Optimal Weighting For Covariance Based Realization Algorithm
2017 IEEE 56TH ANNUAL CONFERENCE ON DECISION AND CONTROL (CDC)(2017)
摘要
Subspace methods are powerful tools for system identification and typically based on the projection of large dimensional data matrices, that store multivariable input/output data points, onto a low rank (extended observability) matrix. The Covariance Based Realization Algorithm (CoBRA) fortunately allows the use of finite size covariance-data matrices due to covariance preprocessing, even when a large number of data points is available. In addition, the covariance preprocessing allows CoBRA to focus on the estimation of the deterministic input/output model, without the need to estimate the state space realization of possibly high-order stochastic noise model during the projection. However, avoiding noise model estimation may negatively influence model parameter variability and this paper investigates an optimal (column) weighting matrix in CoBRA in order to improve its estimation performance during projection. An in-depth theoretical analysis is presented on the asymptotic property of the estimate when using the weighting matrix and results are formulated in terms of minimal variance of the projection error. A simple example is given to illustrate the efficiency of the proposed weighted CoBRA method.
更多查看译文
关键词
store multivariable input/output data points,low rank matrix,covariance based realization algorithm,finite size covariance-data matrices,covariance preprocessing,deterministic input/output model,state space realization,noise model estimation,model parameter variability,optimal weighting matrix,estimation performance,weighted CoBRA method,dimensional data matrices,optimal weighting,system identification,large dimensional data matrices,high-order stochastic noise model
AI 理解论文
溯源树
样例
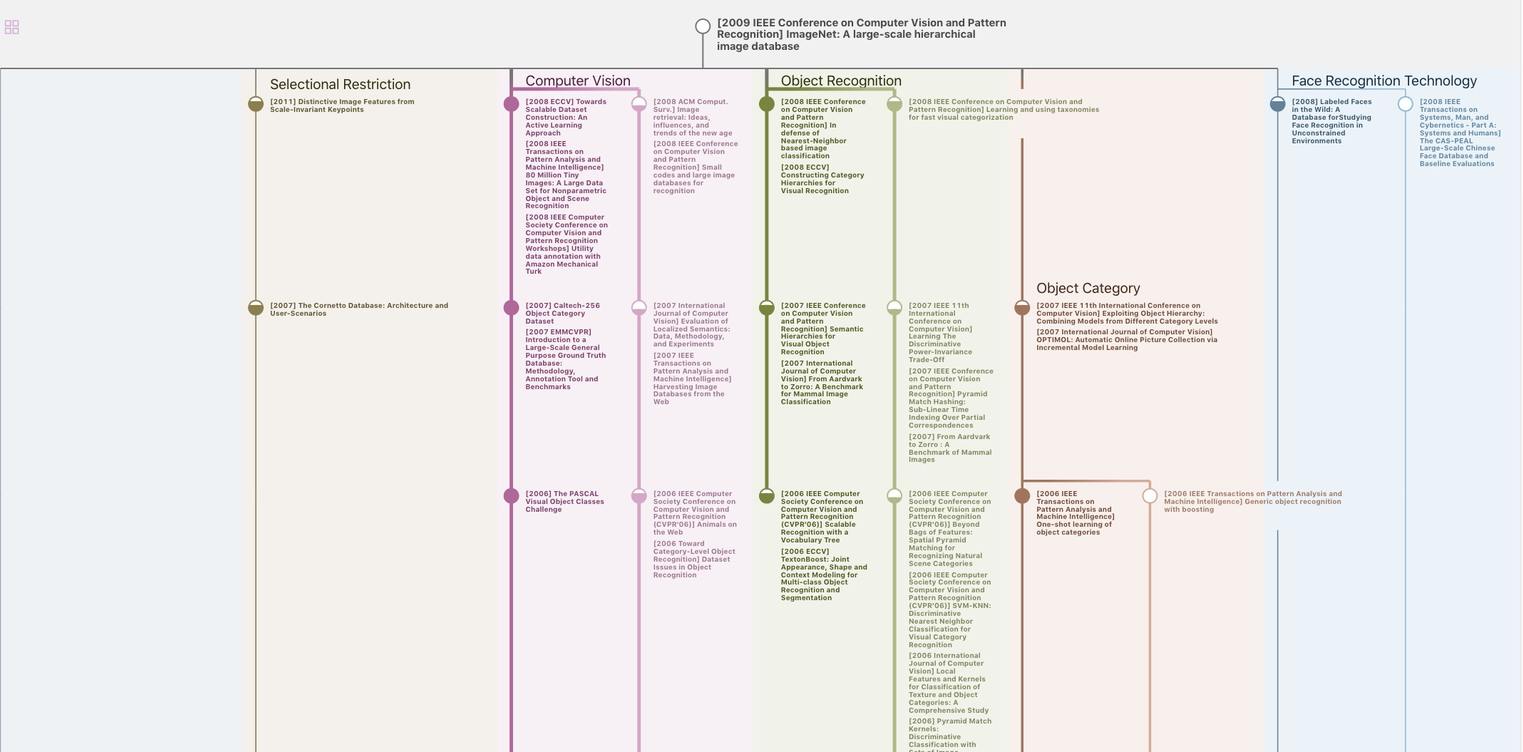
生成溯源树,研究论文发展脉络
Chat Paper
正在生成论文摘要