Low-Shot Face Recognition With Hybrid Classifiers
2017 IEEE INTERNATIONAL CONFERENCE ON COMPUTER VISION WORKSHOPS (ICCVW 2017)(2017)
摘要
In this paper, we present our solution to the MS-Celeb-1M Low-shot Face Recognition Challenge. This challenge aims to recognize 21,000 celebrities, in which 20,000 celebrities (Base Set) come with 50-100 images per person for training. But only one training image is provided for each person in the rest 1,000 celebrities (Novel Set). Given the dispersion in the number of training samples between Base Set and Novel Set, it is hard to build a single classifier that works well for both sets. To solve this problem, we propose a framework with multiple classifiers. This decomposes a single classifier for all data into multiple classifiers that each works well for a part of data. To be more specific, a Deep Convolution Neural Network (CNN) is utilized for Base Set and a Nearest Neighbor (NN) model is applied to Novel Set. The final prediction is based on a fusion of CNN results and NN results. Extensive experiments on MS-Celeb-1M Low-shot face dataset demonstrate the superiority of the proposed method. Our solution achieves 92.64% Coverage@Precision= 0.99 in Novel Set while maintaining 99.58% top-1 accuracy in Base Set. This result wins the challenge in the track of without external data. Moreover, it is worth to note our result even surpasses some models using external data and can achieve the third place if compared with all participants.
更多查看译文
关键词
hybrid classifiers,MS-Celeb-1M Low-shot Face Recognition Challenge,celebrity recognition,Deep Convolution Neural Network,Nearest Neighbor model,face dataset,Base Set,training samples,training image
AI 理解论文
溯源树
样例
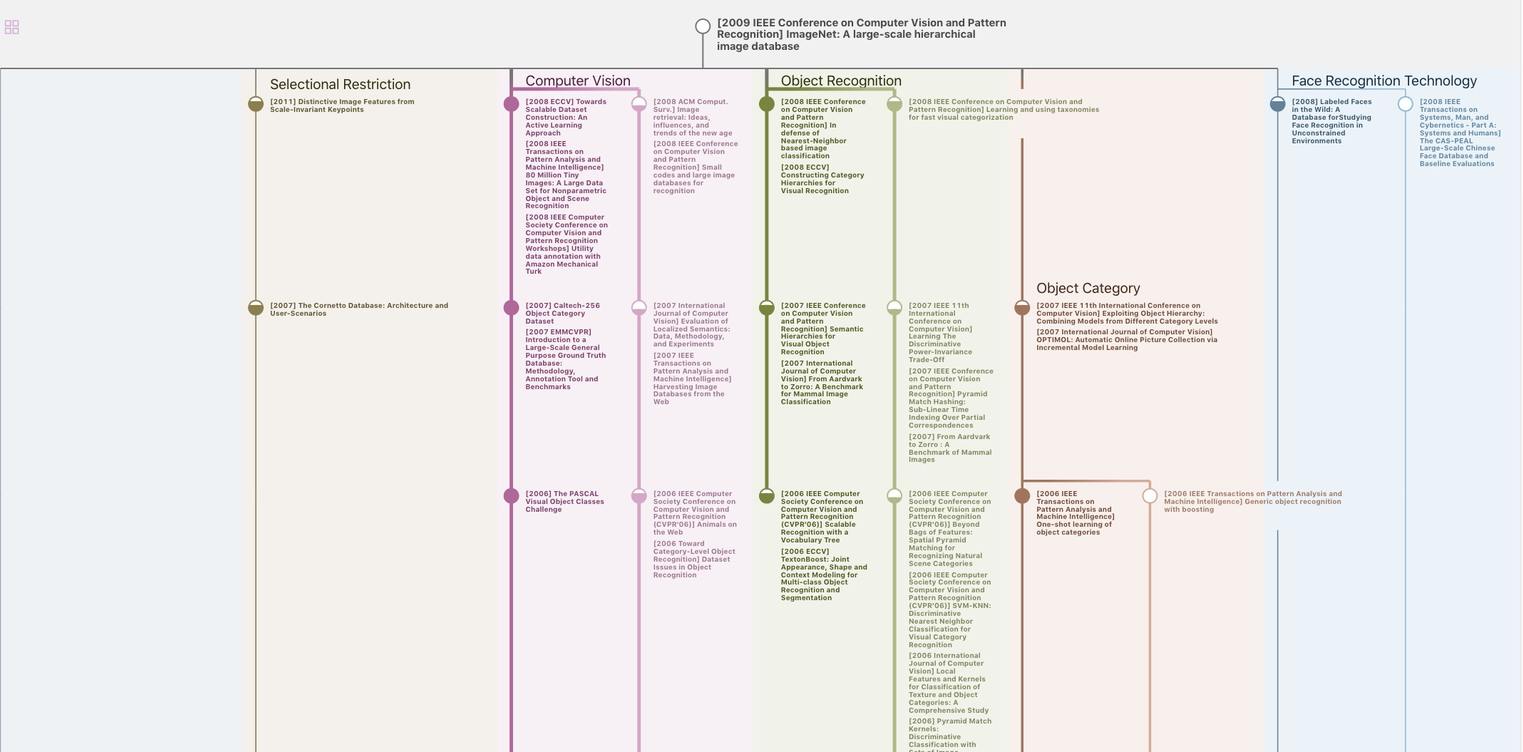
生成溯源树,研究论文发展脉络
Chat Paper
正在生成论文摘要