Does mitigating ML's disparate impact require disparate treatment?
arXiv: Machine Learning(2017)
摘要
Following related work in law and policy, two notions of prejudice have come to shape the study of fairness in algorithmic decision-making. Algorithms exhibit disparate treatment if they formally treat people differently according to a protected characteristic, like race, or if they intentionally discriminate (even if via proxy variables). Algorithms exhibit disparate impact if they affect subgroups differently. Disparate impact can arise unintentionally and absent disparate treatment. The natural way to reduce disparate impact would be to apply disparate treatment in favor of the disadvantaged group, i.e. to apply affirmative action. However, owing to the practiceu0027s contested legal status, several papers have proposed trying to eliminate both forms of unfairness simultaneously, introducing a family of algorithms that we denote disparate learning processes (DLPs). These processes incorporate the protected characteristic as an input to the learning algorithm (e.g.~via a regularizer) but produce a model that cannot directly access the protected characteristic as an input. In this paper, we make the following arguments: (i) DLPs can be functionally equivalent to disparate treatment, and thus should carry the same legal status; (ii) when the protected characteristic is redundantly encoded in the nonsensitive features, DLPs can exactly apply any disparate treatment protocol; (iii) when the characteristic is only partially encoded, DLPs may induce within-class discrimination. Finally, we argue the normative point that rather than masking efforts towards proportional representation, it is preferable to undertake them transparently.
更多查看译文
AI 理解论文
溯源树
样例
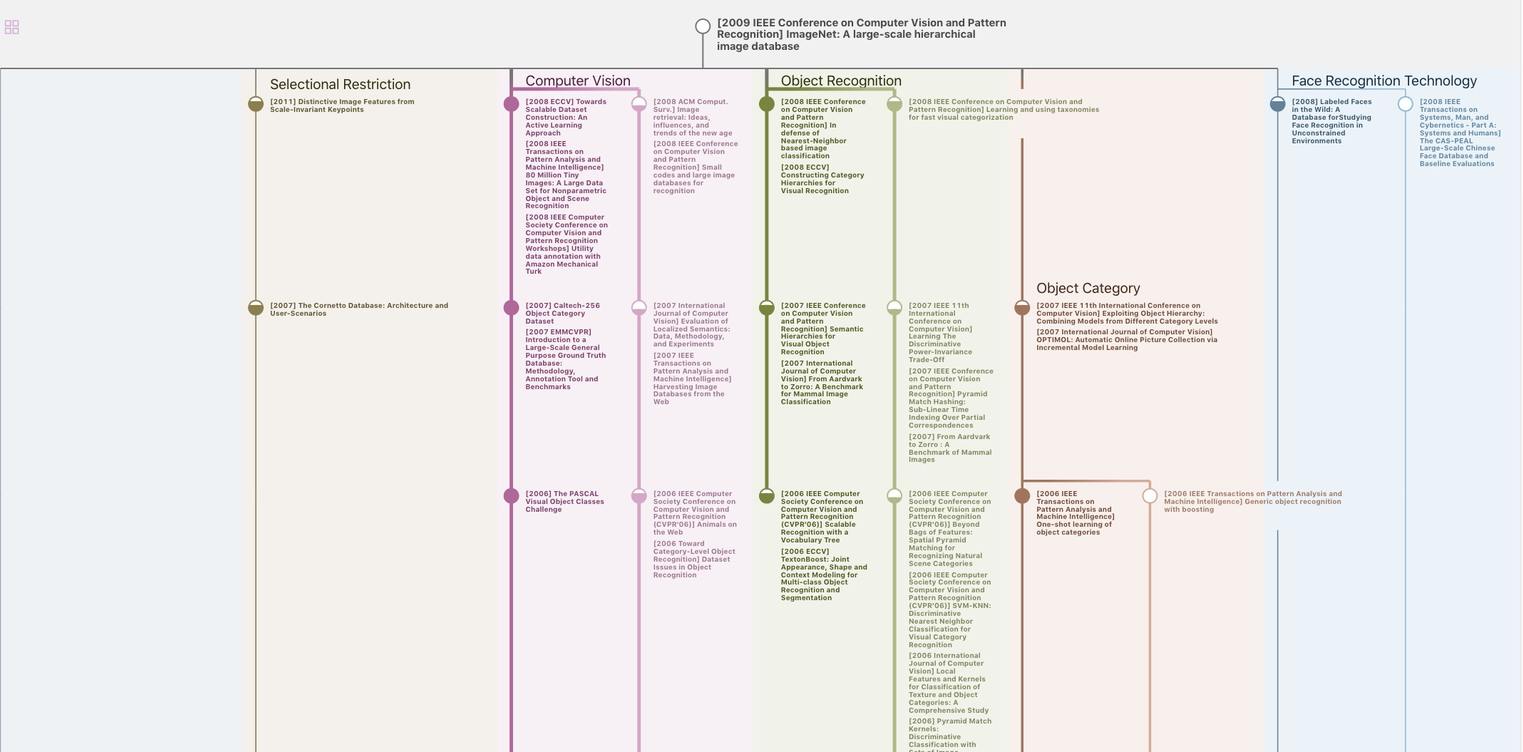
生成溯源树,研究论文发展脉络
Chat Paper
正在生成论文摘要