Approximate Positive Correlated Distributions and Approximation Algorithms for D-optimal Design.
SODA '18: Symposium on Discrete Algorithms New Orleans Louisiana January, 2018(2018)
摘要
Experimental design is a classical area in statistics [21] and has also found new applications in machine learning[2]. In the combinatorial experimental design problem, the aim is to estimate an unknown m-dimensional vector x from linear measurements where a Gaussian noise is introduced in each measurement. The goal is to pick k out of the given n experiments so as to make the most accurate estimate of the unknown parameter x. Given a set S of chosen experiments, the most likelihood estimate x' can be obtained by a least squares computation. One of the robust measures of error estimation is the D-optimality criterion [27] which aims to minimize the generalized variance of the estimator. This corresponds to minimizing the volume of the standard confidence ellipsoid for the estimation error x − x'. The problem gives rise to two natural variants depending on whether repetitions of experiments is allowed or not. The latter variant, while being more general, has also found applications in geographical location of sensors [19].
We show a close connection between approximation algorithms for the D-optimal design problem and constructions of approximately m-wise positively correlated distributions. This connection allows us to obtain a [EQUATION]-approximation for the D-optimal design problem with and without repetitions giving the first constant factor approximation for the problem. We then consider the case when the number of experiments chosen is much larger than the dimension m and show one can obtain (1 − ϵ)-approximation if [EQUATION] when repetitions are allowed and if [EQUATION] when no repetitions are allowed improving on previous work.
更多查看译文
AI 理解论文
溯源树
样例
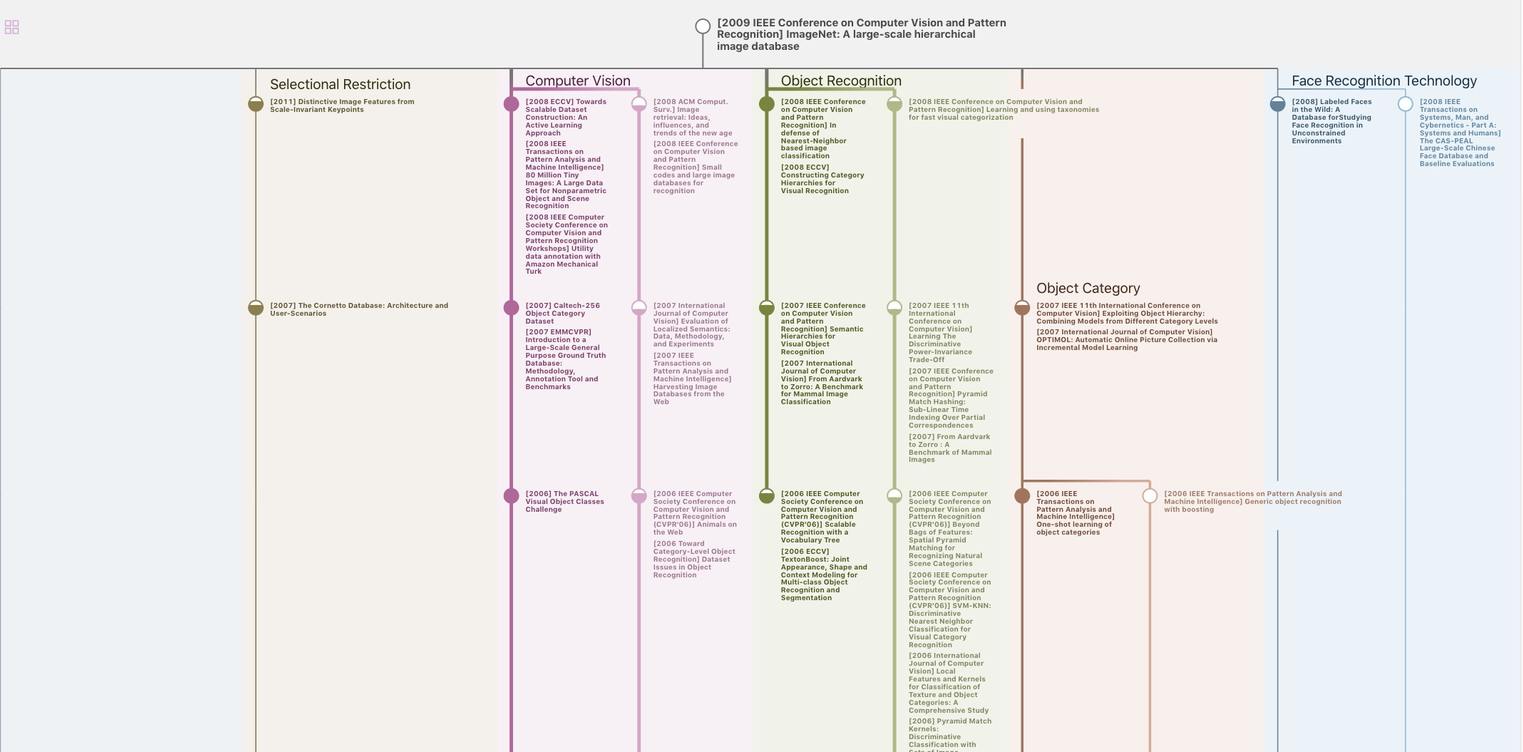
生成溯源树,研究论文发展脉络
Chat Paper
正在生成论文摘要