Analysis Of Score Normalization In Multilingual Speaker Recognition
18TH ANNUAL CONFERENCE OF THE INTERNATIONAL SPEECH COMMUNICATION ASSOCIATION (INTERSPEECH 2017), VOLS 1-6: SITUATED INTERACTION(2017)
摘要
NIST Speaker Recognition Evaluation 2016 has revealed the importance of score normalization for mismatched data conditions. This paper analyzes several score normalization techniques for test conditions with multiple languages. The best performing one for a PLDA classifier is an adaptive s-norm with 30% relative improvement over the system without any score normalization. The analysis shows that the adaptive score normalization (using top scoring files per trial) selects cohorts that in 68% contain recordings from the same language and in 92% of the same gender as the enrollment and test recordings. Our results suggest that the data to select score normalization cohorts should be a pool of several languages and channels and if possible, its subset should contain data from the target domain.
更多查看译文
关键词
speaker recognition, score normalization
AI 理解论文
溯源树
样例
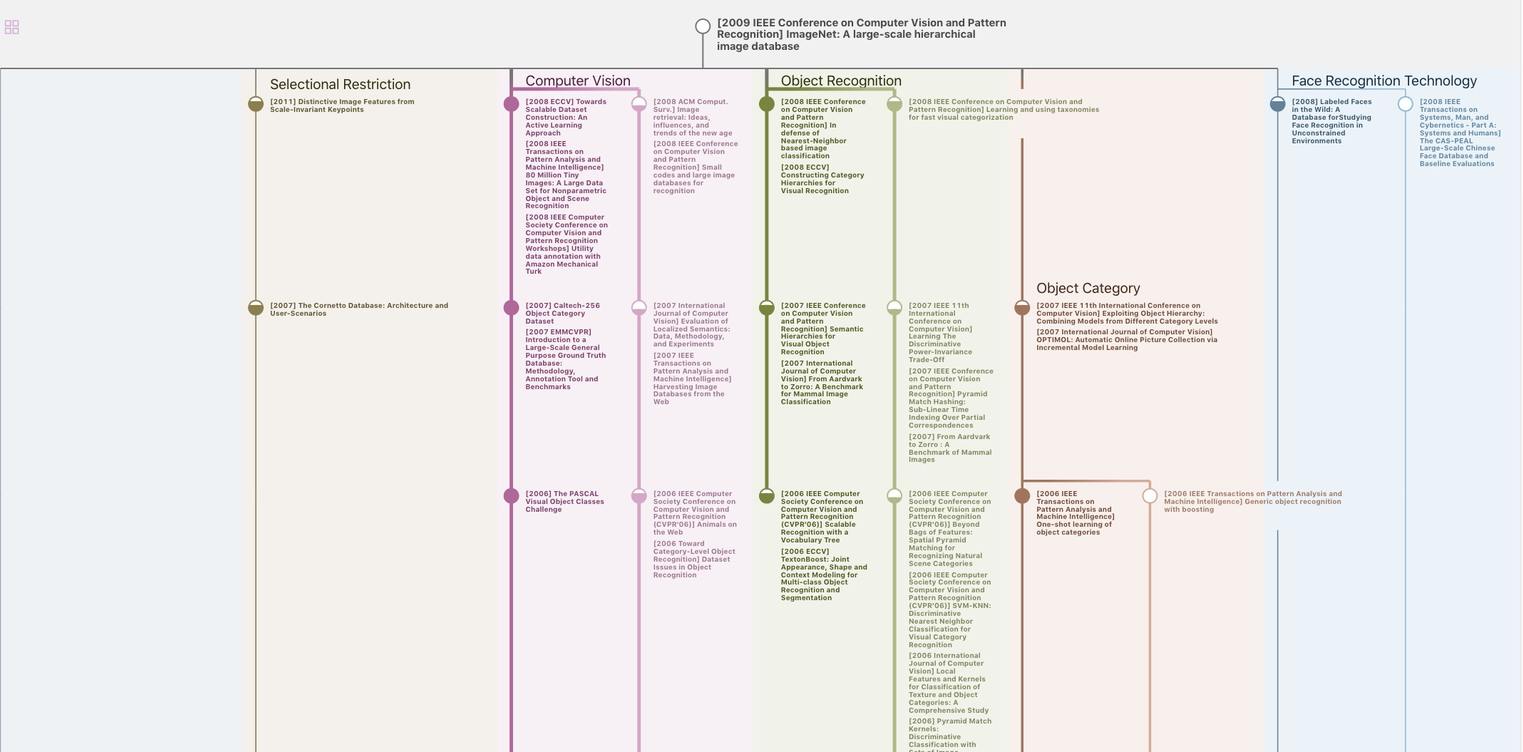
生成溯源树,研究论文发展脉络
Chat Paper
正在生成论文摘要