Gaussian Prediction Based Attention For Online End-To-End Speech Recognition
18TH ANNUAL CONFERENCE OF THE INTERNATIONAL SPEECH COMMUNICATION ASSOCIATION (INTERSPEECH 2017), VOLS 1-6: SITUATED INTERACTION(2017)
摘要
Recently end-to-end speech recognition has obtained much attention. One of the popular models to achieve end-to-end speech recognition is attention based encoder-decoder model, which usually generating output sequences iteratively by attending the whole representations of the input sequences. However. predicting outputs until receiving the whole input sequence is not practical for online or low time latency speech recognition. In this paper, we present a simple but effective attention mechanism which can make the encoder-decoder model generate outputs without attending the entire input sequence and can apply to online speech recognition. At each prediction step, the attention is assumed to be a time-moving gaussian window with variable size and can be predicted by using previous input and output information instead of the content based computation on the whole input sequence. To further improve the online performance of the model, we employ deep convolutional neural networks as encoder. Experiments show that the gaussian prediction based attention works well and under the help of deep convolutional neural networks the online model achieves 19.5% phoneme error rate in TIMIT ASR task.
更多查看译文
关键词
Automatic Speech Recognition, Encoder-Decoder, Online, Gaussian Prediction based Attention, Deep Convolutional Encoder
AI 理解论文
溯源树
样例
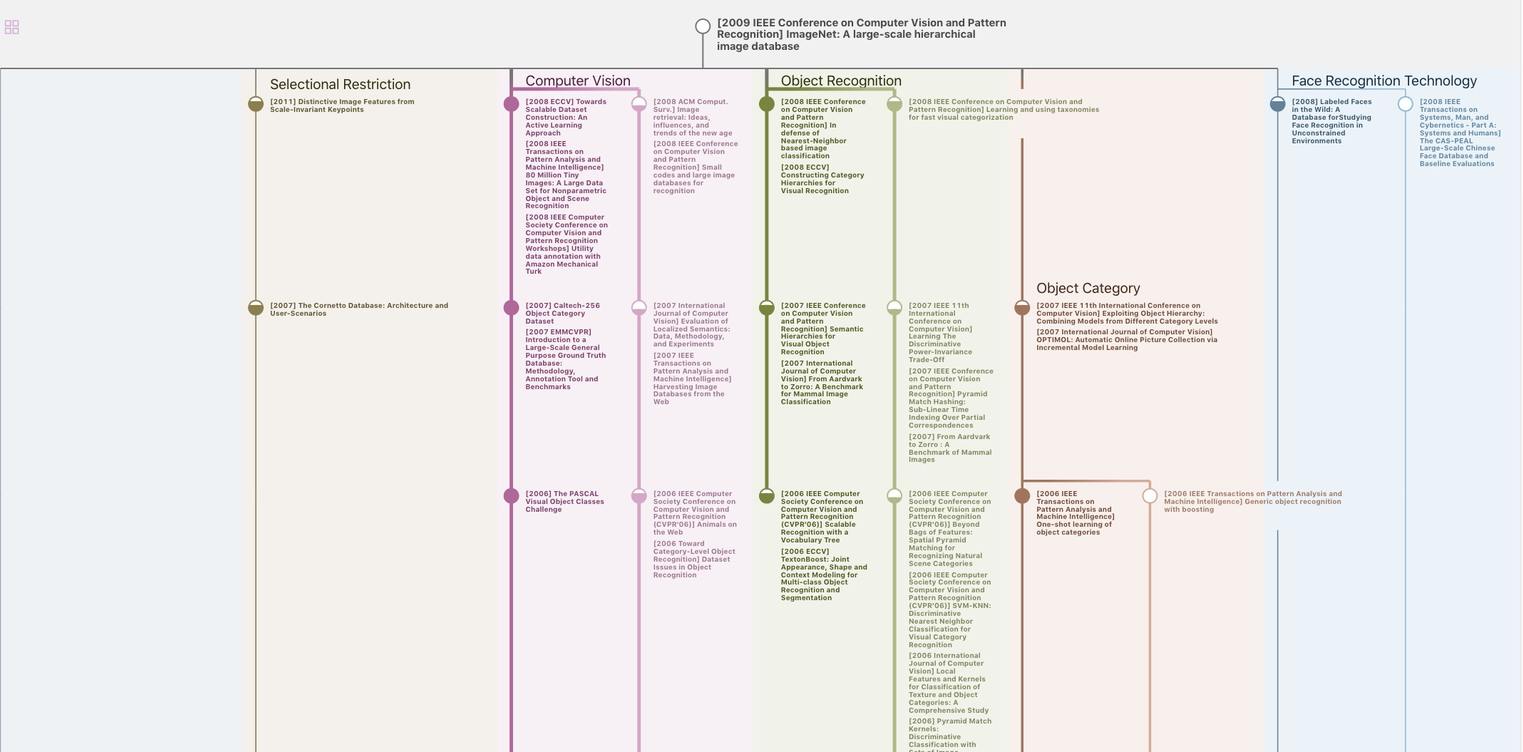
生成溯源树,研究论文发展脉络
Chat Paper
正在生成论文摘要