Block-cyclic stochastic coordinate descent for deep neural networks
Neural Networks(2021)
摘要
We present a stochastic first-order optimization algorithm, named block-cyclic stochastic coordinate descent (BCSC), that adds a cyclic constraint to stochastic block-coordinate descent in the selection of both data and parameters. It uses different subsets of the data to update different subsets of the parameters, thus limiting the detrimental effect of outliers in the training set. Empirical tests in image classification benchmark datasets show that BCSC outperforms state-of-the-art optimization methods in generalization leading to higher accuracy within the same number of update iterations. The improvements are consistent across different architectures and datasets, and can be combined with other training techniques and regularizations.
更多查看译文
关键词
Coordinate descent,Deep neural network,Energy optimization,Stochastic gradient descent
AI 理解论文
溯源树
样例
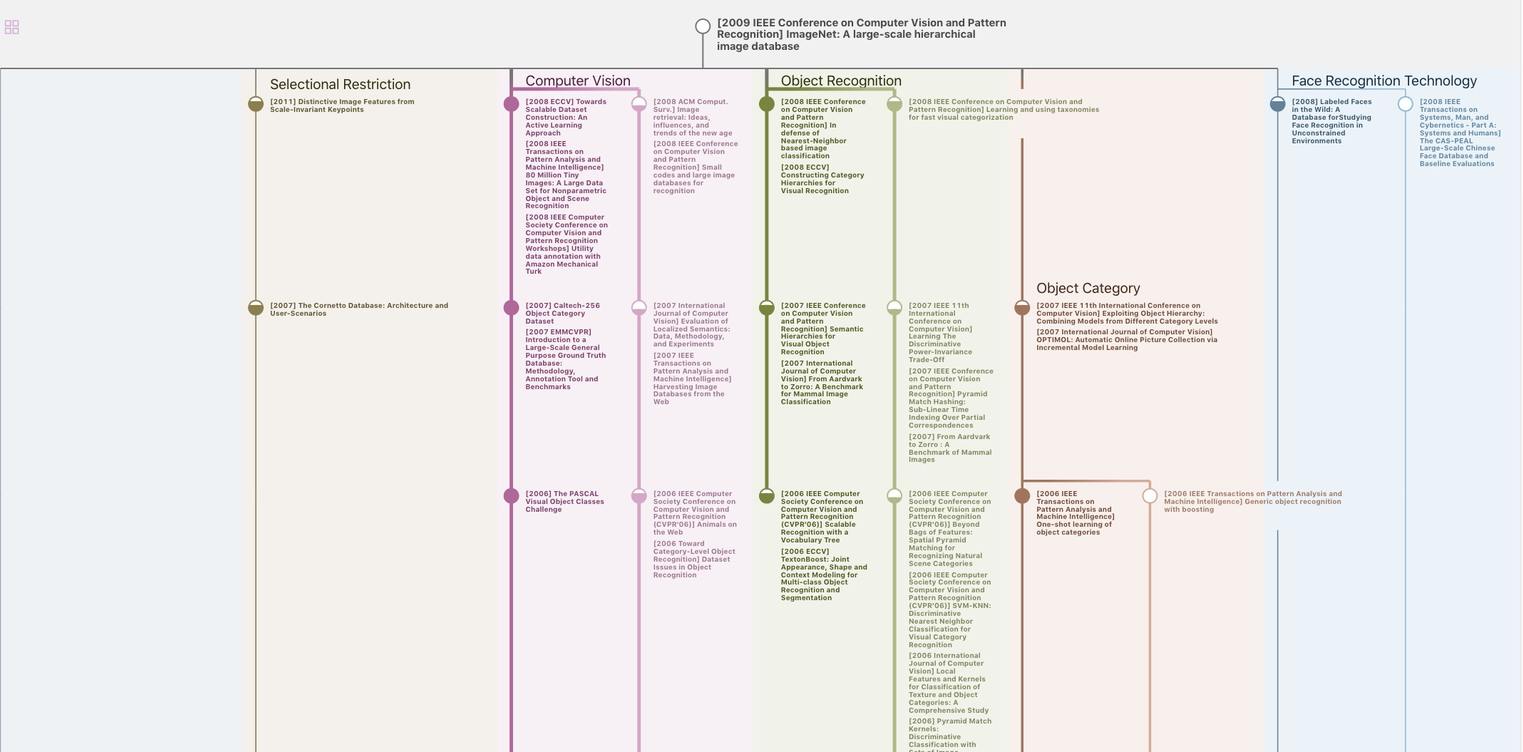
生成溯源树,研究论文发展脉络
Chat Paper
正在生成论文摘要