Compressed Time Delay Neural Network For Small-Footprint Keyword Spotting
18TH ANNUAL CONFERENCE OF THE INTERNATIONAL SPEECH COMMUNICATION ASSOCIATION (INTERSPEECH 2017), VOLS 1-6: SITUATED INTERACTION(2017)
摘要
In this paper we investigate a time delay neural network (TDNN) for a keyword spotting task that requires low CPU, memory and latency. The TDNN is trained with transfer learning and multi-task learning. Temporal subsampling enabled by the time delay architecture reduces computational complexity. We propose to apply singular value decomposition (SVD) to further reduce TDNN complexity. This allows us to first train a larger full-rank TDNN model which is not limited byCPU/memory constraints. The larger TDNN usually achieves better performance. Afterwards, its size can be compressed by SVD to meet the budget requirements. Hidden Markov models (HMM) are used in conjunction with the networks to perform keyword detection and performance is measured in terms of area under the curve (AUC) for detection error tradeoff (DET) curves. Our experimental results on a large in-house far-field corpus show that the full-rank TDNN achieves a 19.7% DET AUC reduction compared to a similar-size deep neural network (DNN) baseline. If we train a larger size full-rank TDNN first and then reduce it via SVD to the comparable size of the DNN, we obtain a 37.6% reduction in DET AUC compared to the DNN baseline.
更多查看译文
关键词
keyword spotting, time delay neural network, singular value decomposition, small-footprint
AI 理解论文
溯源树
样例
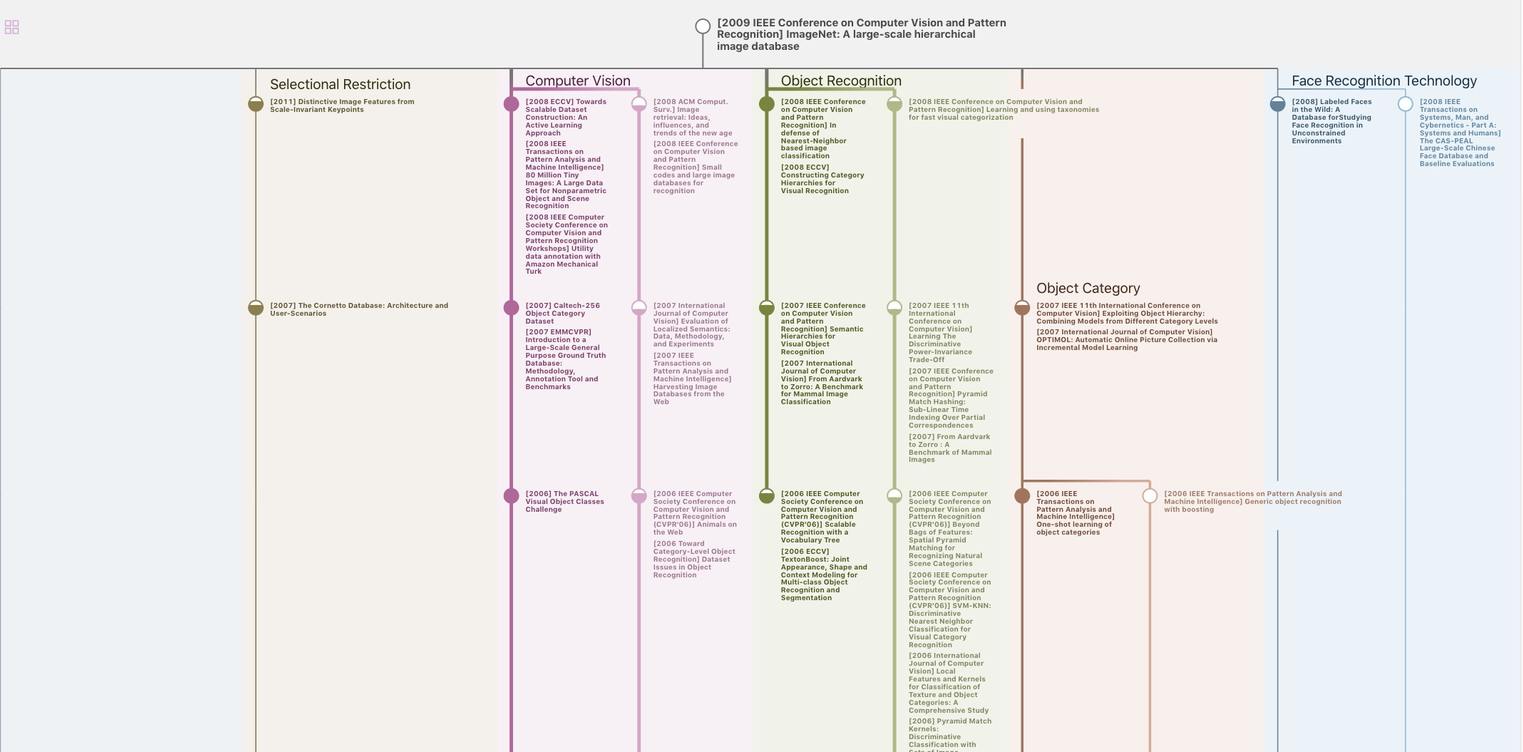
生成溯源树,研究论文发展脉络
Chat Paper
正在生成论文摘要