A Data-Driven Approach To Localization For High Frequency Wireless Mobile Networks
GLOBECOM 2017 - 2017 IEEE GLOBAL COMMUNICATIONS CONFERENCE(2017)
摘要
The use of high frequency millimeter wave (mmWave) bands at 28 GHz or higher will be a defining characteristic of next-generation wireless networks, such as 5G and 802.11ad networks. However, communicating over these high frequencies bands often requires directional antennas on base stations to dynamically align their beams with mobile nodes. To quickly align antennas, we propose a data-driven deep neural network (DNN) approach to localize mobile nodes using lower frequency spectrum. Our methods require fewer than 30 real-world sample locations to learn a model that can localize a mobile node to the required 5G indoor sub-meter accuracy. We demonstrate with real-world data in indoor and outdoor experiments that this performance is achievable, even in multipath-rich environments. Further, we show via simulation that the proposed DNN approach is robust to noise and collinearity between antenna arrays. Our primary contributions are: (1) a novel structure for a deep neural network that reflects the impact base station location has on node localization, (2) a quantized loss function for neural network training that improves localization accuracy and reduces the amount of training data needed, and (3) a procedure for using synthetic data to reduce the required number of real-world measurements needed for training the data-driven localization model. Our real-world experiments show that the use of synthetic data can improve localization accuracy by over 3x.
更多查看译文
关键词
millimeter waves,5G,localization,mobile networks,machine learning,synthetic data for training
AI 理解论文
溯源树
样例
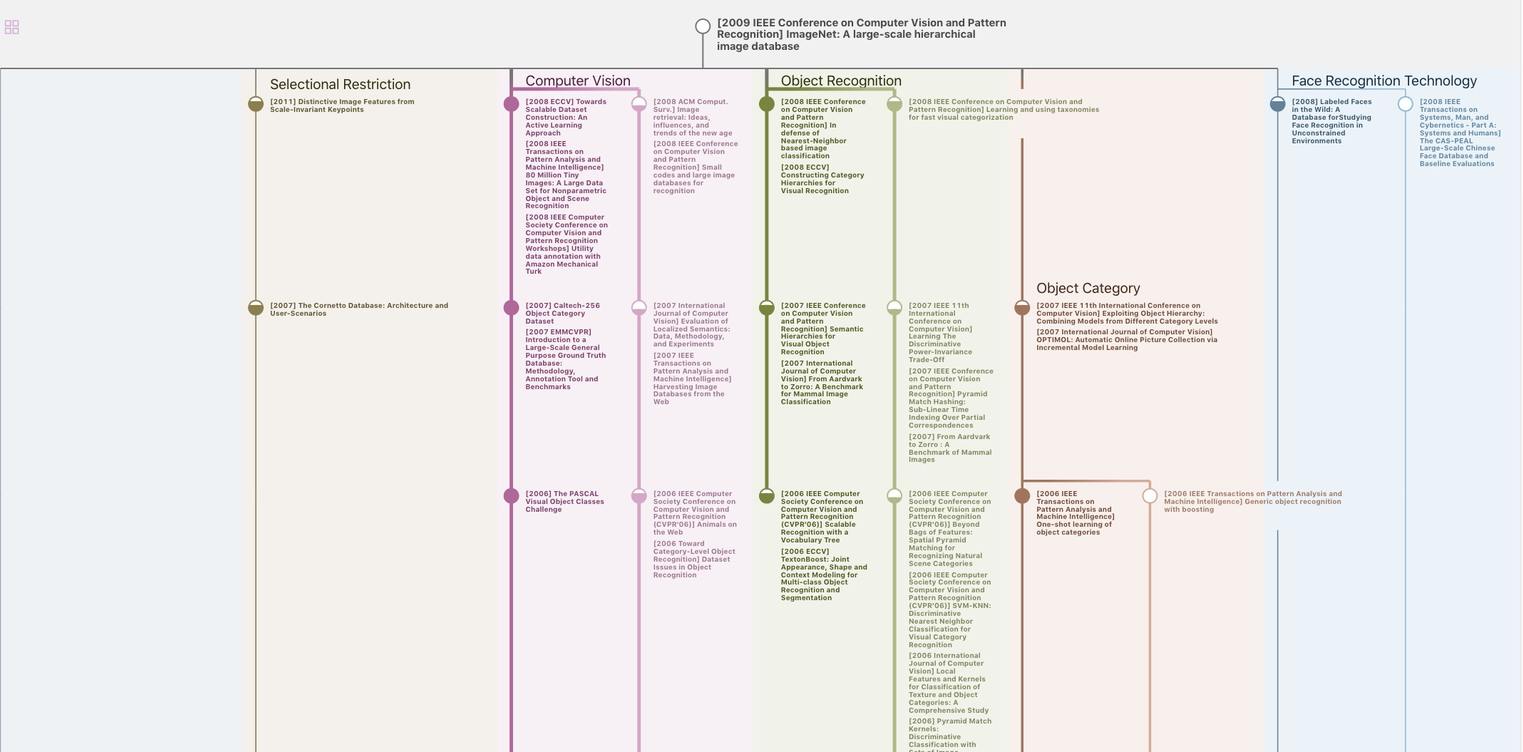
生成溯源树,研究论文发展脉络
Chat Paper
正在生成论文摘要