Combining heterogeneous features for time series prediction
2017 International Conference on Behavioral, Economic, Socio-cultural Computing (BESC)(2017)
摘要
Time series prediction is a challenging task in reality, and various methods have been proposed for it. However, only the historical series of values are exploited in most of existing methods. Therefore, the predictive models might be not effective in some cases, due to: (1) the historical series of values is not sufficient usually, and (2) features from heterogeneous sources such as the intrinsic features of data samples themselves, which could be very useful, are not take into consideration. To address these issues, we proposed a novel method in this paper which learns the predictive model based on the combination of dynamic features extracted from series of historical values and static features of data samples. To evaluate the performance of our proposed method, we compare it with linear regression and boosted trees, and the experimental results validate our method's superiority.
更多查看译文
关键词
predictive model,dynamic features,historical values,static features,data samples,time series prediction,historical series,heterogeneous sources,intrinsic features
AI 理解论文
溯源树
样例
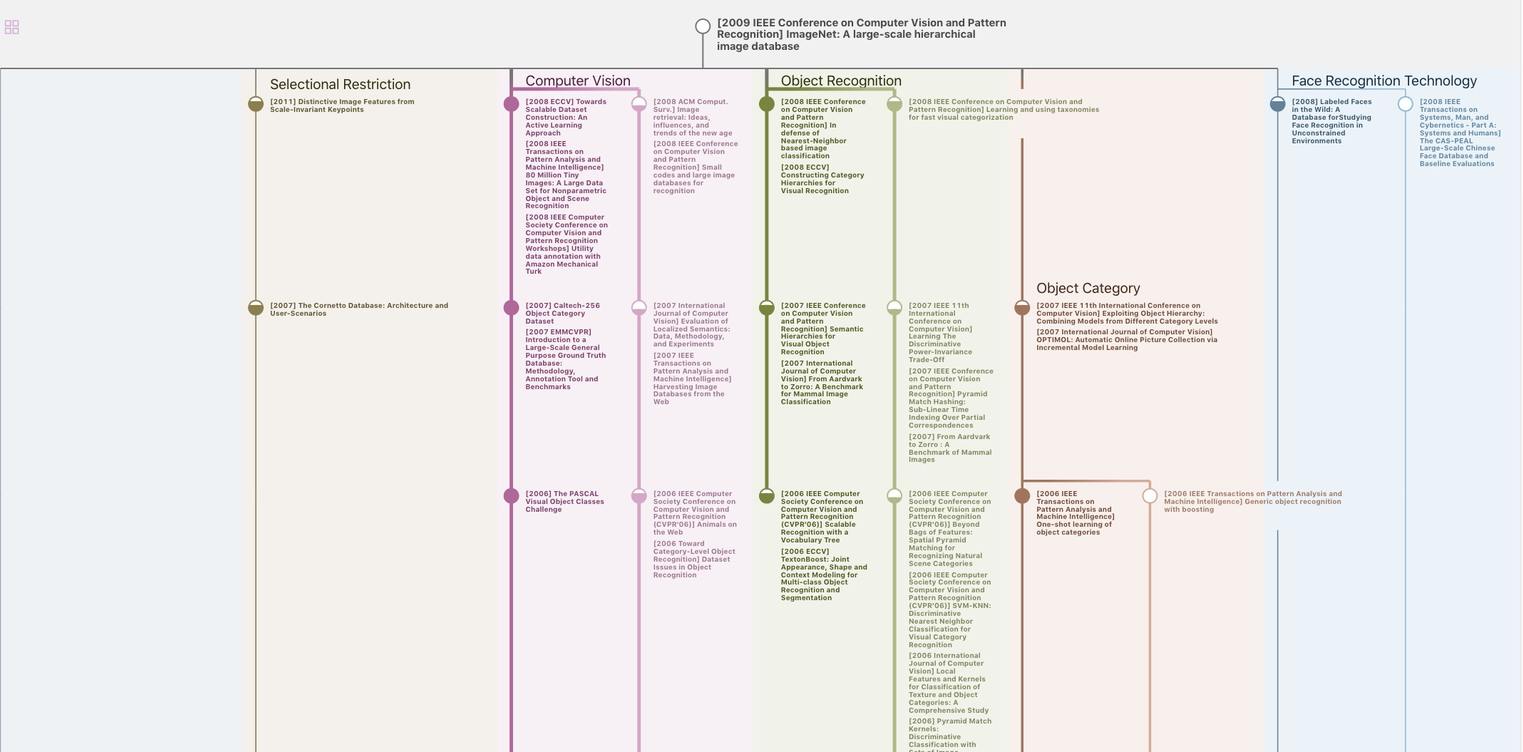
生成溯源树,研究论文发展脉络
Chat Paper
正在生成论文摘要