Literally better: Analyzing and improving the quality of literals.
SEMANTIC WEB(2018)
摘要
Quality is a complicated and multifarious topic in contemporary Linked Data research. The aspect of literal quality in particular has not yet been rigorously studied. Nevertheless, analyzing and improving the quality of literals is important since literals form a substantial (one in seven statements) and crucial part of the Semantic Web. Specifically, literals allow infinite value spaces to be expressed and they provide the linguistic entry point to the LOD Cloud. We present a toolchain that builds on the LOD Laundromat data cleaning and republishing infrastructure and that allows us to analyze the quality of literals on a very large scale, using a collection of quality criteria we specify in a systematic way. We illustrate the viability of our approach by lifting out two particular aspects in which the current LOD Cloud can be immediately improved by automated means: value canonization and language tagging. Since not all quality aspects can be addressed algorithmically, we also give an overview of other problems that can be used to guide future endeavors in tooling, training, and best practice formulation.
更多查看译文
关键词
Data Quality,Data Observatory,Quality Assessment,Quality Improvement,Linked Data
AI 理解论文
溯源树
样例
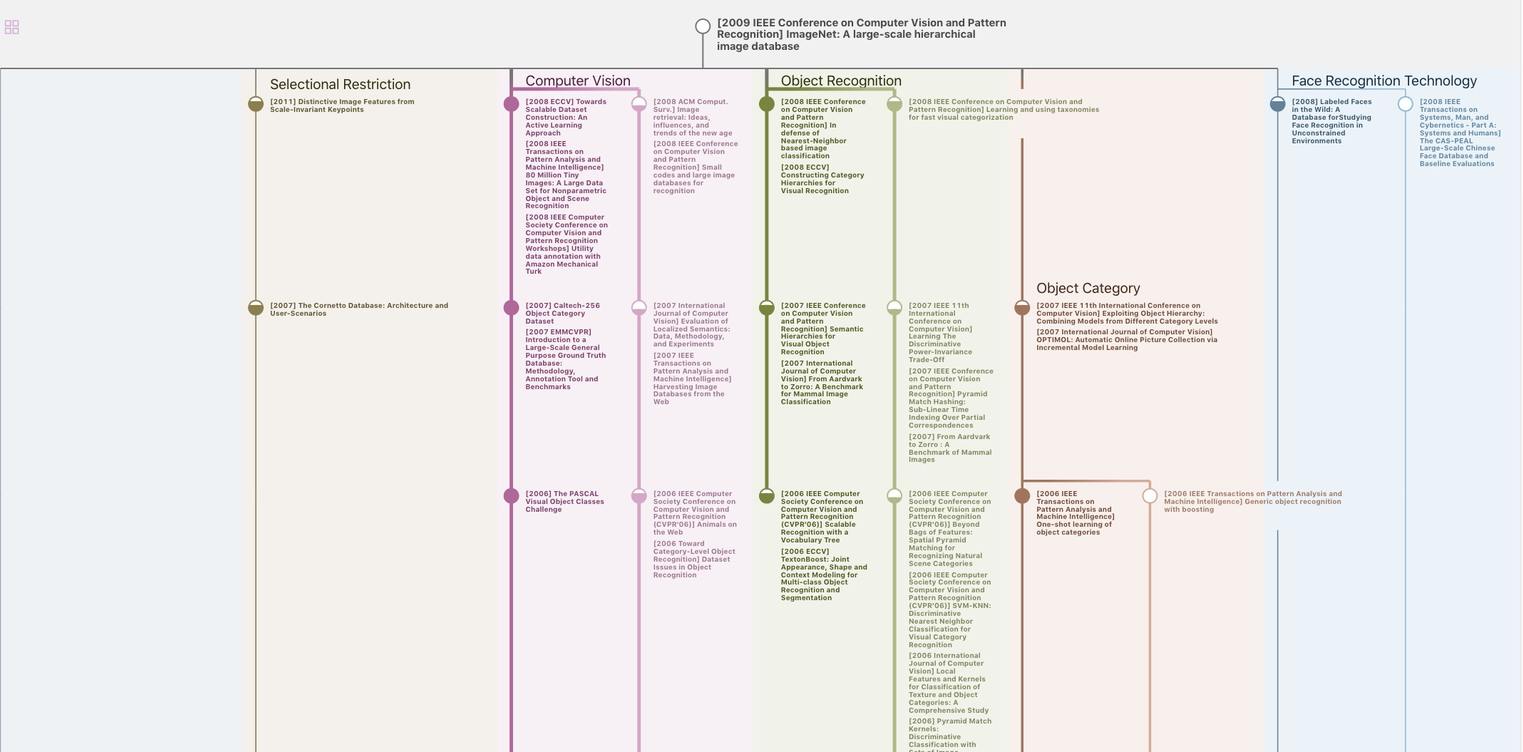
生成溯源树,研究论文发展脉络
Chat Paper
正在生成论文摘要