Computing Based on Material Training: Application to Binary Classification Problems
2017 IEEE International Conference on Rebooting Computing (ICRC)(2017)
摘要
Evolution-in-materio is a form of unconventional computing combining materials' training and evolutionary search algorithms. In previous work, a mixture of single-walled-carbon-nanotubes (SWCNTs) dispersed in a liquid crystal (LC) was trained so that its morphology and electrical properties were gradually changed to perform a computational task. Material-based computation is treated as an optimisation problem with a hybrid search space consisting of the voltages used for creating the electrical field and the material's infinitely possible SWCNT arrangements in LC. In this paper, we study solutions using synthetic data with a non-linear separating boundary. In addition, results for two real life datasets with partly merged classes are presented. The training process is based on a differential evolution (DE) algorithm, which subjects the SWCNT/LC material to repeated electrical charging, leading to progressive morphological and electric conductivity modifications. It is shown that the material configuration the DE algorithm converges to form a non-negligible part of the solution. Furthermore, the problem's complexity is relevant to the properties of the resulting "physical solver". The material structures created when training for a problem allow the retraining for a less complex one. The result is a doubly-trained material that keeps the memory of the original more complex problem. This is not the case for doubly-trained materials where initial training is for the less complex problem. The optimal electric field found by the DE algorithm is also a necessary solution component for the material's output to be interpreted as a computation.
更多查看译文
关键词
material structures,doubly-trained materials,optimal electric field,DE algorithm,material training,binary classification problems,evolution-in-materio,evolutionary search algorithms,single-walled-carbon-nanotubes,liquid crystal,morphology,electrical properties,material-based computation,optimisation problem,hybrid search space,electrical field,infinitely possible SWCNT arrangements,SWCNT/LC material,repeated electrical charging,electric conductivity modifications,material configuration
AI 理解论文
溯源树
样例
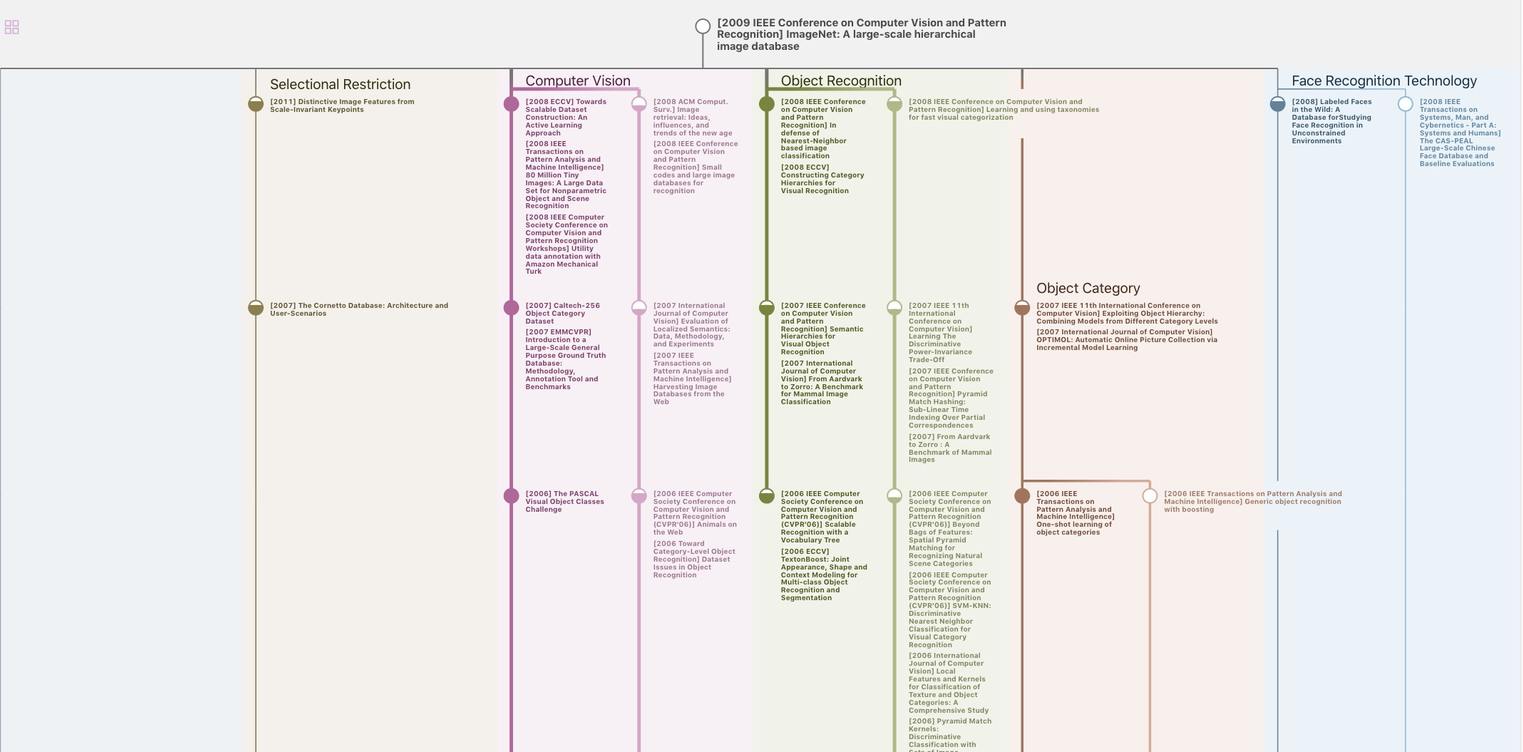
生成溯源树,研究论文发展脉络
Chat Paper
正在生成论文摘要