On Multi-Domain Training And Adaptation Of End-To-End Rnn Acoustic Models For Distant Speech Recognition
18TH ANNUAL CONFERENCE OF THE INTERNATIONAL SPEECH COMMUNICATION ASSOCIATION (INTERSPEECH 2017), VOLS 1-6: SITUATED INTERACTION(2017)
摘要
Recognition of distant (far-field) speech is a challenge for ASR due to mismatch in recording conditions resulting from room reverberation and environment noise. Given the remarkable learning capacity of deep neural networks, there is increasing interest to address this problem by using a large corpus of reverberant far-field speech to train robust models. In this study. we explore how an end-to-end RNN acoustic model trained on speech from different rooms and acoustic conditions (different domains) achieves robustness to environmental variations. It is shown that the first hidden layer acts as a domain separator, projecting the data from different domains into different sub-spaces. The subsequent layers then use this encoded domain knowledge to map these features to final representations that are invariant to domain change. This mechanism is closely related to noise-aware or room-aware approaches which append manually-extracted domain signatures to the input features. Additionaly, we demonstrate how this understanding of the learning procedure provides useful guidance for model adaptation to new acoustic conditions. We present results based on AMI corpus to demonstrate the propagation of domain information in a deep RNN, and perform recognition experiments which indicate the role of encoded domain knowledge on training and adaptation of RNN acoustic models.
更多查看译文
关键词
distant speech recognition, recurrent neural network, multi-domain training
AI 理解论文
溯源树
样例
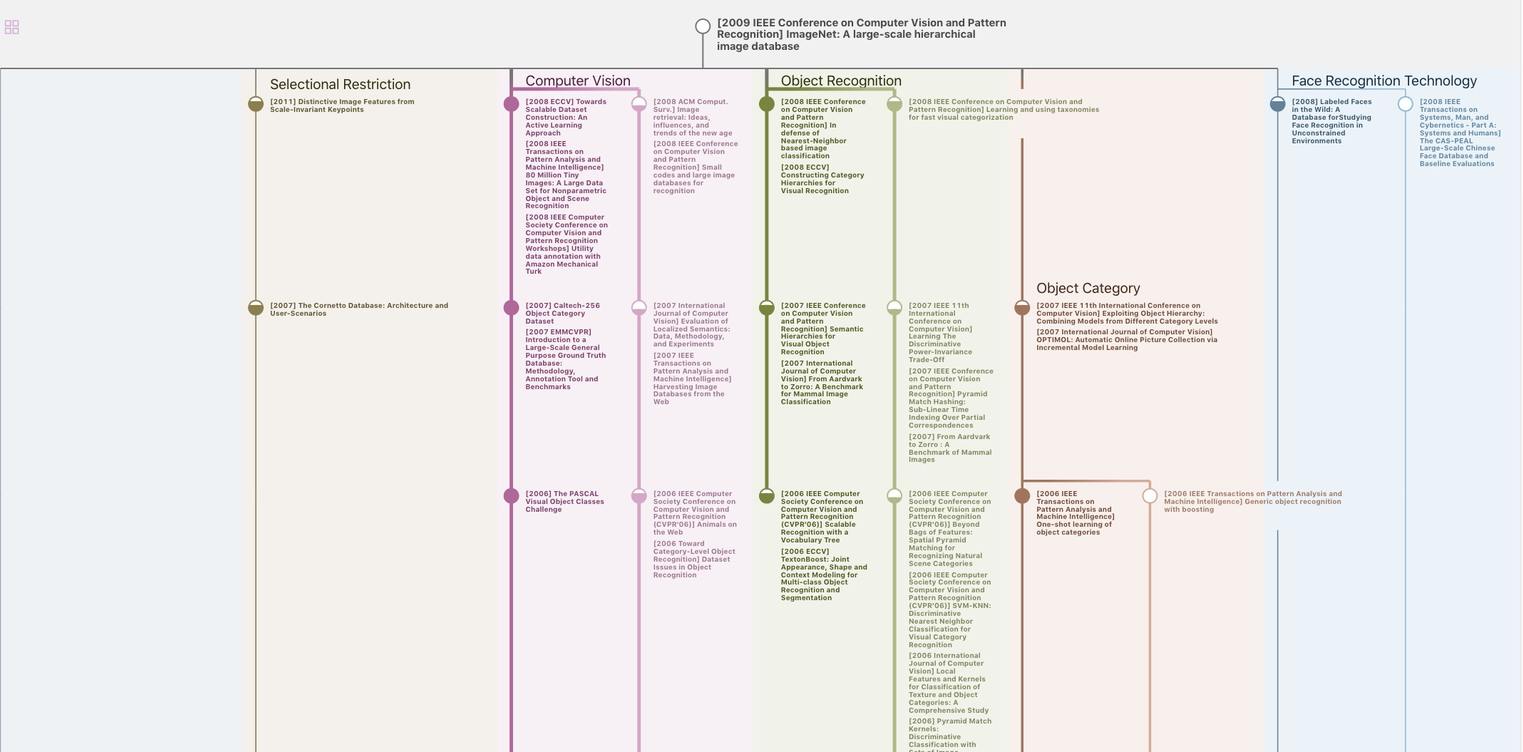
生成溯源树,研究论文发展脉络
Chat Paper
正在生成论文摘要