Learning Factorized Transforms For Unsupervised Adaptation Of Lstm-Rnn Acoustic Models
18TH ANNUAL CONFERENCE OF THE INTERNATIONAL SPEECH COMMUNICATION ASSOCIATION (INTERSPEECH 2017), VOLS 1-6: SITUATED INTERACTION(2017)
摘要
Factorized Hidden Layer (FHL) adaptation has been proposed for speaker adaptation of deep neural network (DNN) based acoustic models. In FHL adaptation, a speaker-dependent (SD) transformation matrix and an SD bias are included in addition to the standard affine transformation. The SD transformation is a linear combination of rank-1 matrices whereas the SD bias is a linear combination of vectors. Recently. the Long Short Term Memory (LSTM) Recurrent Neural Networks (RNNs) have shown to outperform DNN acoustic models in many Automatic Speech Recognition (ASR) tasks. In this work, we investigate the effectiveness of SD transformations for LSTM-RNN acoustic models. Experimental results show that when combined with scaling of LSTM cell states' outputs, SD transformations achieve 2.3% and 2.1% absolute improvements over the baseline LSTM systems for the AMI IHM and AMI SDM tasks respectively.
更多查看译文
关键词
Long Short-Term memory (LSTM), Recurrent Neural Networks (RNNs), Speaker Adaptation, Acoustic Modeling
AI 理解论文
溯源树
样例
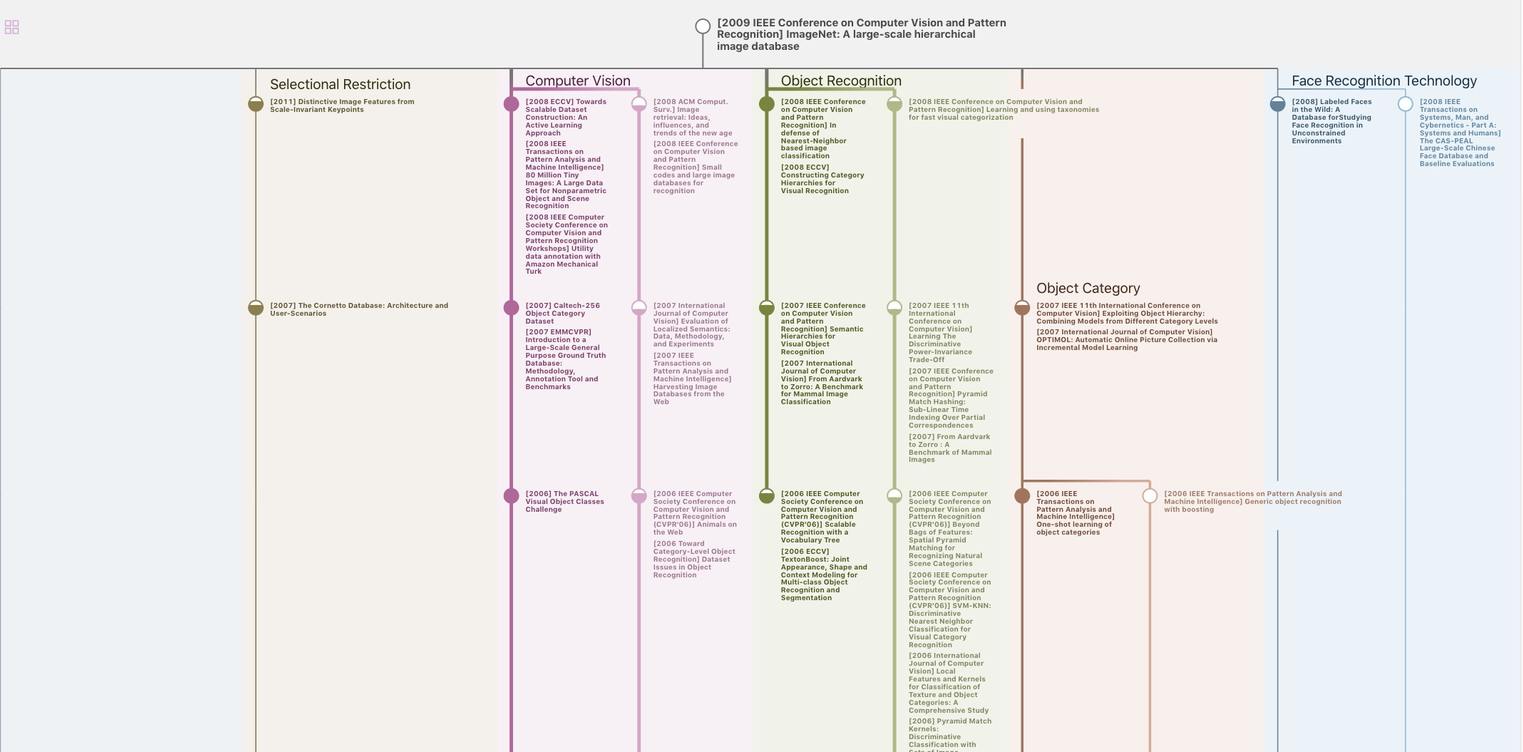
生成溯源树,研究论文发展脉络
Chat Paper
正在生成论文摘要