware CF that can model how both the foreground and background of the object varies over time. Our approach, like conventional CFs, is extremely computationally efficient - and extensive experiments over multiple tracking benchmarks demonstrate the superior accuracy and real-time performance of our method compared to the state-of-the-art trackers including those based on a deep learning paradigm.
更多查看译文
关键词
visual tracking,geometric variations,suboptimal performance,discriminative deep features,high-end GPUs,Background-Aware CF,hand-crafted features,photometric variations,background-aware correlation filters,deep tracking frameworks,object foreground,object background
AI 理解论文
溯源树
样例
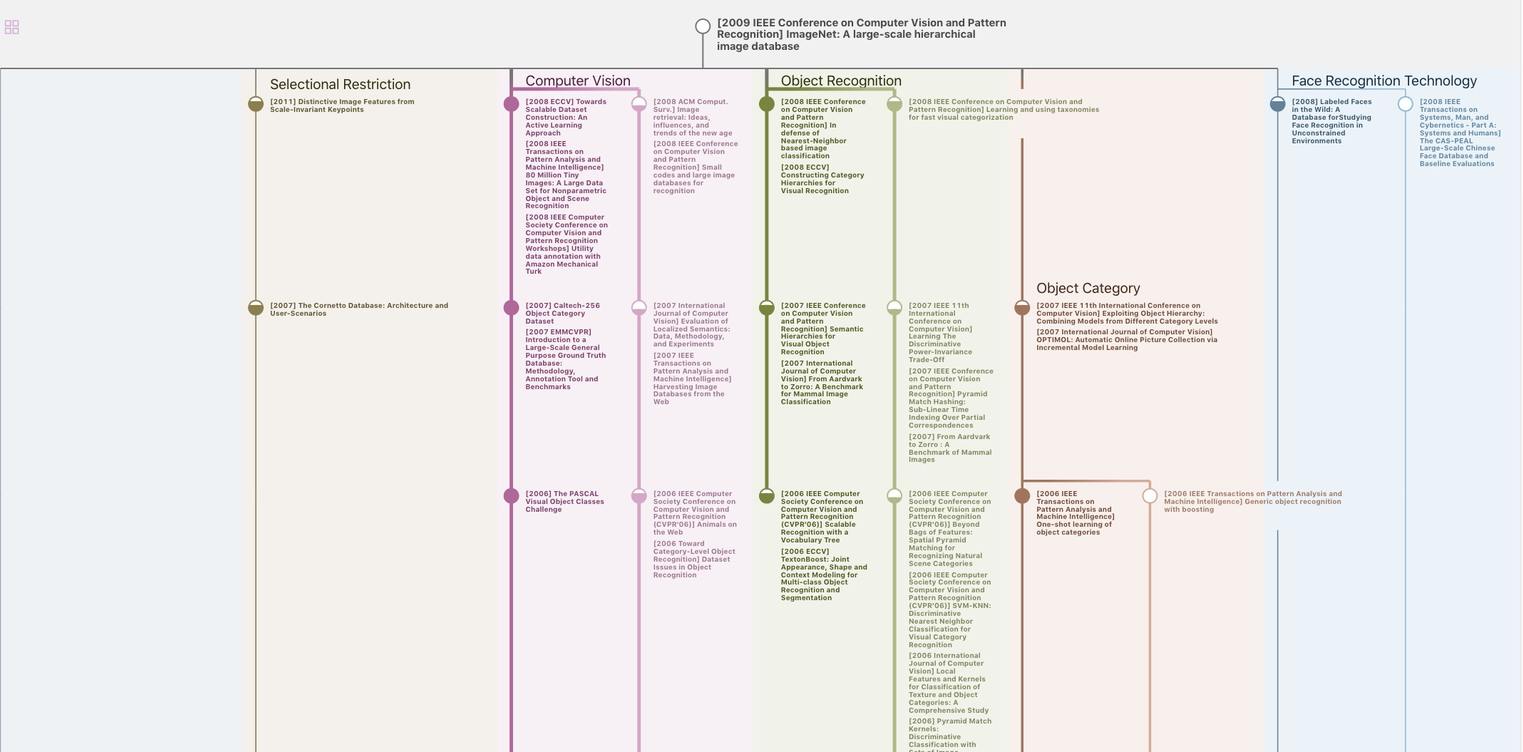
生成溯源树,研究论文发展脉络
Chat Paper
正在生成论文摘要