Learning A Recurrent Residual Fusion Network For Multimodal Matching
2017 IEEE INTERNATIONAL CONFERENCE ON COMPUTER VISION (ICCV)(2017)
摘要
A major challenge in matching between vision and language is that they typically have completely different features and representations. In this work, we introduce a novel bridge between the modality-specific representations by creating a co-embedding space based on a recurrent residual fusion (RRF) block. Specifically, RRF adapts the recurrent mechanism to residual learning, so that it can recursively improve feature embeddings while retaining the shared parameters. Then, a fusion module is used to integrate the intermediate recurrent outputs and generates a more powerful representation. In the matching network, RRF acts as a feature enhancement component to gather visual and textual representations into a more discriminative embedding space where it allows to narrow the cross-modal gap between vision and language. Moreover, we employ a bi-rank loss function to enforce separability of the two modalities in the embedding space. In the experiments, we evaluate the proposed RRF-Net using two multi-modal datasets where it achieves state-of-the-art results.
更多查看译文
关键词
recurrent residual fusion network learning,vision matching,crossmodal gap,multimodal datasets,discriminative embedding space,textual representations,visual representations,feature enhancement component,matching network,intermediate recurrent outputs,fusion module,shared parameters,feature embeddings,residual learning,recurrent mechanism,RRF,recurrent residual fusion block,co-embedding space,modality-specific representations,multimodal matching
AI 理解论文
溯源树
样例
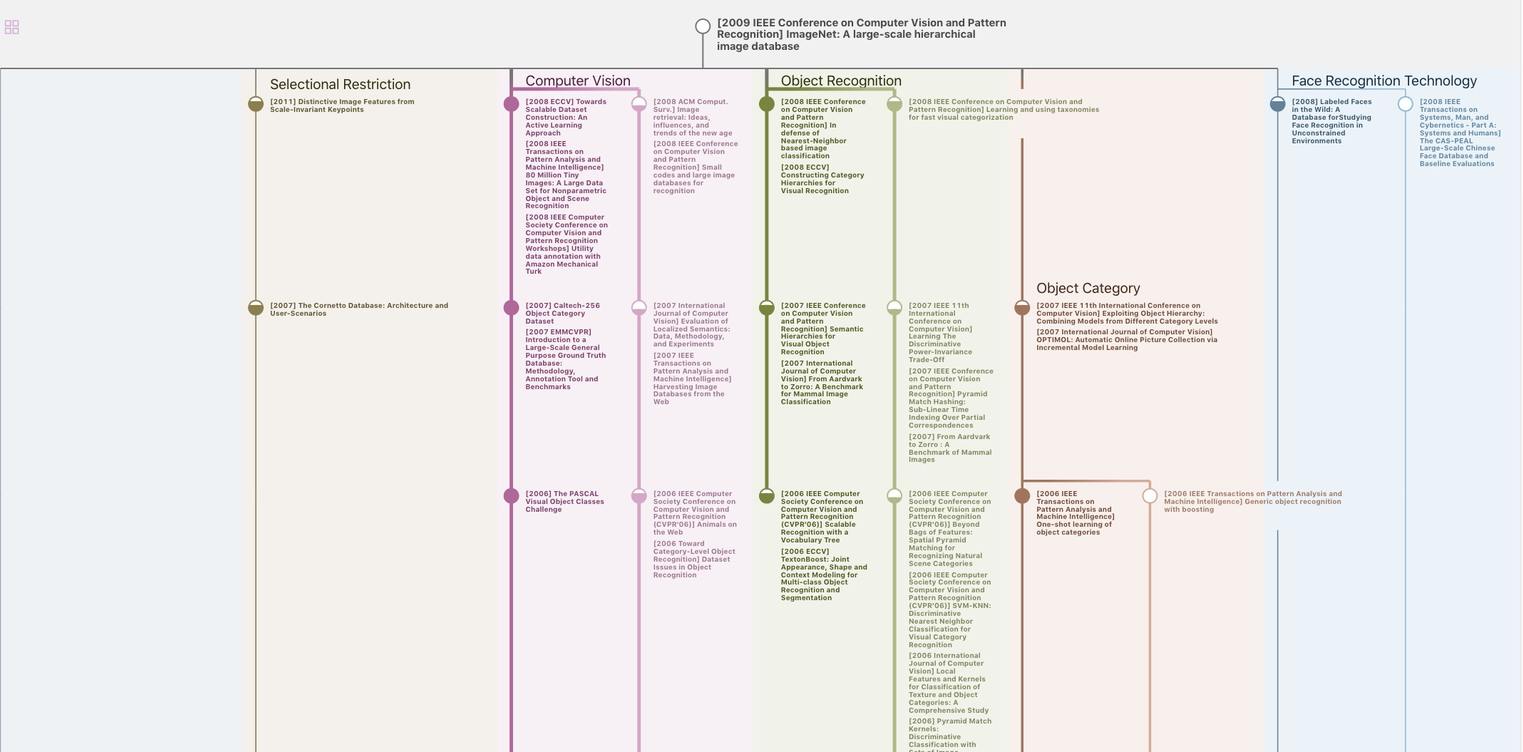
生成溯源树,研究论文发展脉络
Chat Paper
正在生成论文摘要