A clustering algorithm using skewness-based boundary detection.
Neurocomputing(2018)
摘要
Clustering analysis has been applied in all aspects of data mining. Density-based and grid-based clustering algorithms are used to form clusters from the core points or dense grids to extend to the boundary of the clusters. However, deficiencies are still existed. To find out the right boundary and improve the precision of the cluster, this paper has proposed a new clustering algorithm (named C-USB) based on the skew characteristic of the data distribution in the cluster margin region. The boundary degree calculated by skew degree and the local density are used to distinguish whether a data is an internal point or non-internal point. And the connected matrix is constructed by removing the neighbor relationships of non-internal points from the relationships of all points, then the clusters can be formed by searching from the connected matrix towards internal of the clusters. Experimental results on synthetic and real data sets show that the C-USB has higher accuracy than that of similar algorithms.
更多查看译文
关键词
Skewness,Boundary degree,Clustering algorithm,Clustering boundary
AI 理解论文
溯源树
样例
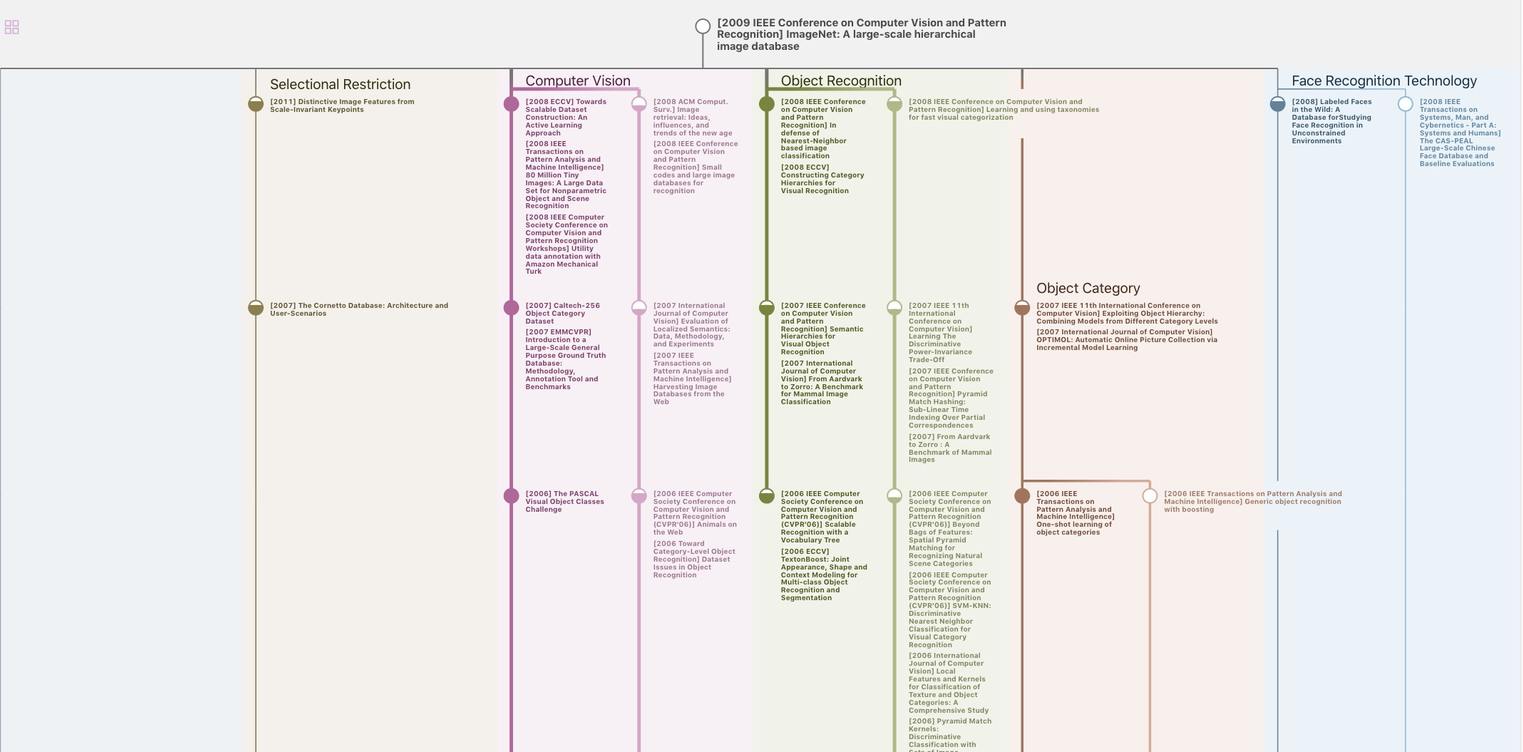
生成溯源树,研究论文发展脉络
Chat Paper
正在生成论文摘要